SEM Series (2016) 3. Exploratory Factor Analysis (EFA)
Summary
TLDRThis video script details a comprehensive guide to conducting Exploratory Factor Analysis (EFA). It emphasizes the importance of reflective latent measures, excluding formative and categorical variables, and setting up the analysis with maximum likelihood extraction. The speaker discusses the significance of KMO and Bartlett's test, communalities, and factor extraction. They address issues like cross-loadings and low factor loadings, suggesting strategies for resolving them. The script concludes with reporting the final pattern matrix, factor correlations, and reliability analysis, ensuring convergent and discriminant validity.
Takeaways
- 📊 The speaker begins by emphasizing the importance of saving data sets after making changes, suggesting to save the data as 'trimmed and no missing'.
- 🔄 The process of reordering variables in the data set is discussed, with imputed variables being moved to the end and key demographic variables placed at the bottom.
- 🔍 The speaker conducts an exploratory factor analysis (EFA), highlighting the need to include only reflective latent measures and excluding formative measures, categorical variables, and demographics not part of a reflective latent construct.
- 🎯 The choice of extraction method is discussed, with the speaker preferring maximum likelihood estimation due to its use in subsequent confirmatory factor analysis (CFA).
- 🔄 The use of Promax rotation is mentioned, which is less forgiving but may be necessary if issues arise with the factor analysis.
- 📉 The speaker discusses the suppression of small coefficients, aiming to focus on loadings greater than 0.5 for meaningful results.
- 📊 The Kaiser-Meyer-Olkin (KMO) measure and the significance of the Bartlett's test are highlighted as part of assessing the adequacy of the factor analysis.
- 📈 The total variance explained by the factors is discussed, with the speaker aiming for over 60% as an ideal threshold.
- 🔍 The pattern matrix is scrutinized for high loadings and cross-loadings, with the speaker identifying and addressing issues such as low loadings and Haywood cases.
- 🔄 The speaker demonstrates how to refine the factor analysis by iteratively removing problematic items and rerunning the analysis until a satisfactory model is achieved.
- 📝 The final step involves reporting the KMO and Bartlett's test results, the total variance explained, non-redundant residuals, the pattern matrix, and factor correlations to assess convergent and discriminant validity.
Q & A
What is the first step the speaker takes in the exploratory factor analysis process?
-The first step the speaker takes is to save the dataset with the changes made, renaming it to 'trimmed and no missing'.
Why does the speaker reorder the variables in the dataset?
-The speaker reorders the variables to place the imputed variables at the end and to organize the dataset with age, ID, gender, frequency, and experience at the bottom for better analysis.
What type of measures should be included in an Exploratory Factor Analysis (EFA) according to the speaker?
-The speaker emphasizes that only reflective, not formative, measures should be included in EFA. Categorical variables and demographics not part of a reflective latent construct should be excluded.
What extraction method does the speaker prefer to use in EFA?
-The speaker prefers to use the maximum likelihood extraction method because it is the same algorithm used in Amos for confirmatory factor analysis.
What rotation method is suggested by the speaker for EFA?
-The speaker suggests using the Promax rotation method as it is less forgiving and can help in resolving issues with factor loadings.
Why does the speaker choose to suppress small coefficients at 0.3 in the analysis?
-The speaker suppresses small coefficients at 0.3 because they are not interested in loadings less than 0.5, which is a threshold for meaningful factor loadings.
What does the speaker look for in the KMO (Kaiser-Meyer-Olkin) measure to assess the adequacy of the factor analysis?
-The speaker looks for a KMO value above 0.7 and ideally about 0.9 to ensure the factor analysis is adequate.
How does the speaker interpret the total variance explained by the factors in the analysis?
-The speaker interprets the total variance explained by the factors as good if it is more than 60%, with 64.5% being a satisfactory result in this case.
What issue does the speaker identify with the pattern matrix and how does it affect the analysis?
-The speaker identifies a discriminant validity issue between decision quality and information acquisition, which affects the convergent validity of information acquisition.
What action does the speaker take to resolve the discriminant validity issue between decision quality and information acquisition?
