Introduction to Data Science - Fundamental Concepts
Summary
TLDRSiobhan Kadar introduces the 2017 Data Science Bootcamp, outlining the interdisciplinary nature of data science and its importance in extracting insights from structured and unstructured data. She discusses the skills required to be a data scientist, including computer science, statistics, and domain expertise, and emphasizes the growing demand for such professionals. The presentation covers the role of data scientists in making sense of data, the significance of data analysis in various industries, and concludes with recommended books for further learning.
Takeaways
- 📊 Data Science is an interdisciplinary field focused on using scientific methods, processes, and systems to extract knowledge or insights from structured or unstructured data.
- 🌟 The demand for data scientists is high across industries due to the vast amounts of data available and the need to extract value from it.
- 👨💻 Hal Varian, Chief Economist at Google, emphasizes the importance of the ability to understand, process, extract value from, visualize, and communicate data.
- 🏆 Data science jobs have been recognized for their work-life balance and are considered one of the 'sexiest jobs of the 21st century' by the Harvard Business Review.
- 🧠 A data scientist must have a unique blend of skills, including more computer science knowledge than a statistician and more statistics than a computer scientist.
- 📈 The role of a data scientist involves cleaning, processing, analyzing data, and drawing inferences to make sense of complex datasets.
- 💡 Data scientists are equipped with knowledge in statistics, machine learning, linear algebra, programming, mathematics, data visualization, and domain expertise.
- 🌐 The availability of inexpensive computing power and cloud services like AWS, Google Cloud Platform, and Microsoft Azure has facilitated data analysis on a large scale.
- 🔍 Data scientists use advanced machine learning and programming to uncover deeper insights and make future predictions, going beyond the capabilities of traditional data analysts.
- 📚 The speaker recommends three books for further reading: 'Data Science for Business', 'The Art of Data Science', and 'The Elements of Statistical Learning'.
Q & A
What is the definition of data science according to the speaker?
-Data science is an interdisciplinary field about scientific methods, processes, and systems to extract knowledge or insights from data in various forms, either structured or unstructured.
Why is data science considered a hot field and important for the future?
-Data science is considered a hot field due to the availability of huge amounts of data and the need for professionals who can understand, process, extract value from, visualize, and communicate data across industries. Hal Varian, Chief Economist of Google, emphasized the importance of these skills for the next decades.
What does the speaker suggest as the key skills for a data scientist?
-A data scientist should know more computer science than a statistician and more statistics than a computer scientist. They must be proficient in statistics, machine learning, linear algebra, programming, mathematics, data visualization, and possess domain expertise.
Why is the demand for data scientists increasing?
-The demand for data scientists is increasing because of the massive amounts of data being collected across industries, the decreasing cost of computing power, and the need for advanced analytics to make future predictions and informed business decisions.
How does the speaker describe the role of a data scientist compared to a traditional data analyst?
-A data scientist uses advanced knowledge of machine learning, programming, and engineering to manipulate data and uncover deeper insights, making future predictions, while a traditional data analyst is bound by SQL queries and analytic packages to extract information from historical data.
What are the steps in data analysis according to the speaker?
-The steps in data analysis are stating the right question, exploratory data analysis, building a model, interpreting the results, and communicating the findings.
What is the significance of Google Trends mentioned in the script?
-Google Trends is significant as it provides an indication of the growing interest in data science over time by showing search trends and the popularity of data science-related queries.
What does the speaker mean by 'data-fication'?
-Data-fication refers to the process of turning previously invisible processes into data, such as quantifying preferences based on likes on Facebook or evaluating the significance of web pages using Google's PageRank algorithm.
What are the types of questions that can be asked during data analysis according to the script?
-The types of questions that can be asked during data analysis include descriptive, exploratory, predictive, causal, and mechanistic.
What are the three books recommended by the speaker for learning data science?
-The three books recommended are 'Data Science for Business' for a general audience, 'The Art of Data Science' for a more technical understanding, and 'The Elements of Statistical Learning' which is a technical book on statistical machine learning.
