Q-Star 2.0 - AI Breakthrough Unlocks New Scaling Law (New Strawberry)
Summary
TLDRA breakthrough in AI development, test time training, is pushing models towards artificial general intelligence (AGI). By dynamically adjusting model parameters during inference, this method improves problem-solving capabilities. Applied to the Arc Prize challenge, it led to a score of 61.9%, surpassing the average human score. The technique, leveraging efficient fine-tuning and augmented data, showcases how AI can adapt to novel tasks with minimal data. This development suggests that improving AI's adaptability, rather than expanding training data, could be key to achieving AGI, even with smaller models.
Takeaways
- 😀 The Ark Prize is a key benchmark for AGI, testing models' ability to generalize to new tasks, much like human reasoning.
- 😀 The 01 family of models introduced the Chain of Thought technique, enabling models to think through problems rather than provide instant answers.
- 😀 Test Time Training (TTT) is a new method that fine-tunes models during inference, improving their performance on unseen tasks.
- 😀 The new Test Time Training method achieved a score of 61.9% on the Ark Prize, significantly surpassing the previous record of 42%.
- 😀 The average human score on the Ark Prize tasks is 60%, and the best human score is nearly 98%. Models still have a long way to go to match human performance.
- 😀 Test Time Training allows models to adapt dynamically during inference, fine-tuning themselves with new data generated from the problem at hand.
- 😀 Previous AI models struggled with novel reasoning tasks, but Test Time Training allows them to solve such problems by creating and using variations of the input data.
- 😀 Techniques like Chain of Thought, augmented inference, and ensembling predictions have been key in improving model performance.
- 😀 The core insight of the research is that computational resources during test time (inference) are critical to improving model performance, even without symbolic reasoning.
- 😀 The paper demonstrates that smaller models, when augmented with these techniques, can perform significantly better on complex reasoning tasks.
- 😀 Test Time Training challenges previous assumptions about symbolic components and suggests that adapting model parameters dynamically during inference is a key to solving novel tasks.
Q & A
What is the new language model technique mentioned in the script?
-The new language model technique discussed is called test time training, which allows a model to update its parameters during inference, enabling it to adapt dynamically to new tasks in real-time.
How does test time training differ from traditional fine-tuning?
-Test time training updates model parameters temporarily during inference, using a small amount of additional data to fine-tune the model for specific problems, while traditional fine-tuning involves updating the model based on a large dataset during the training phase.
What major achievement did the test time training method accomplish in the context of the Ark Prize?
-Test time training helped achieve a significant improvement in the Ark Prize, reaching a score of 61.9%, surpassing the previous top score of 42%. This was a notable advancement in AI's ability to generalize to novel tasks.
What is the Ark Prize, and how does it relate to AGI benchmarks?
-The Ark Prize is a competition aimed at creating a solution for the Ark AGI Benchmark, which tests generalization capabilities by challenging models to solve novel problems with minimal guidance. The prize aims to evaluate advancements in Artificial General Intelligence (AGI).
How does the Ark Prize evaluate models and their generalization abilities?
-The Ark Prize evaluates models based on their ability to generalize from a few examples and apply learned knowledge to solve new problems, such as transforming a 7x7 grid based on a small number of provided examples.
What does the success of the new test time training method suggest about model capabilities?
-The success of test time training suggests that models can perform complex reasoning tasks by dynamically adjusting their parameters during inference, challenging the assumption that symbolic components are necessary for solving novel problems.
What is the importance of Chain of Thought and how does it relate to test time training?
-Chain of Thought is a method where the model takes time to reason through problems, and when combined with test time training, it helps the model better handle complex problems by updating its parameters during inference, thus improving its overall performance.
What is the concept of Laura (Low-Rank Adaptation) mentioned in the script?
-Laura is a technique for efficiently fine-tuning models during inference by updating only a small number of parameters, while keeping the majority of the model weights frozen. It allows for lightweight customization of models and was used in conjunction with test time training.
What are the three key components of test time training outlined in the research paper?
-The three key components are: 1) Initial fine-tuning on similar tasks, 2) Auxiliary task formats and augmentations to generate training data, and 3) Per-instance training, which involves dynamically adapting the model for each test input.
How did the use of augmented inference and ensembling improve the performance of the model?
-Augmented inference involves generating multiple prediction candidates through geometric transformations, while ensembling uses a voting strategy to select the best candidates. This process helps to refine the model's predictions, resulting in improved performance on complex tasks.
