Prompt Engineering = BS? (Must Watch)
Summary
TLDRThis video delves into a groundbreaking research study on prompt engineering techniques for AI in software engineering tasks, focusing on code generation, translation, and summarization. It reveals surprising findings, such as the effectiveness of zero-shot prompting for advanced models like GPT-4, and challenges the reliance on detailed custom instructions. The study emphasizes the importance of simple prompts and iterative feedback for improving results, rather than complex techniques like Chain-of-Thought or agent-based approaches. Key recommendations include using reasoning models for multi-step tasks and opting for cost-effective, straightforward prompting strategies.
Takeaways
- 😀 Zero-shot prompting often outperforms other techniques like Chain of Thought for reasoning models like O1 Mini.
- 😀 Advanced LLMs (e.g., GPT-4) require simpler, less detailed prompts compared to older models, reducing the need for complex prompt engineering.
- 😀 Chain of Thought is valuable for complex tasks with multiple steps but can increase token costs and slow down performance for simple tasks.
- 😀 Iterative feedback (e.g., error correction and runtime testing) is crucial for improving code generation, often more impactful than fine-tuning prompts.
- 😀 Reasoning models like O1 Mini are more expensive and slower, making them less ideal for simple tasks but effective for complex ones with deep reasoning.
- 😀 For cost-sensitive applications, using non-reasoning models like GPT-4 for simpler tasks can be more efficient than reasoning models.
- 😀 Research found that detailed custom instructions and role-playing prompts can hinder performance in certain scenarios, especially for advanced models.
- 😀 Using separate models for architecture and code execution (e.g., splitting tasks between an architect and an executioner) improves code generation results.
- 😀 Prompt engineering benefits are reduced for advanced models compared to earlier models like GPT-3.5 and GPT-4, especially when reasoning is involved.
- 😀 Simple prompt structures, with context provided dynamically and in real-time, can yield better results than rigid, lengthy prompts.
- 😀 There is a significant tradeoff between the cost of using reasoning models and their performance, with excessive reasoning leading to diminished returns.
Q & A
What was the main focus of the research discussed in the video?
-The research focused on evaluating the effectiveness of different prompt engineering techniques in software engineering tasks, specifically for code generation, translation, and summarization, using advanced AI models like GPT-4 and O1 Mini.
What are the primary prompt engineering techniques mentioned in the video?
-The primary techniques discussed include zero-shot prompting, few-shot prompting, chain of thought, expert prompting, agent-based approaches, and iterative refinement.
How did non-reasoning models like GPT-4 perform with prompt engineering techniques?
-For non-reasoning models like GPT-4, prompt engineering still helped, but the benefits were reduced. Execution feedback became more important than the prompt structure itself.
What did the research reveal about the performance of reasoning models like O1 Mini?
-For reasoning models like O1 Mini, zero-shot prompting often performed best, and more complex prompt engineering techniques could actually hinder performance. Built-in reasoning capabilities reduced the need for elaborate prompts.
What tasks are reasoning models like O1 Mini particularly suited for?
-Reasoning models excel at complex tasks requiring multi-step reasoning and problems with chain of thought lengths greater than five steps.
What are the downsides of using reasoning models like O1 Mini?
-Reasoning models are more expensive, take longer to process, and can underperform for simpler tasks. They also struggle with less structured output formats.
When is it more efficient to use non-reasoning models like GPT-4?
-Non-reasoning models are more efficient for simple tasks, tasks requiring concise output, and cost-sensitive applications, where less complex prompts suffice.
What is the main recommendation for using reasoning models effectively?
-When using reasoning models, the research suggests keeping prompts simple, focusing on tasks with complex reasoning requirements, and avoiding complex prompt structures like role-playing or few-shot examples.
Why is iterative refinement an important aspect of prompt engineering?
-Iterative refinement allows for continuous improvement of the output, using feedback from execution or errors, which helps generate higher quality results, especially for complex tasks.
How does the research suggest handling cost and time when using advanced models?