Outlines
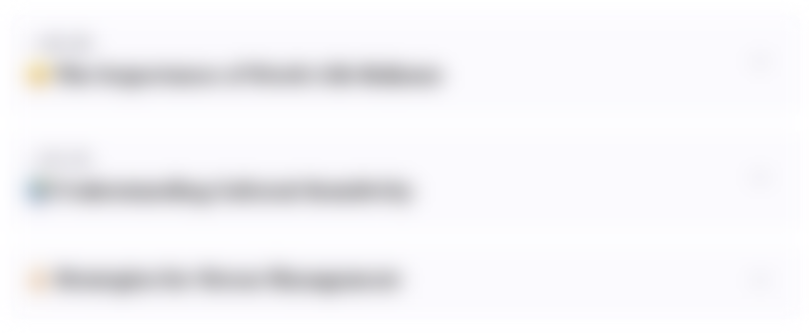
هذا القسم متوفر فقط للمشتركين. يرجى الترقية للوصول إلى هذه الميزة.
قم بالترقية الآنMindmap
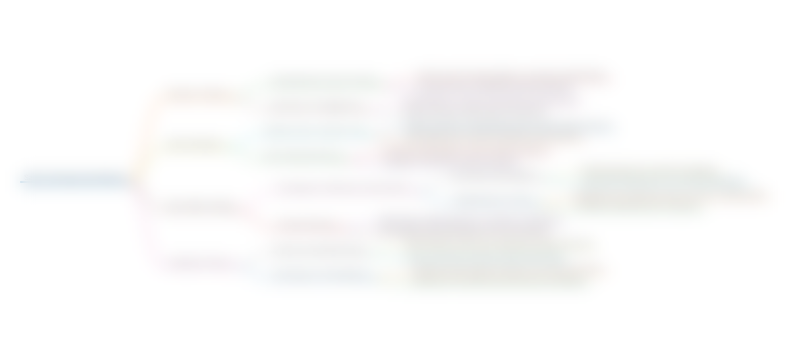
هذا القسم متوفر فقط للمشتركين. يرجى الترقية للوصول إلى هذه الميزة.
قم بالترقية الآنKeywords
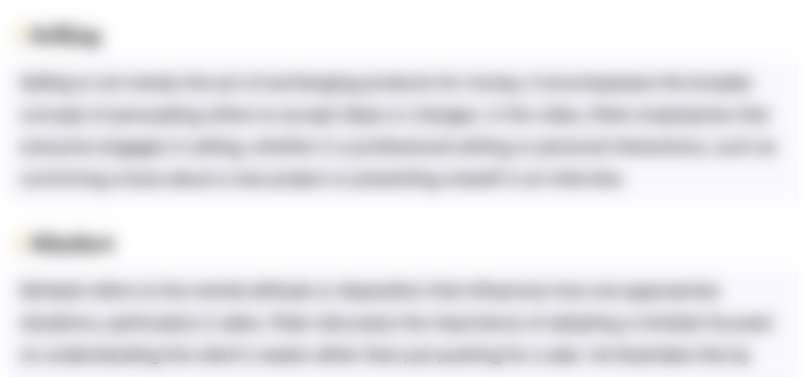
هذا القسم متوفر فقط للمشتركين. يرجى الترقية للوصول إلى هذه الميزة.
قم بالترقية الآنHighlights
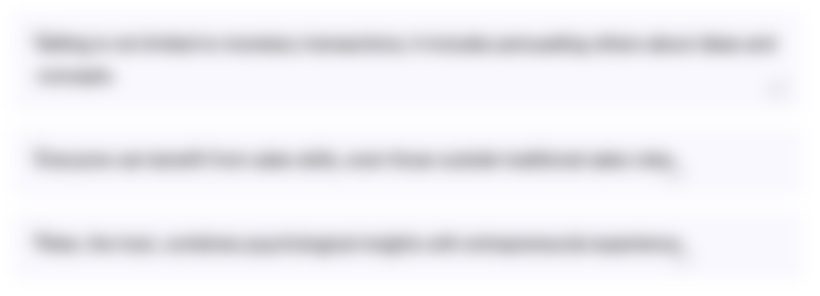
هذا القسم متوفر فقط للمشتركين. يرجى الترقية للوصول إلى هذه الميزة.
