Spatial Sampling & Interpolation
Summary
TLDRThe video script delves into the intricacies of spatial sampling, a critical process in gathering and analyzing geographical data. It explains the concept of a sample frame and the importance of unbiased sampling where every element has an equal chance of selection. However, due to the complexities of spatial data, biased sampling is sometimes necessary, especially when proximity influences relationships among objects. The script outlines various sampling methods, including random, systematic, stratified, cluster, and transect sampling, each with its advantages and limitations. It also touches on the challenges of implementing random sampling in practice and the trade-offs between representativeness and resource investment. Furthermore, the script introduces spatial interpolation as a technique to estimate values between sampled points, leveraging the spatial autocorrelation found in continuous fields. This method is particularly useful for estimating weather conditions, elevation, and adjusting raster image resolutions. The inverse distance weighting example illustrates how spatial interpolation can be applied effectively.
Takeaways
- 📐 **Spatial Sampling Definition**: Selecting points from within an area, known as the sample frame, to gather data.
- ⚖️ **Bias in Sampling**: A sample is biased if elements have unequal chances of selection, while unbiased sampling gives every element an equal chance.
- 🌐 **Spatial Autocorrelation**: Objects close to each other are more likely to be related, which is important in spatial sampling.
- 🔢 **Random Sampling**: A straightforward method where x and y coordinates are randomly generated within the sample frame.
- 🔄 **Repeated Sampling**: Taking multiple random samples to increase representativeness, but it's more time and resource-intensive.
- 📏 **Systematic Sampling**: Imposing a regular grid on the sample space to ensure evenness, but it may miss features with regular patterns.
- 🤝 **Combined Sampling**: Using a combination of systematic and random sampling to balance structure and randomness.
- 🏪 **Cluster Sampling**: Intensively sampling features within selected clusters, useful for focusing on specific areas.
- 🚶 **Transect Sampling**: Focusing sampling efforts on a specific area of interest, efficient but requires good pre-existing understanding.
- 🌳 **Sample Quantity**: The number of samples needed depends on the spatial homogeneity or heterogeneity of the population.
- 📈 **Spatial Interpolation**: Estimating values between known samples for continuous fields, using patterns observed in the dataset.
- 🔍 **Inverse Distance Weighting**: A spatial interpolation technique that estimates values based on distance from known points, with closer samples weighted more.
Q & A
What is spatial sampling?
-Spatial sampling is the process of selecting points from within an area, known as the sample frame, to gather spatial data for analysis.
Why is it impossible to capture and describe everything about the world at once?
-The world is essentially infinitely complex, making it impossible to capture and describe everything due to the vast amount of information and variables involved.
What are the two main types of sampling that can be applied to spatial data?
-The two main types of sampling are unbiased (where each element has an equal chance of being selected) and biased (where some elements have a greater or lower chance of being selected).
Why might we intentionally design our samples to be biased in spatial sampling?
-In some cases, such as geography, objects that are close to each other are more likely to be related, so a biased sample that focuses on these relationships can be more useful than a completely random one.
What are the advantages of a random sample?
-A random sample is fairly easy to define and implement in theory, providing a good range of possible values in a distribution, and it helps to avoid bias.
What are the potential drawbacks of random sampling?
-Random sampling might result in oversampling of large homogeneous areas or missing smaller elements, leading to an unrepresentative sample of the population.
What is systematic sampling and how does it differ from random sampling?
-Systematic sampling involves imposing a regular grid on the sample space to ensure evenness. It differs from random sampling by introducing a structured bias, which can make the sample more spatially representative but may miss some features with regular patterns.
How can we combine the benefits of systematic and random sampling?
-By defining a regularly spaced grid and then taking random samples within them, we can induce some randomness into the sampling method, which helps to alleviate issues with overlapping periodic features.
What is cluster sampling and when is it beneficial?