-The research highlights that reasoning models are more costly and time-consuming, so they should be used selectively for tasks requiring deep reasoning. Non-reasoning models should be used for simpler tasks to optimize cost and efficiency.
Outlines
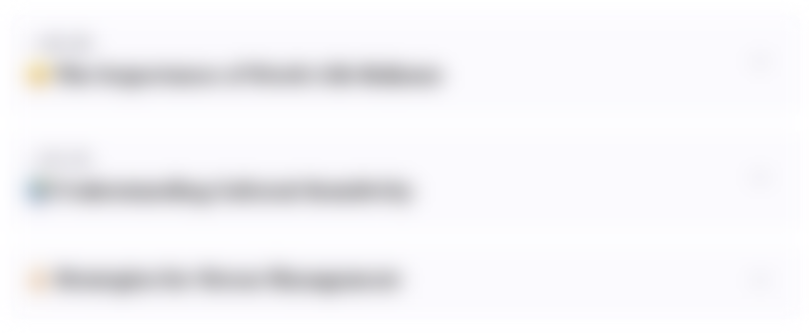
هذا القسم متوفر فقط للمشتركين. يرجى الترقية للوصول إلى هذه الميزة.
قم بالترقية الآنMindmap
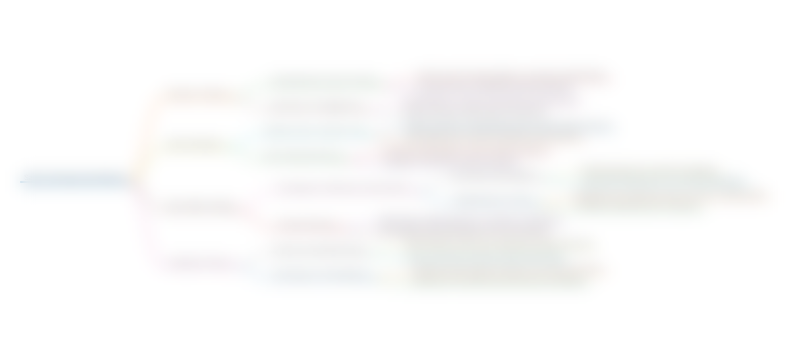
هذا القسم متوفر فقط للمشتركين. يرجى الترقية للوصول إلى هذه الميزة.
قم بالترقية الآنKeywords
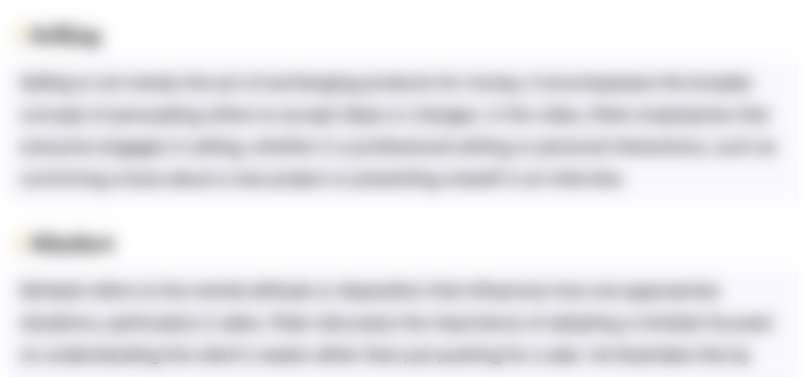
هذا القسم متوفر فقط للمشتركين. يرجى الترقية للوصول إلى هذه الميزة.
