The Sad Reality of AI Job Market w/ ML Engineer
Summary
TLDRDans ce script de vidéo, un ingénieur en apprentissage automatique partage ses expériences et les prévisions du marché de l'emploi dans l'IA. Il discute des malentendus courants autour de l'IA, la difficulté de l'intégration des modèles ML dans les infrastructures des entreprises et les compétences manquantes dans les formations académiques. Il met en lumière l'importance de l'ingénierie de l'intégration et de la maintenance des modèles, ainsi que l'émergence des compétences en ingénierie de prompt pour les modèles génératifs d'IA.
Takeaways
- 🎓 L'auteur a choisi de suivre une maîtrise pendant l'essor de l'IA, sachant que le domaine était en pleine croissance.
- 📈 Il y a eu une forte demande pour les ingénieurs en apprentissage automatique et la science des données, mais celle-ci a diminué en 2023, suite à une surévaluation de l'IA en 2022.
- 🤖 La communauté a une tendance à surestimer les capacités de l'IA comme étant une solution miracle, ce qui n'est pas le cas.
- 🏢 Les entreprises ont souvent besoin d'ingénieurs qui peuvent non seulement créer des modèles de machine learning, mais aussi les intégrer efficacement dans leur infrastructure existante.
- 💼 Les salaires des ingénieurs en apprentissage automatique sont élevés, et leur productivité doit justifier ces coûts.
- 🔍 L'intégration de l'IA dans les entreprises est un défi majeur, nécessitant des compétences d'ingénierie pour gérer les pipelines de données et l'hébergement des modèles.
- 👨🏫 L'éducation traditionnelle en apprentissage automatique se concentre plus sur la théorie que sur les compétences pratiques nécessaires pour l'industrie.
- 📉 En 2023, les postes de machine learning ont diminué, tandis que ceux liés à l'intelligence artificielle générative et à l'ingénierie de prompts ont augmenté.
- 🛠️ L'ingénierie de prompts est une compétence clé pour tirer le meilleur parti des modèles de l'IA, et elle n'est pas souvent enseignée dans les écoles.
- 🔄 La maintenance des modèles de machine learning est importante, car leur performance peut dériver avec le temps si elle n'est pas surveillée et retraînée.
- 🧐 L'auteur a découvert par lui-même l'importance de l'ingénierie de prompts en utilisant des modèles de l'IA pour résoudre des problèmes techniques complexes.
Q & A
Quelles étaient les attentes initiales de Tanner concernant l'industrie de l'IA lorsqu'il a commencé son master?
-Tanner pensait qu'il terminerait son master au moment parfait, avec une forte demande pour des ingénieurs en apprentissage automatique, ce qui lui permettrait de décrocher un emploi facilement, de gagner beaucoup d'argent et de prendre sa retraite dans dix ans.
Quels sont les malentendus courants sur l'IA que Tanner a rencontrés dans son travail?
-Un malentendu courant est que l'IA est une solution miracle qui peut augmenter rapidement les revenus de l'entreprise. En réalité, il est beaucoup plus difficile de créer une valeur durable avec l'IA qu'on ne le pense.
Pourquoi Tanner pense-t-il que les entreprises ont embauché massivement en 2022?
-Les entreprises ne voulaient pas être laissées pour compte dans la course à l'IA, elles ont donc embauché massivement sans nécessairement savoir comment utiliser ces talents, par crainte que leurs concurrents ne les devancent.
Quelles compétences sont souvent négligées dans les programmes universitaires en IA, selon Tanner?
-Les programmes universitaires se concentrent souvent sur la théorie et les mathématiques avancées nécessaires pour la recherche, mais négligent les compétences pratiques nécessaires pour intégrer les modèles dans les infrastructures complexes des entreprises.
Quel est l'impact de la formation axée sur la théorie par rapport à la formation axée sur la pratique, selon Tanner?