-The speaker runs another factor analysis excluding all items except decision quality and information acquisition to isolate and resolve the issue.
How does the speaker address items with low loadings in the pattern matrix?
-The speaker considers dropping items with low loadings, such as decision quality one and eight, and re-runs the analysis to see the impact on the pattern matrix and overall model.
What additional analysis does the speaker perform to assess the reliability of the items?
-The speaker performs a Cronbach's alpha scale reliability analysis to assess the internal consistency reliability of the items.
Outlines
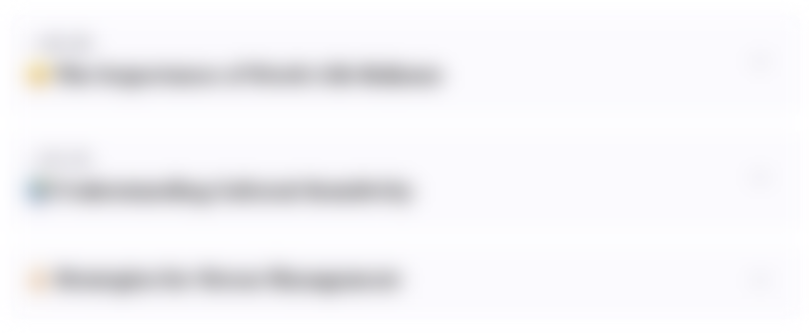
هذا القسم متوفر فقط للمشتركين. يرجى الترقية للوصول إلى هذه الميزة.
قم بالترقية الآنMindmap
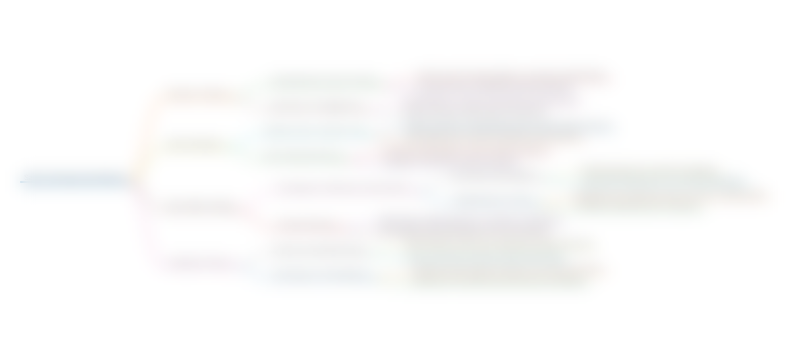
هذا القسم متوفر فقط للمشتركين. يرجى الترقية للوصول إلى هذه الميزة.
قم بالترقية الآنKeywords
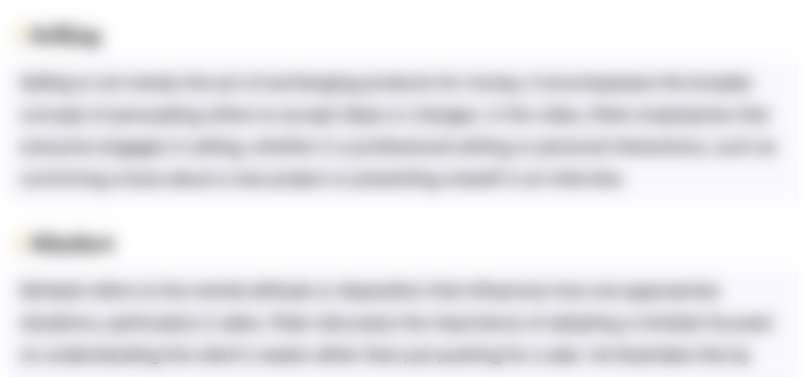
هذا القسم متوفر فقط للمشتركين. يرجى الترقية للوصول إلى هذه الميزة.