-Cluster sampling involves intensive sampling of features in clusters around selected locations. It is beneficial when focusing on certain areas, such as shopping centers for a survey, as it allows for efficient targeting of the desired population.
What is transect sampling and how is it used?
-Transect sampling allows for focusing efforts on a specific area of interest, making it an efficient way to sample a key feature without sampling outside of the focus area. It is commonly used along linear features like roads or rivers.
How does the number of samples required to represent a population relate to the spatial homogeneity of the area?
-The number of samples required is a function of how similar the units of a population are. More homogenous areas require fewer samples, while areas with high spatial heterogeneity need more samples to adequately represent the population.
What is spatial interpolation and why is it used?
-Spatial interpolation is a technique used to estimate values of a continuous field at places where measurements are not available. It is used to fill in gaps between samples, making estimations between weather stations, estimating elevation, and changing the resolution of raster images.
How does inverse distance weighting work in spatial interpolation?
-Inverse distance weighting is a method where values are interpolated based on their distance from known points, with closer samples given more weight than distant ones. The further away an object is, the less weight it has in the interpolation process.
Outlines
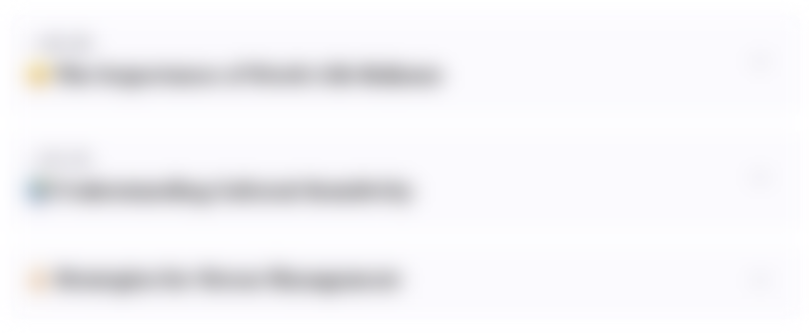
هذا القسم متوفر فقط للمشتركين. يرجى الترقية للوصول إلى هذه الميزة.
قم بالترقية الآنMindmap
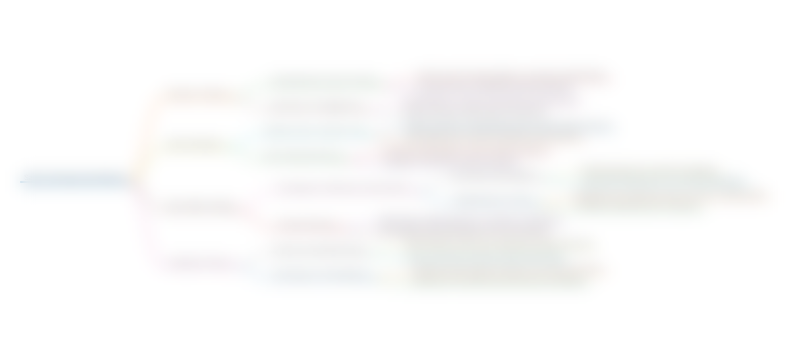
هذا القسم متوفر فقط للمشتركين. يرجى الترقية للوصول إلى هذه الميزة.
قم بالترقية الآنKeywords
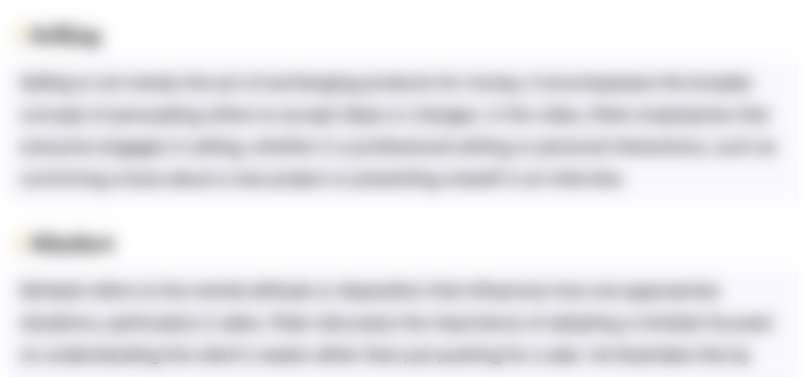
هذا القسم متوفر فقط للمشتركين. يرجى الترقية للوصول إلى هذه الميزة.