-Une formation axée sur la théorie prépare mieux les étudiants à poursuivre des études académiques ou de recherche, mais ne les prépare pas suffisamment à appliquer leurs compétences de manière pratique dans l'industrie.
Comment Tanner a-t-il découvert l'ingénierie de prompts (prompt engineering)?
-Tanner a découvert l'ingénierie de prompts en essayant de comprendre un bloc de code complexe avec l'aide de chatGPT. Cela a changé sa perception de l'utilité de chatGPT et l'a poussé à apprendre à mieux formuler ses requêtes.
Pourquoi Tanner pense-t-il que l'intégration des modèles d'IA est plus compliquée que leur création?
-L'intégration des modèles d'IA est complexe car elle nécessite de l'ingénierie pour héberger le modèle, gérer les pipelines de données, assurer des prédictions rapides et surveiller les performances du modèle au fil du temps.
Quelles sont les nouvelles tendances en matière d'emplois en IA en 2023, selon Tanner?
-En 2023, les emplois liés à l'IA générative et à l'ingénierie de prompts ont augmenté, tandis que la demande pour les ingénieurs en apprentissage automatique a diminué. Les compétences en chatGPT et l'ingénierie de prompts sont particulièrement recherchées.
Comment Tanner gère-t-il les attentes irréalistes des entreprises concernant l'IA?
-Tanner explique aux entreprises que la création de valeur avec l'IA est plus difficile qu'elles ne le pensent et nécessite des compétences pratiques en ingénierie, pas seulement en modélisation.
Quel conseil Tanner donne-t-il à ceux qui veulent améliorer leurs compétences en ingénierie de prompts?
-Tanner conseille d'expérimenter avec chatGPT pour voir ce qu'il peut faire, d'apprendre des techniques avancées d'ingénierie de prompts et de s'inspirer du travail de prompters expérimentés.
Outlines
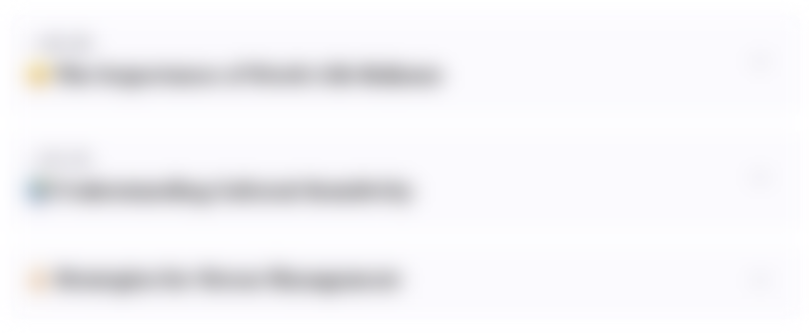
هذا القسم متوفر فقط للمشتركين. يرجى الترقية للوصول إلى هذه الميزة.
قم بالترقية الآنMindmap
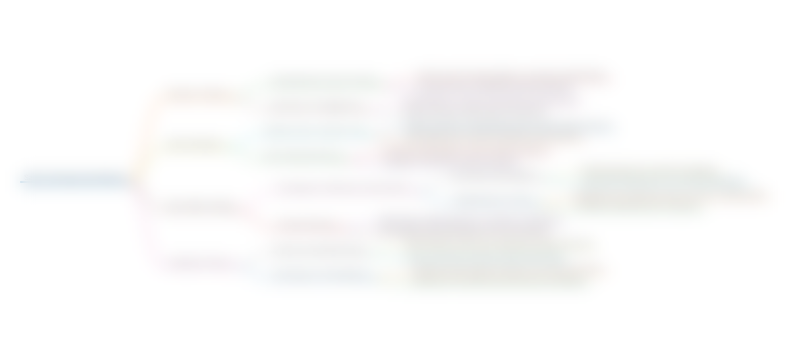
هذا القسم متوفر فقط للمشتركين. يرجى الترقية للوصول إلى هذه الميزة.
