W05 Clip 4
Summary
TLDRThis video explains the architecture of GPT models, focusing on their use of a decoder-only setup, which contrasts with traditional Transformer models that use both an encoder and a decoder. It delves into how GPT models achieve tasks like translation, summarization, and question answering without an encoder, thanks to masked self-attention and advanced training methods. The architecture simplifies and enhances efficiency, especially in tasks such as language modeling, while allowing for large-scale training on unlabeled data. The video highlights how this approach makes GPT models powerful despite their streamlined design.
Takeaways
- 🤖 Transformer-based models have revolutionized how machines understand and generate human language.
- 🔄 Traditional Transformer models feature both an encoder and a decoder for language processing tasks.
- ✂️ OpenAI's GPT models diverge by using a decoder-only architecture, omitting the encoder.
- ⚙️ In traditional Transformers, the encoder processes input data into an abstract representation, while the decoder generates the output.
- 🧠 GPT models input data directly into the decoder without prior encoding, utilizing masked self-attention.
- ⏭️ The GPT decoder is restricted from accessing future input segments during output generation, preserving the auto-regressive nature of the model.
- ⚡ The decoder-only architecture simplifies the model, enhancing efficiency, particularly for tasks like language modeling.
- 📊 GPT models can still perform tasks like translation and summarization without an encoder, thanks to self-attention and advanced training techniques.
- 📚 GPT models use self-supervised learning to predict the next word in a sequence based on previous words, learning linguistic patterns.
- 🏆 GPT models excel in tasks typically handled by encoder-decoder models due to their robust decoder and training methodologies.
Q & A
What is the main difference between traditional Transformer models and GPT models?
-Traditional Transformer models use both an encoder and a decoder, while GPT models use a decoder-only architecture.
What role does the encoder play in traditional Transformer models?
-In traditional Transformer models, the encoder ingests input data and converts it into an abstract representation, capturing the semantic and syntactic nuances of the input.
How does the decoder function in a traditional Transformer model?
-The decoder in traditional Transformer models takes the encoded representation from the encoder and generates the output, such as translating a sentence, by utilizing an attention mechanism.
How does the GPT model handle input differently from a traditional Transformer?
-In GPT models, input data is fed directly into the decoder without prior transformation into a higher-level abstract representation by an encoder.
What is the role of masked self-attention in GPT models?
-Masked self-attention in GPT models ensures that the model does not access future segments of the input sequence when generating each output segment, aligning with the auto-regressive nature of GPT training.
Why is the decoder-only architecture considered more efficient in GPT models?
-The decoder-only architecture simplifies the model by omitting the encoder, which makes the model more efficient, especially in tasks like language modeling, and allows for faster output generation.
How do GPT models perform tasks such as translation and summarization without an encoder?
-GPT models leverage self-attention in the decoder and their training methodology to effectively understand input data, allowing them to perform tasks like translation and summarization without an encoder.
What training technique is used in GPT models to enable them to excel in various tasks?
-GPT models are trained using self-supervised learning techniques, where the model predicts the next word in a sentence based on the preceding words.
What advantage does GPT’s decoder-only architecture provide when dealing with large datasets?
-GPT’s decoder-only architecture facilitates training on large volumes of unlabeled data, which is particularly useful in NLP tasks where annotated data is often limited.
In what types of tasks do GPT models excel despite being decoder-only?
-GPT models excel in diverse tasks such as question answering, translation, and summarization, traditionally associated with encoder-decoder models, due to their robust self-attention mechanisms and training techniques.
Outlines
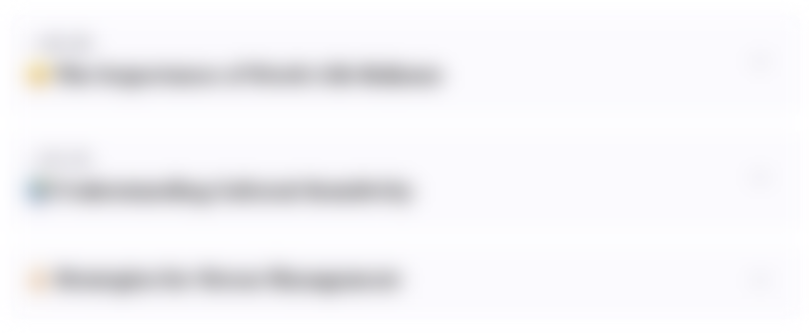
هذا القسم متوفر فقط للمشتركين. يرجى الترقية للوصول إلى هذه الميزة.