قم بالترقية الآنHighlights
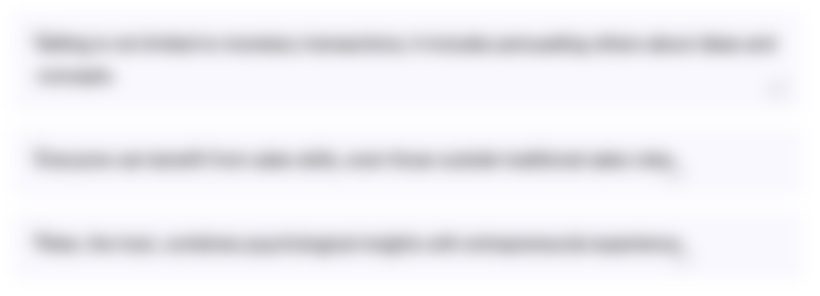
هذا القسم متوفر فقط للمشتركين. يرجى الترقية للوصول إلى هذه الميزة.
قم بالترقية الآنTranscripts
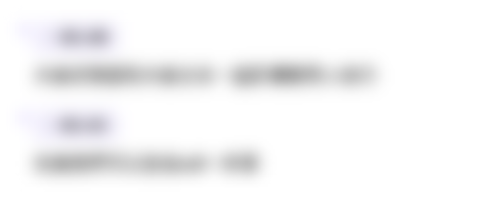