قم بالترقية الآنHighlights
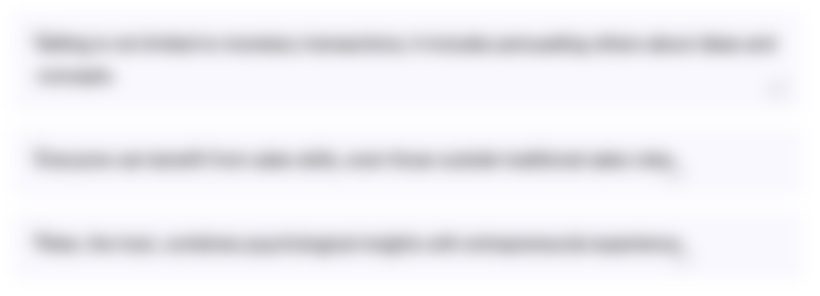
هذا القسم متوفر فقط للمشتركين. يرجى الترقية للوصول إلى هذه الميزة.
قم بالترقية الآنTranscripts
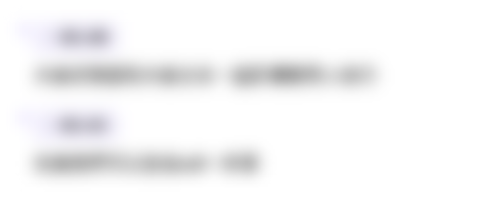
هذا القسم متوفر فقط للمشتركين. يرجى الترقية للوصول إلى هذه الميزة.