قم بالترقية الآنHighlights
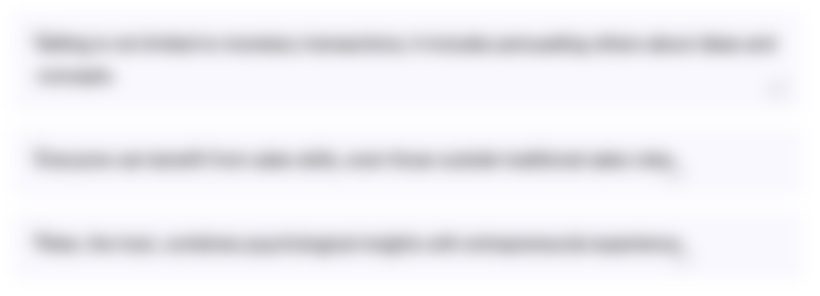
هذا القسم متوفر فقط للمشتركين. يرجى الترقية للوصول إلى هذه الميزة.
قم بالترقية الآنTranscripts
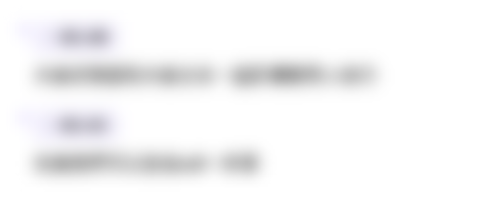
هذا القسم متوفر فقط للمشتركين. يرجى الترقية للوصول إلى هذه الميزة.
قم بالترقية الآنتصفح المزيد من مقاطع الفيديو ذات الصلة
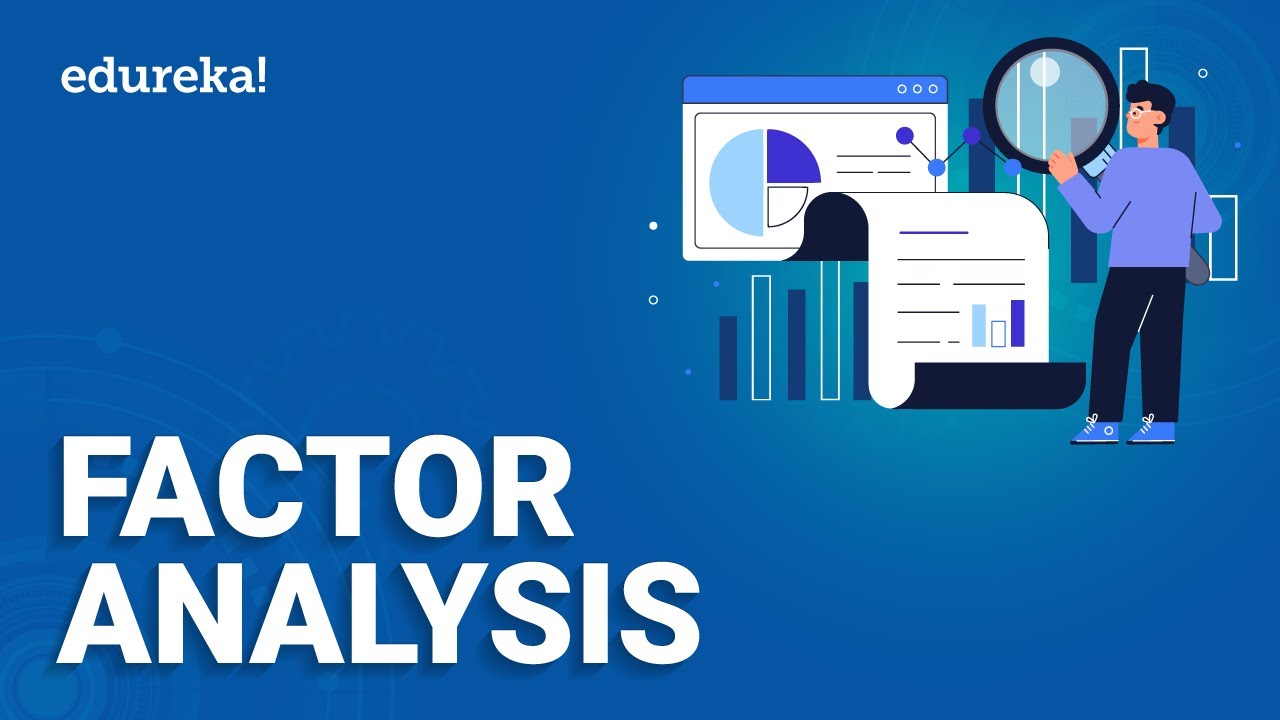