هذا القسم متوفر فقط للمشتركين. يرجى الترقية للوصول إلى هذه الميزة.
قم بالترقية الآنتصفح المزيد من مقاطع الفيديو ذات الصلة
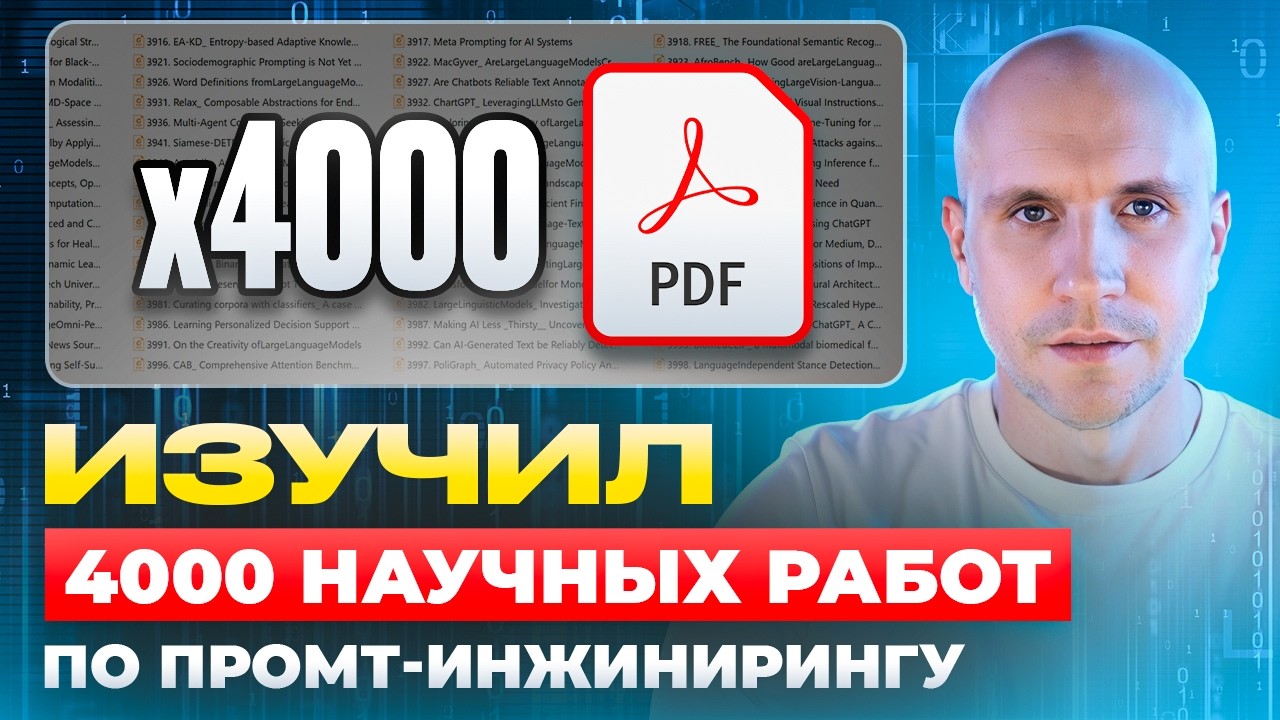
Мои ОТКРЫТИЯ! Потратил $600 на 4000 НОВЕЙШИХ исследований GPT по промпт-инжинирингу