قم بالترقية الآنتصفح المزيد من مقاطع الفيديو ذات الصلة
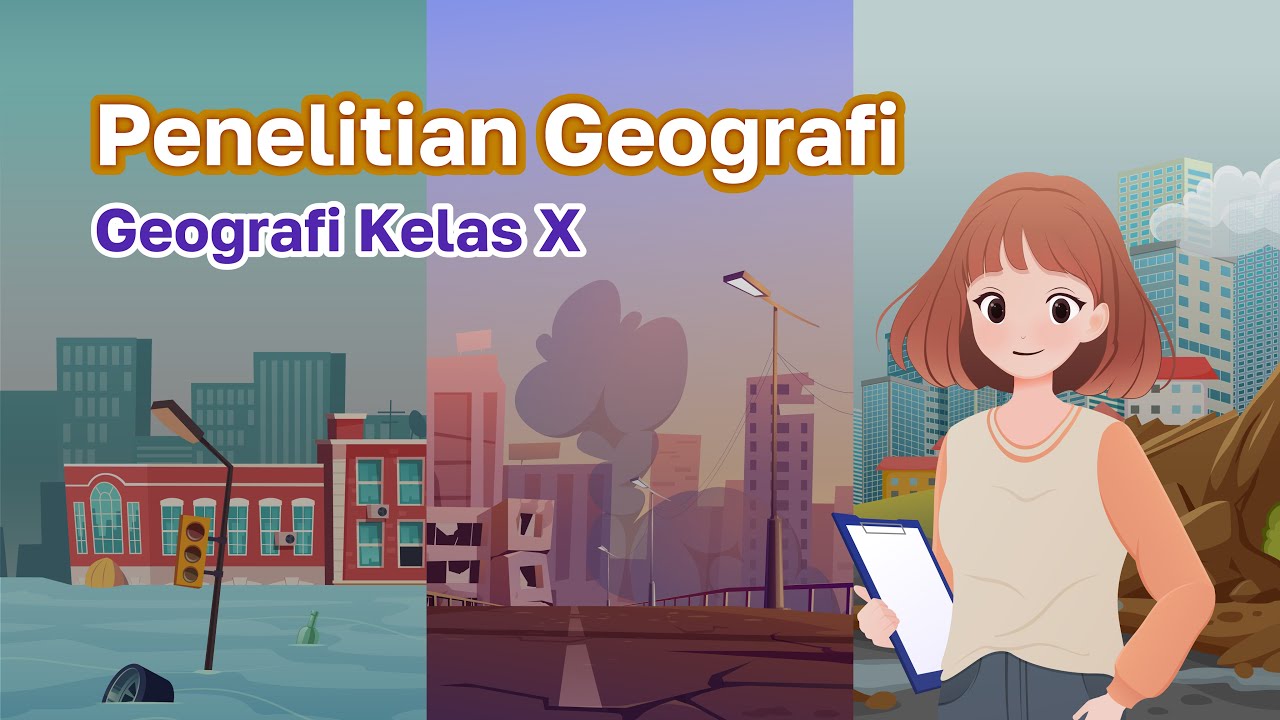
Penelitian Geografi | Geografi Kelas 10 - KHATULISTIWA MENGAJAR

Penelitian Geografi - Materi Geografi kelas 10 - Penjelasan Lengkap dan Mendalam - Jago UTBK
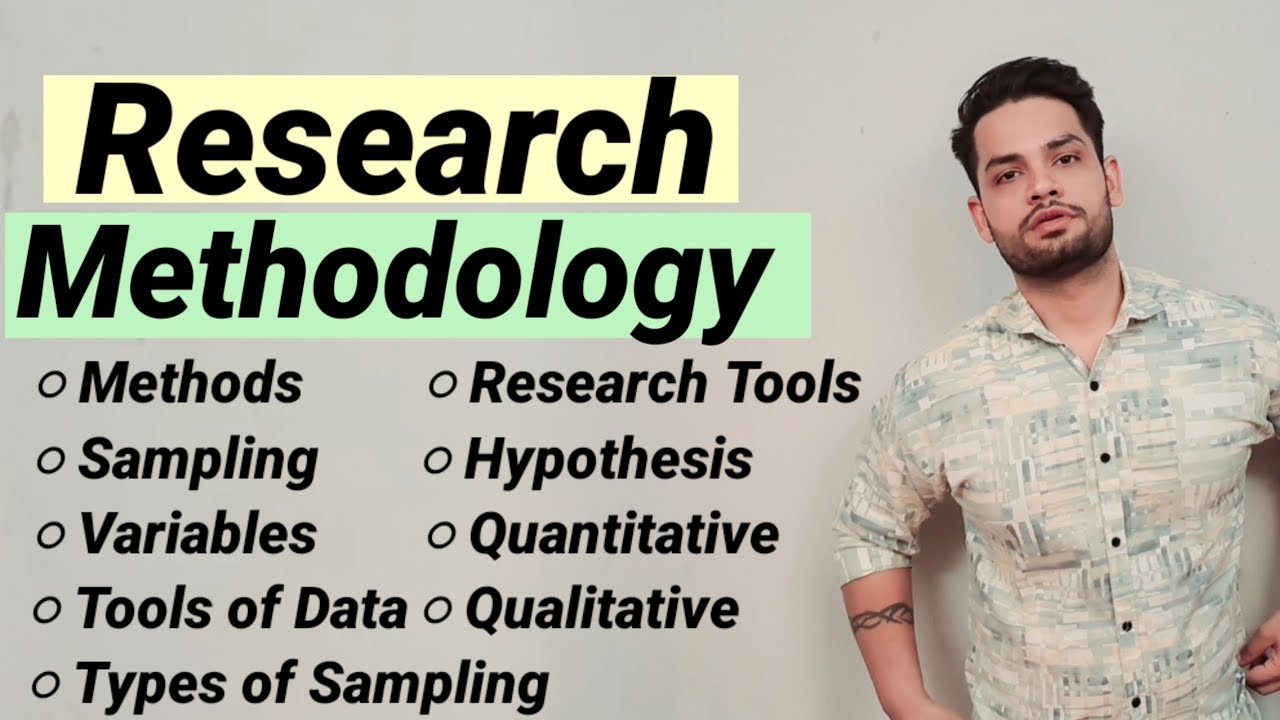