قم بالترقية الآنKeywords
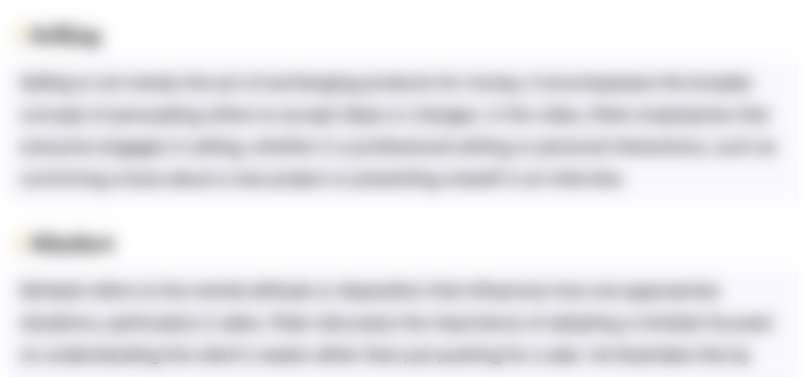
هذا القسم متوفر فقط للمشتركين. يرجى الترقية للوصول إلى هذه الميزة.
قم بالترقية الآنHighlights
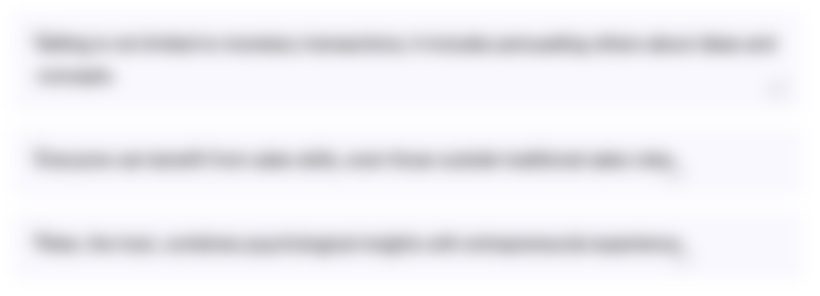
هذا القسم متوفر فقط للمشتركين. يرجى الترقية للوصول إلى هذه الميزة.
قم بالترقية الآنTranscripts
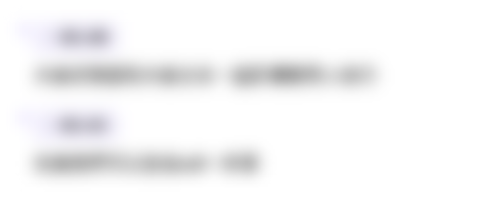
هذا القسم متوفر فقط للمشتركين. يرجى الترقية للوصول إلى هذه الميزة.
قم بالترقية الآنتصفح المزيد من مقاطع الفيديو ذات الصلة

I'm BUYING Now That The Next Phase Of The Bull Market Has Started! Do This Now!

Bitcoin Is About To Get OUT OF CONTROL!