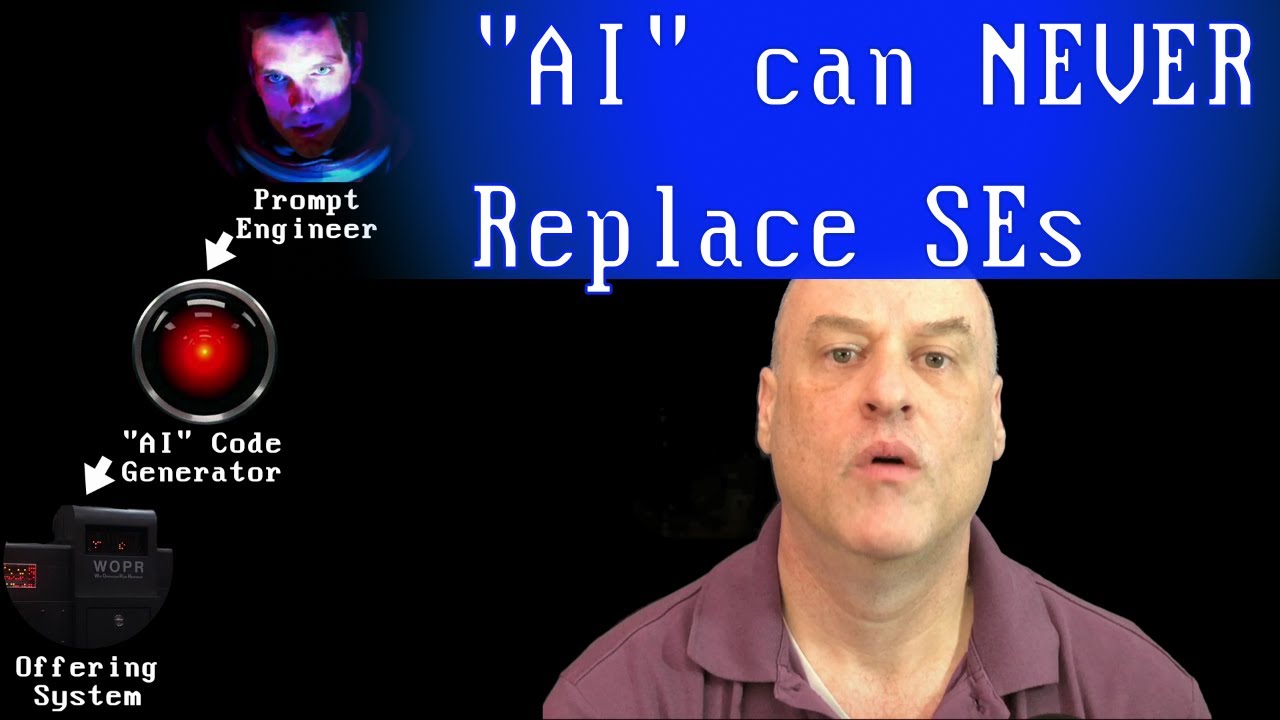
Software Engineer jobs (Mid-Senior) can NEVER be replaced by a Coding "AI" like Devin
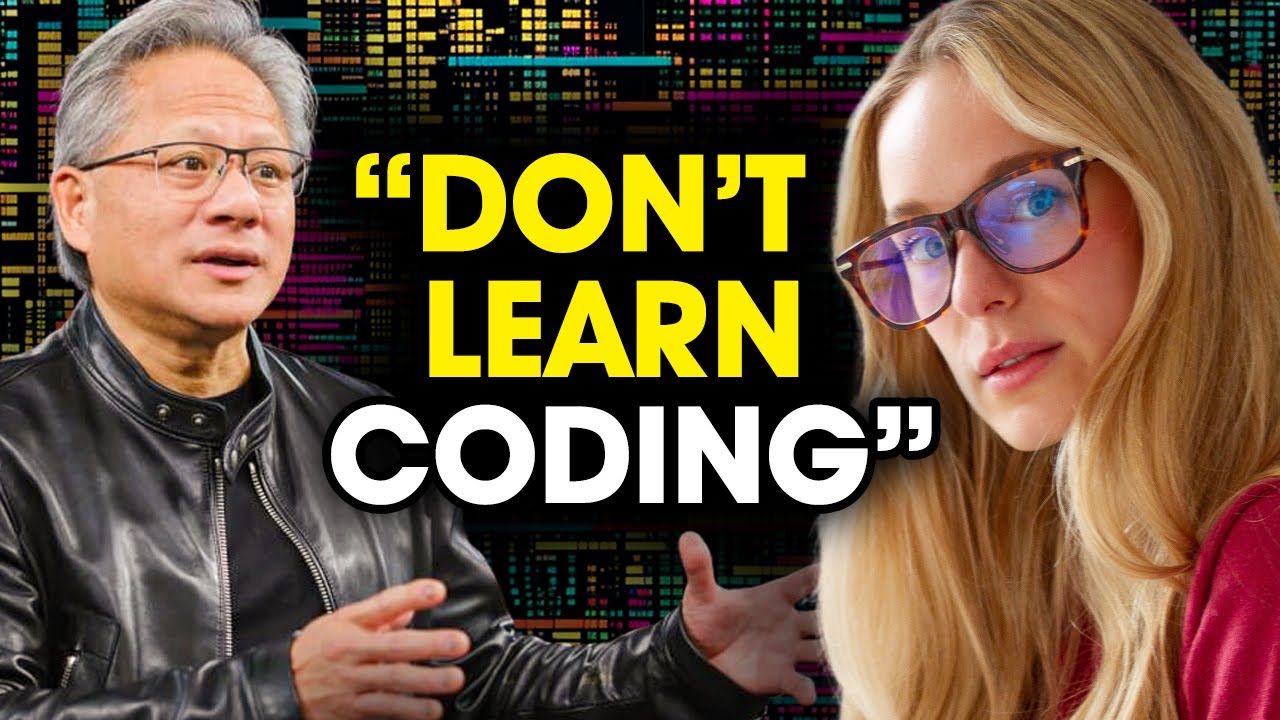
Is Prompt Engineering the NEW Software Engineering?