Research Methodology | Sampling | variables complete video

PRINSIP GEOGRAFI #geography #education #kurikulummerdeka
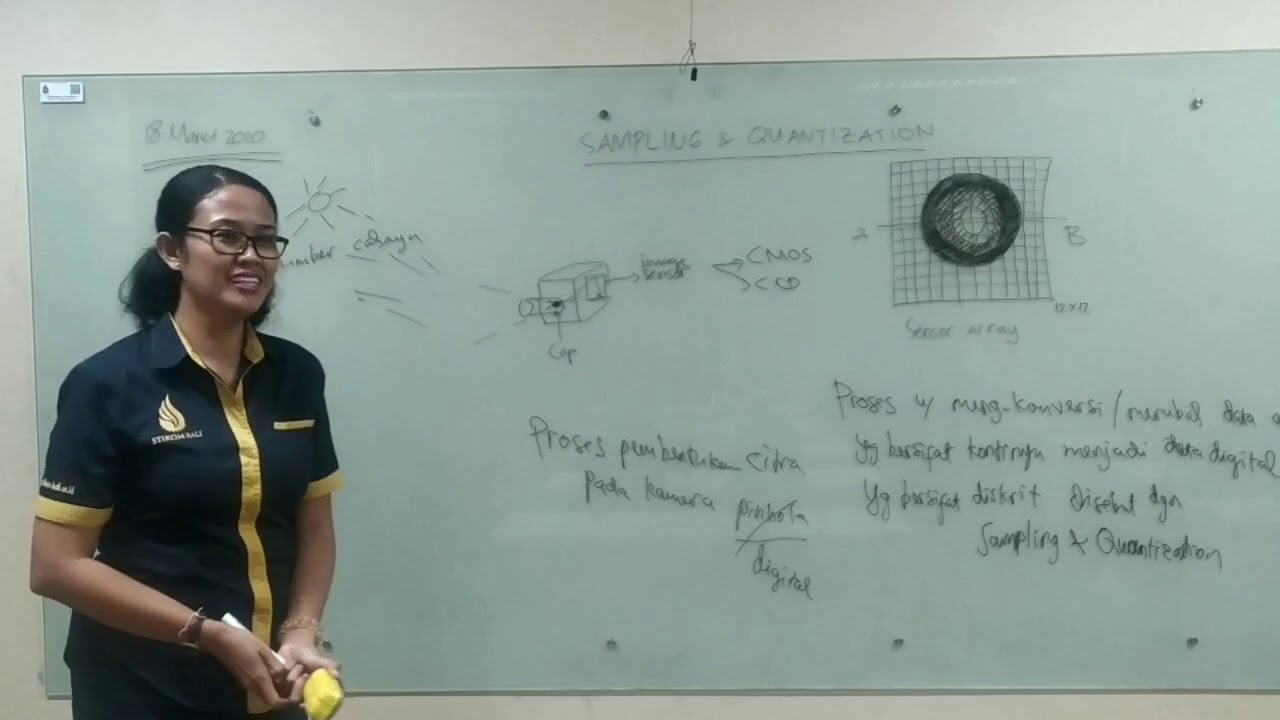
Pengolahan Citra Digital: Sampling dan Kuantisasi

Territorialização na Atenção Básica
5.0 / 5 (0 votes)