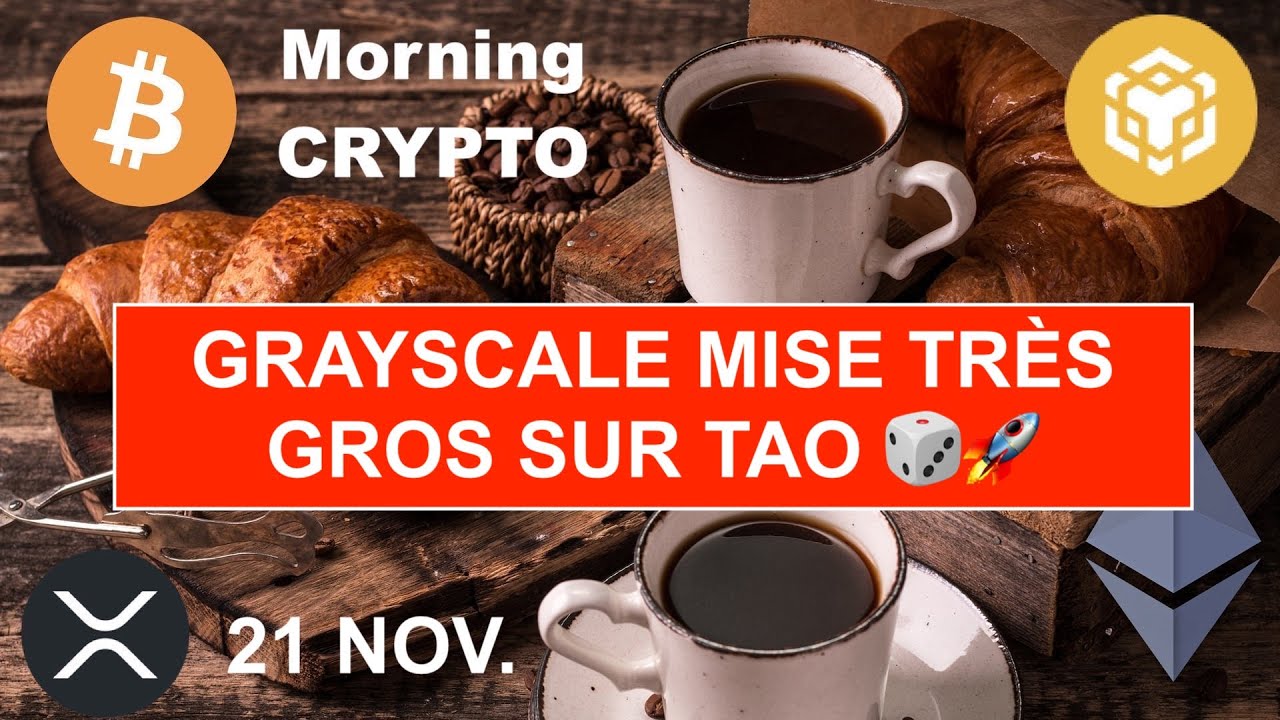
🚨 21 NOV 2024☀️☕️🥐 GRAYSCALE MISE TRÈS GROS SUR TAO BITTENSOR ⚠️ NVIDIA MI FIGUE 🫐 MI RAISIN 🍇
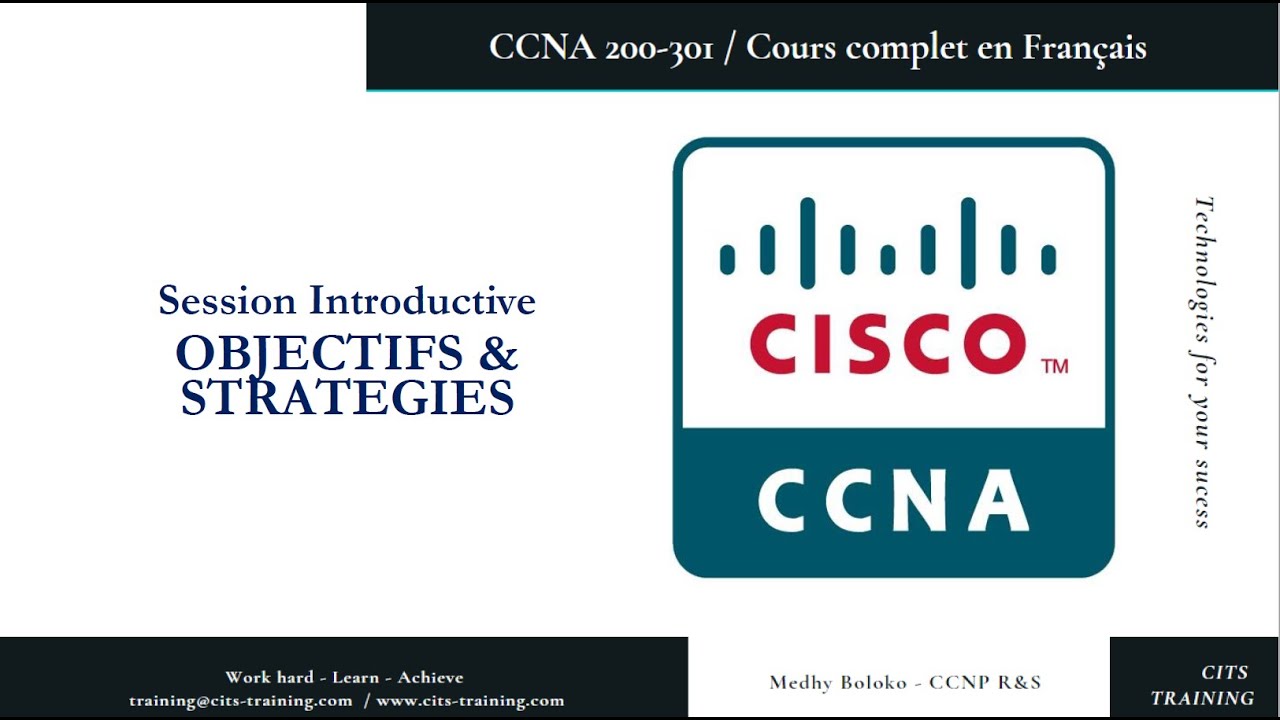