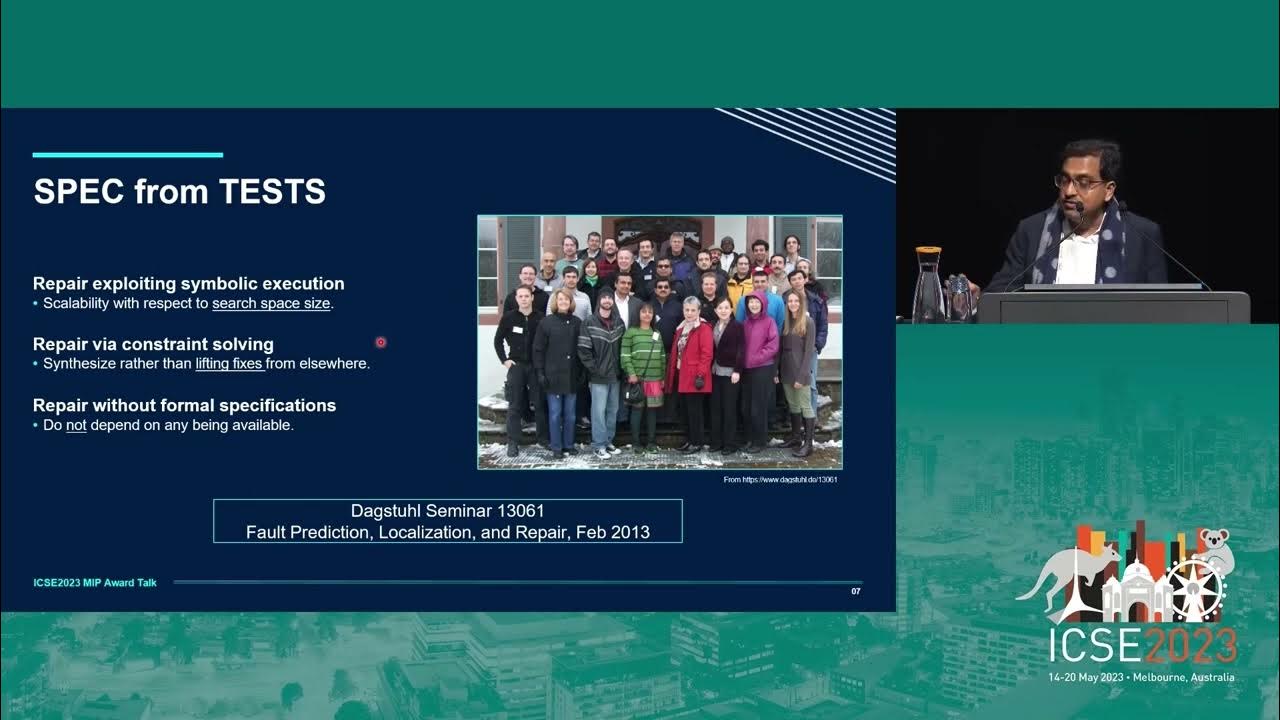
ICSE 2023 - MIP Award talk by Abhik Roychoudhury
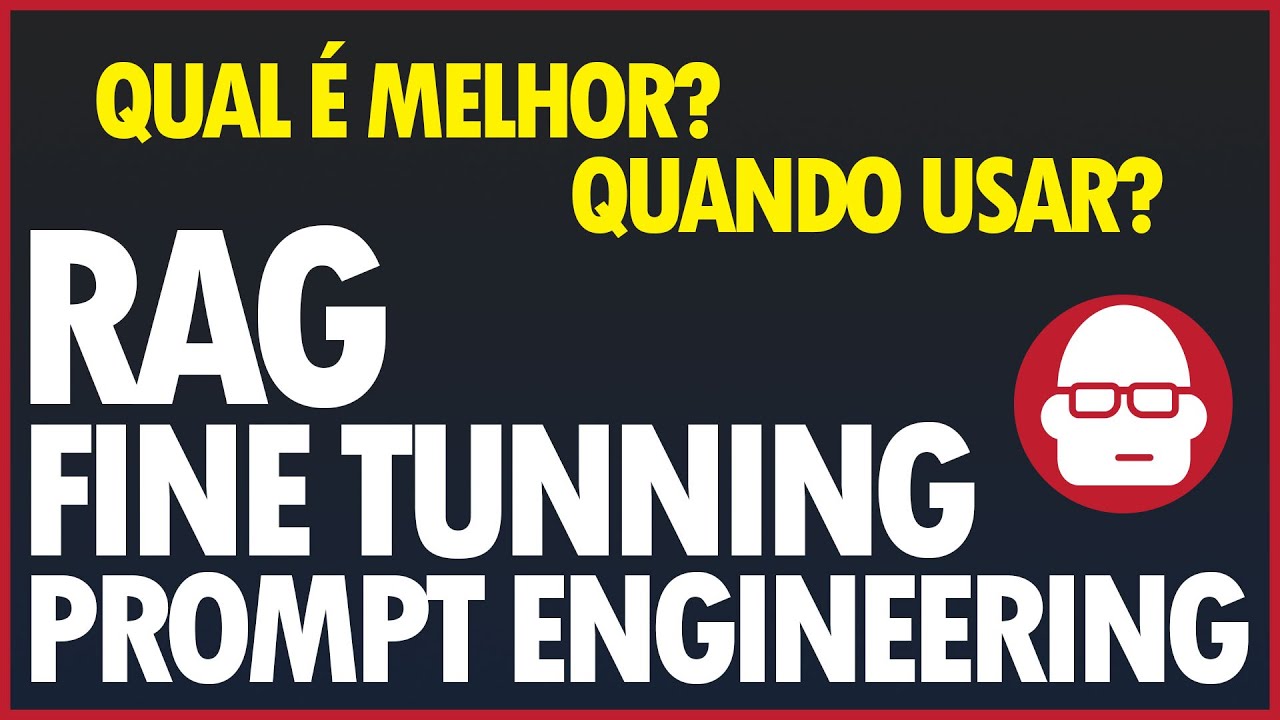
Fine Tuning, RAG e Prompt Engineering: Qual é melhor? e Quando Usar?