Outlines
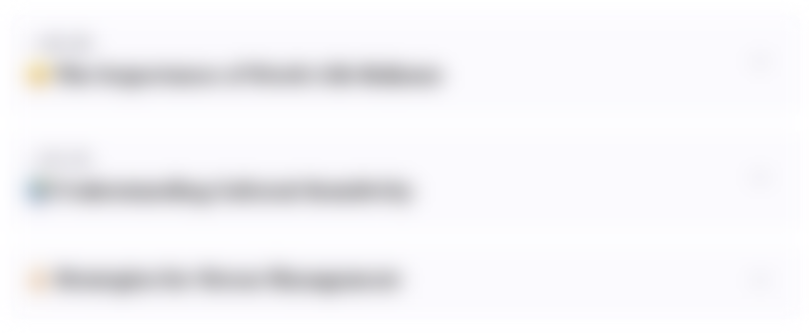
هذا القسم متوفر فقط للمشتركين. يرجى الترقية للوصول إلى هذه الميزة.
قم بالترقية الآنMindmap
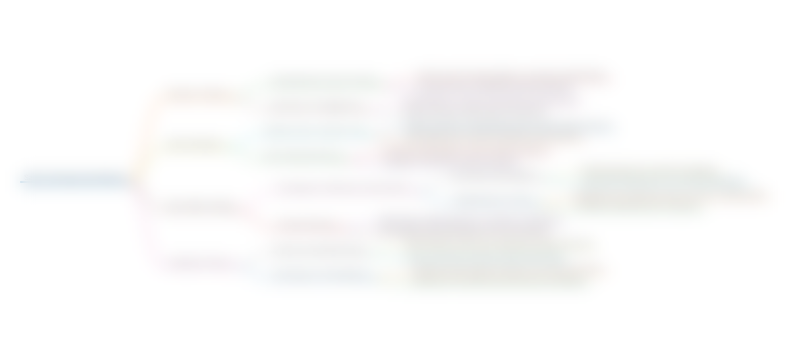
هذا القسم متوفر فقط للمشتركين. يرجى الترقية للوصول إلى هذه الميزة.
قم بالترقية الآنKeywords
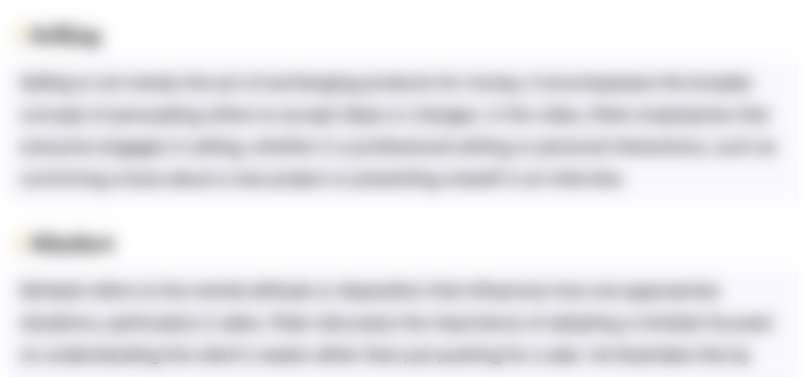
هذا القسم متوفر فقط للمشتركين. يرجى الترقية للوصول إلى هذه الميزة.
قم بالترقية الآنHighlights
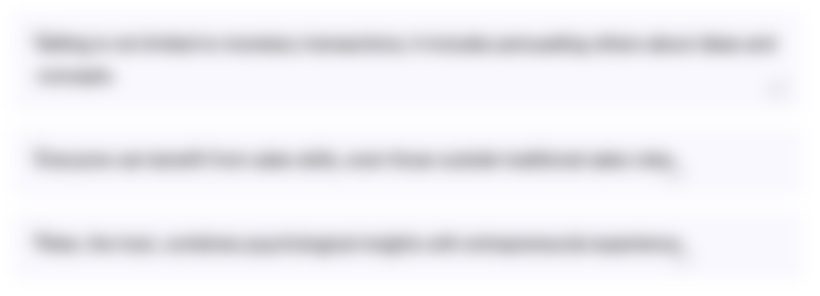
هذا القسم متوفر فقط للمشتركين. يرجى الترقية للوصول إلى هذه الميزة.
قم بالترقية الآنTranscripts
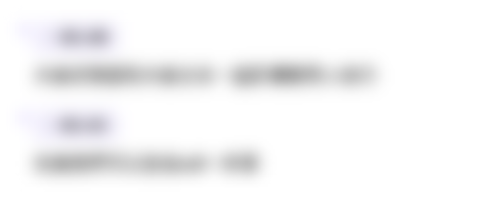
هذا القسم متوفر فقط للمشتركين. يرجى الترقية للوصول إلى هذه الميزة.
قم بالترقية الآنتصفح المزيد من مقاطع الفيديو ذات الصلة
5.0 / 5 (0 votes)