Factor Analysis | What is Factor Analysis? | Factor Analysis Explained | Machine Learning | Edureka

SPSS Factor Analysis for Beginners 1 - Basic Ideas

Gel Electrophoresis Protocol
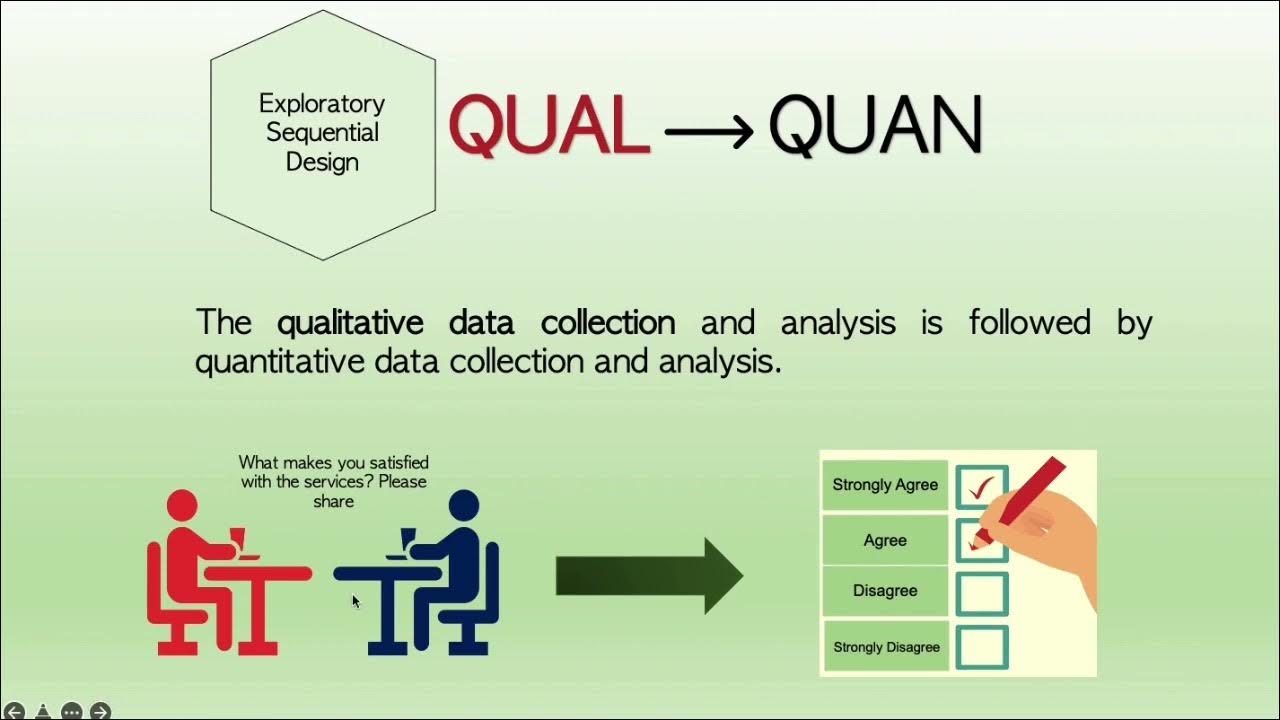
EXPLORATORY SEQUENTIAL MIXED METHOD RESEARCH DESIGN

SEM Series (2016) 5. Confirmatory Factor Analysis Part 2
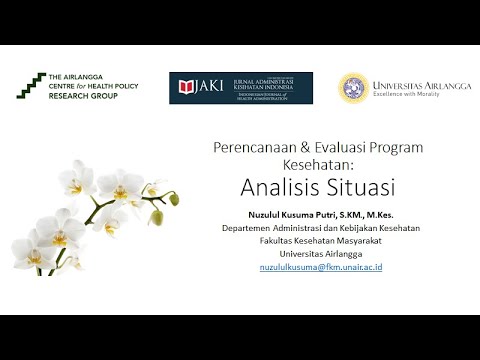
Analisis situasi
5.0 / 5 (0 votes)