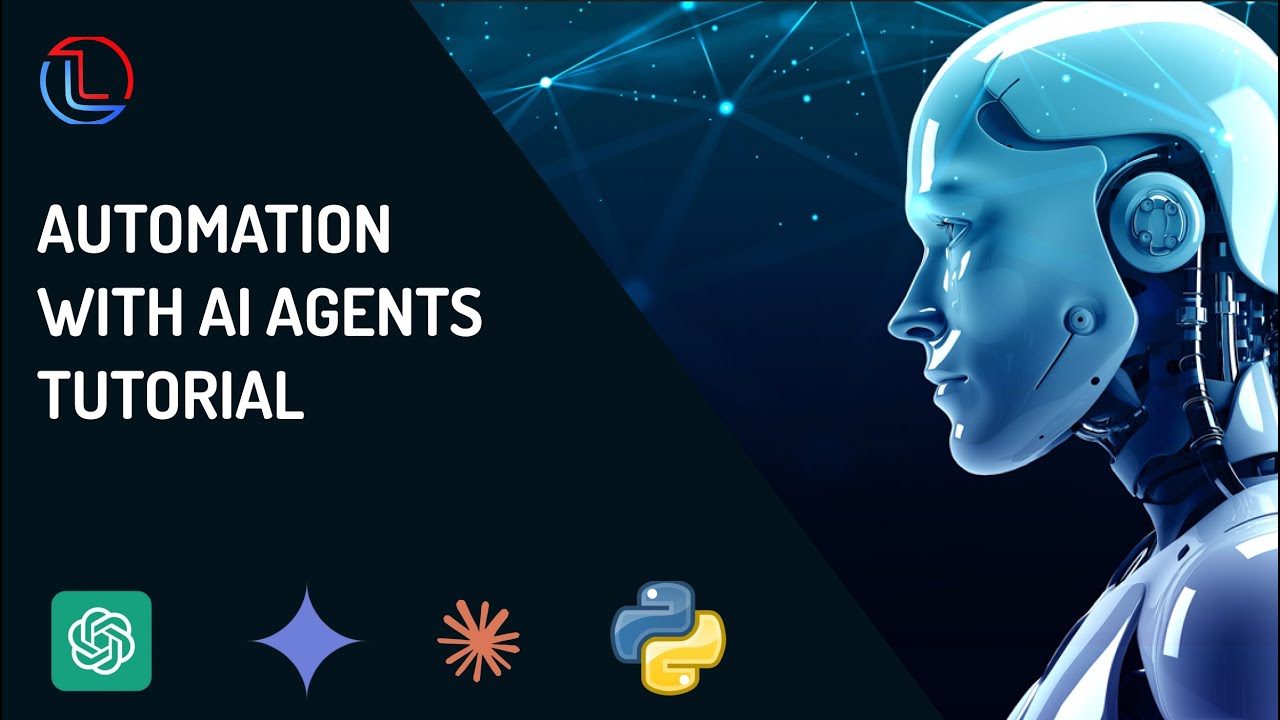
AI Agents Explained: Guide for beginners - Tutorial
5.0 / 5 (0 votes)