قم بالترقية الآنMindmap
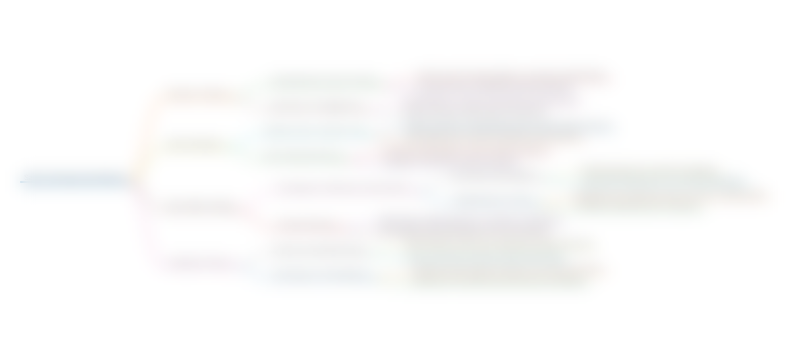
هذا القسم متوفر فقط للمشتركين. يرجى الترقية للوصول إلى هذه الميزة.
قم بالترقية الآنKeywords
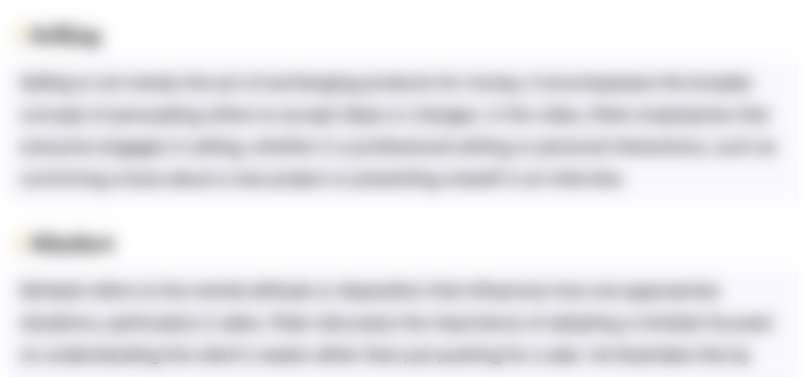
هذا القسم متوفر فقط للمشتركين. يرجى الترقية للوصول إلى هذه الميزة.
قم بالترقية الآنHighlights
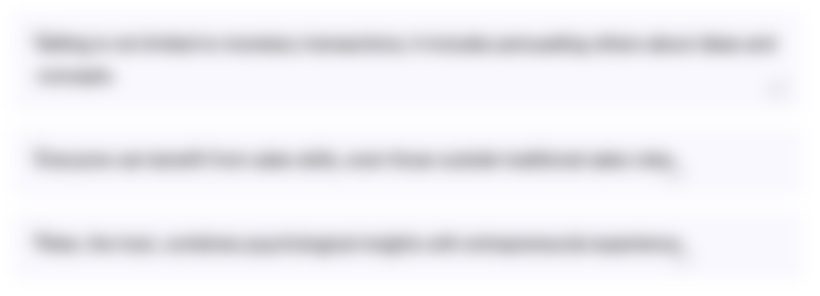
هذا القسم متوفر فقط للمشتركين. يرجى الترقية للوصول إلى هذه الميزة.
قم بالترقية الآنTranscripts
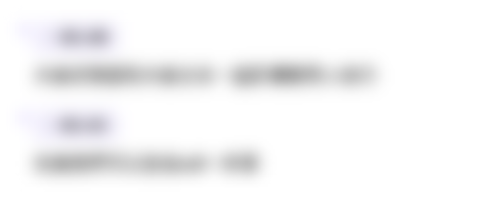
هذا القسم متوفر فقط للمشتركين. يرجى الترقية للوصول إلى هذه الميزة.