CCNA 200-301 en Français - Session Introductive : Objectifs & Strategies
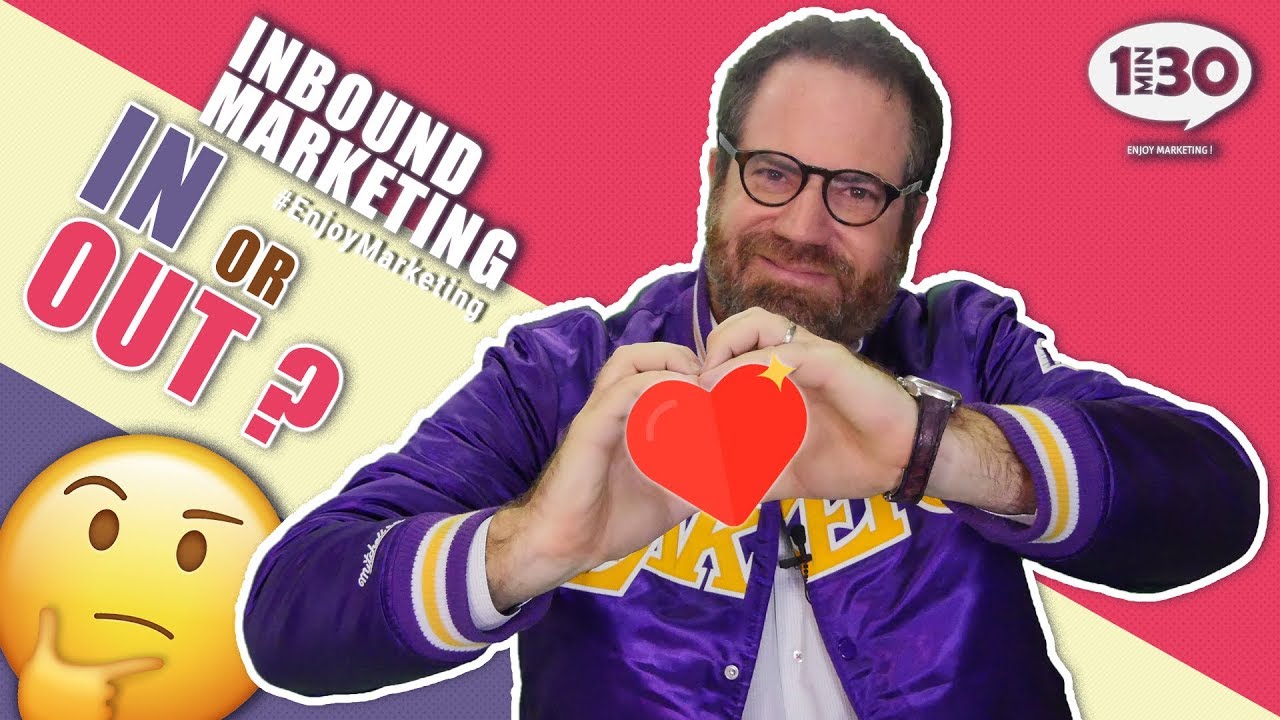