قم بالترقية الآنتصفح المزيد من مقاطع الفيديو ذات الصلة

Encoder-Decoder Architecture: Overview
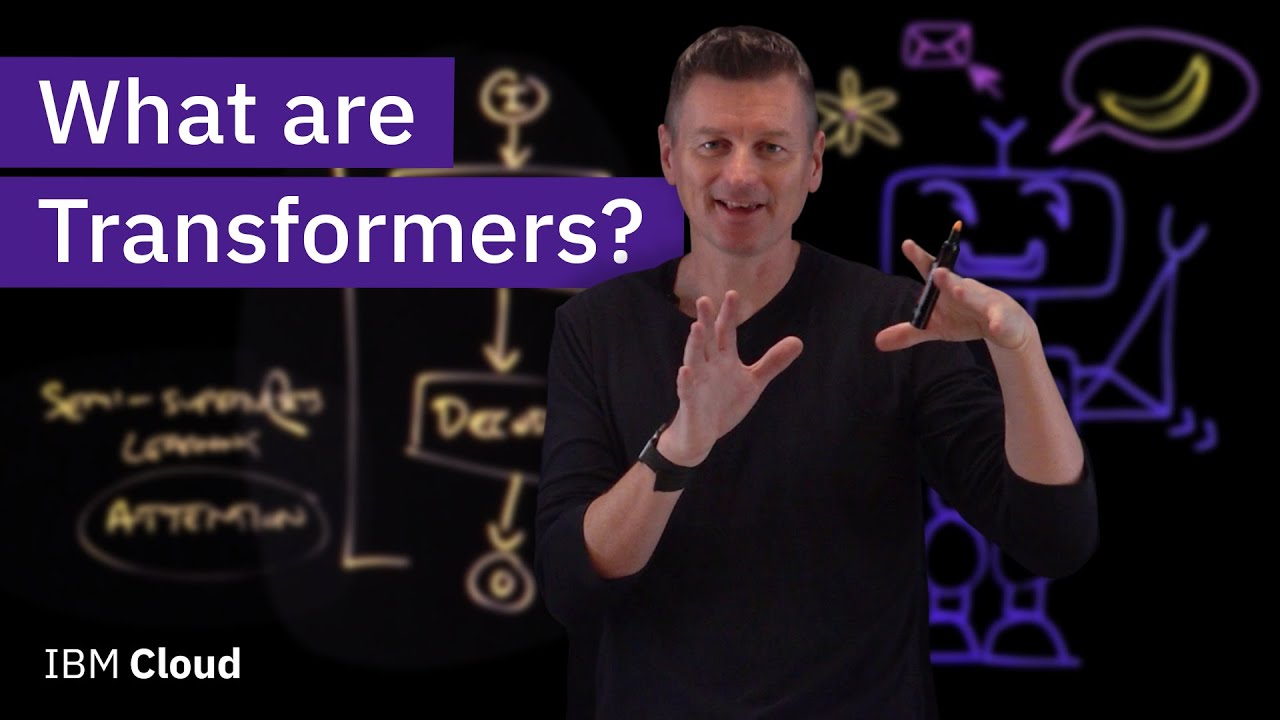
What are Transformers (Machine Learning Model)?
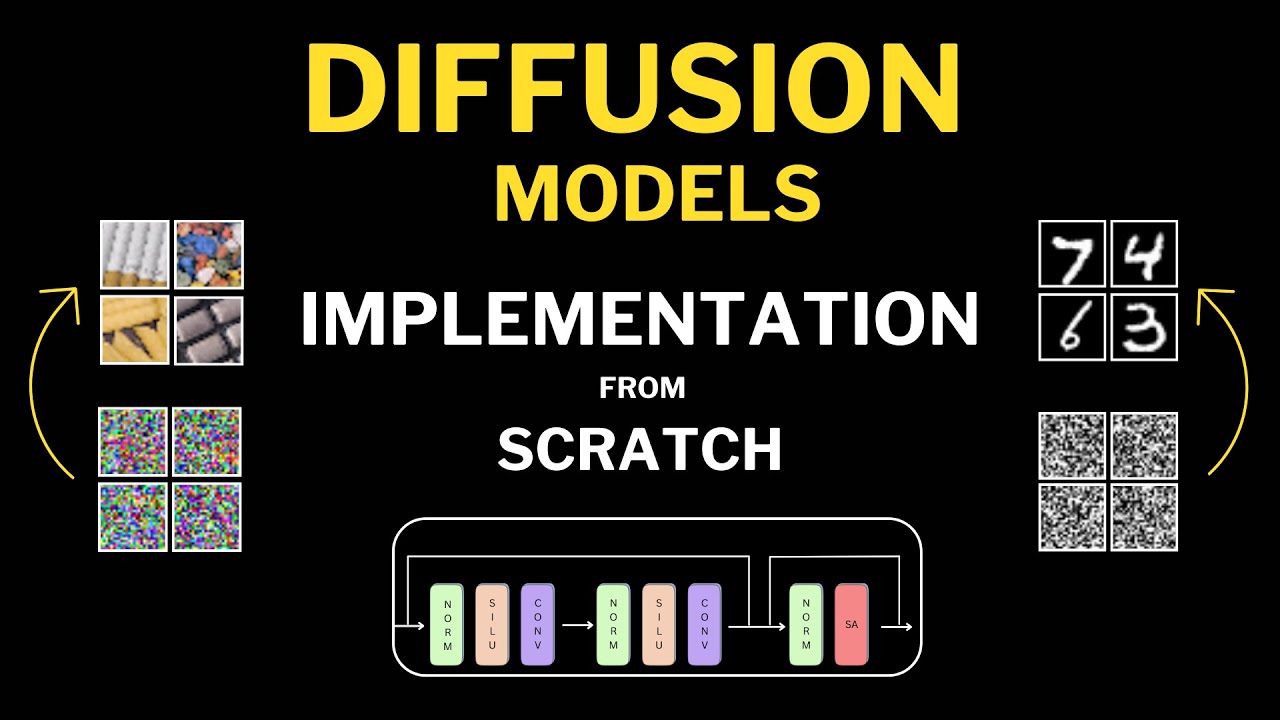
Denoising Diffusion Probabilistic Models Code | DDPM Pytorch Implementation

Illustrated Guide to Transformers Neural Network: A step by step explanation

Attention Mechanism: Overview

Attention for Neural Networks, Clearly Explained!!!
5.0 / 5 (0 votes)