Inbound Marketing : In or Out ? #EnjoyMarketing
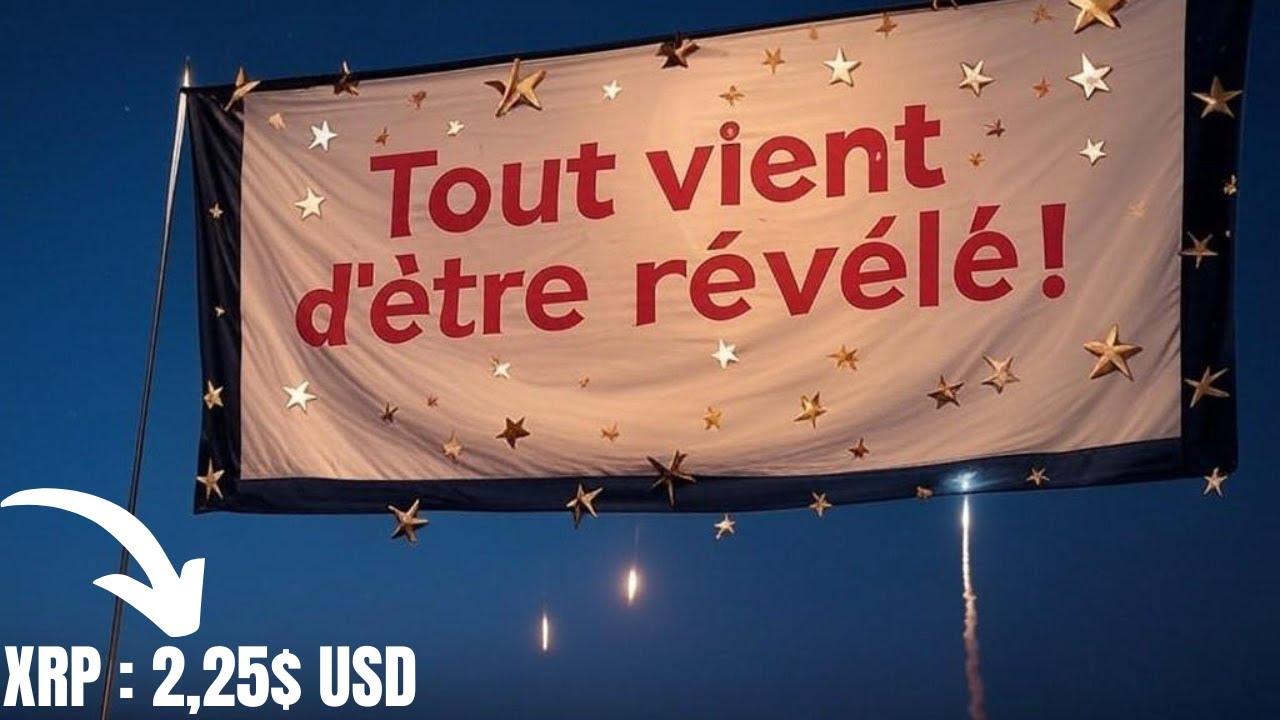
Le mystère est levé : tout vient d’être révélé sur XRP et Ripple ! 🕵️♂️🌍
5.0 / 5 (0 votes)