قم بالترقية الآنTranscripts
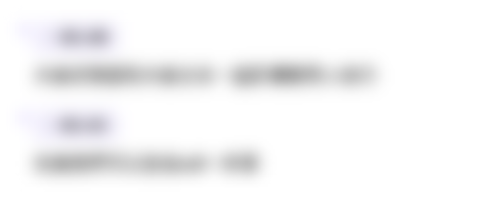
هذا القسم متوفر فقط للمشتركين. يرجى الترقية للوصول إلى هذه الميزة.
قم بالترقية الآنتصفح المزيد من مقاطع الفيديو ذات الصلة
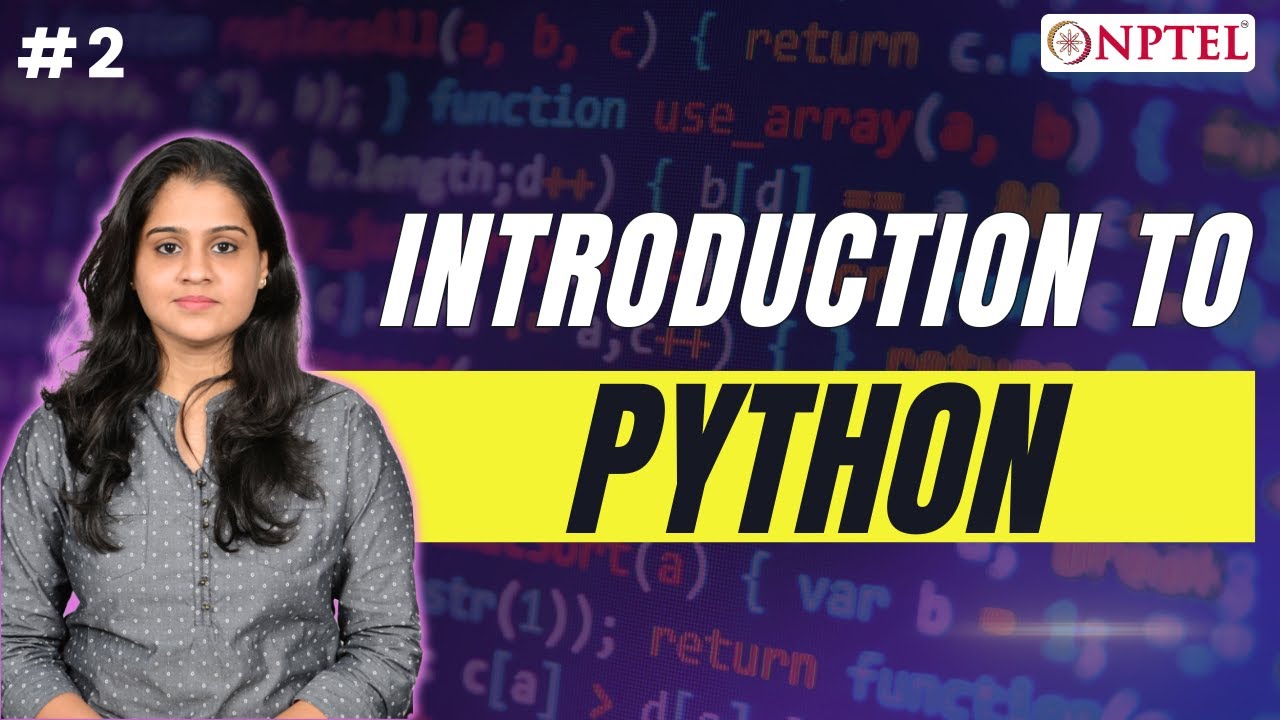
Introduction to Python
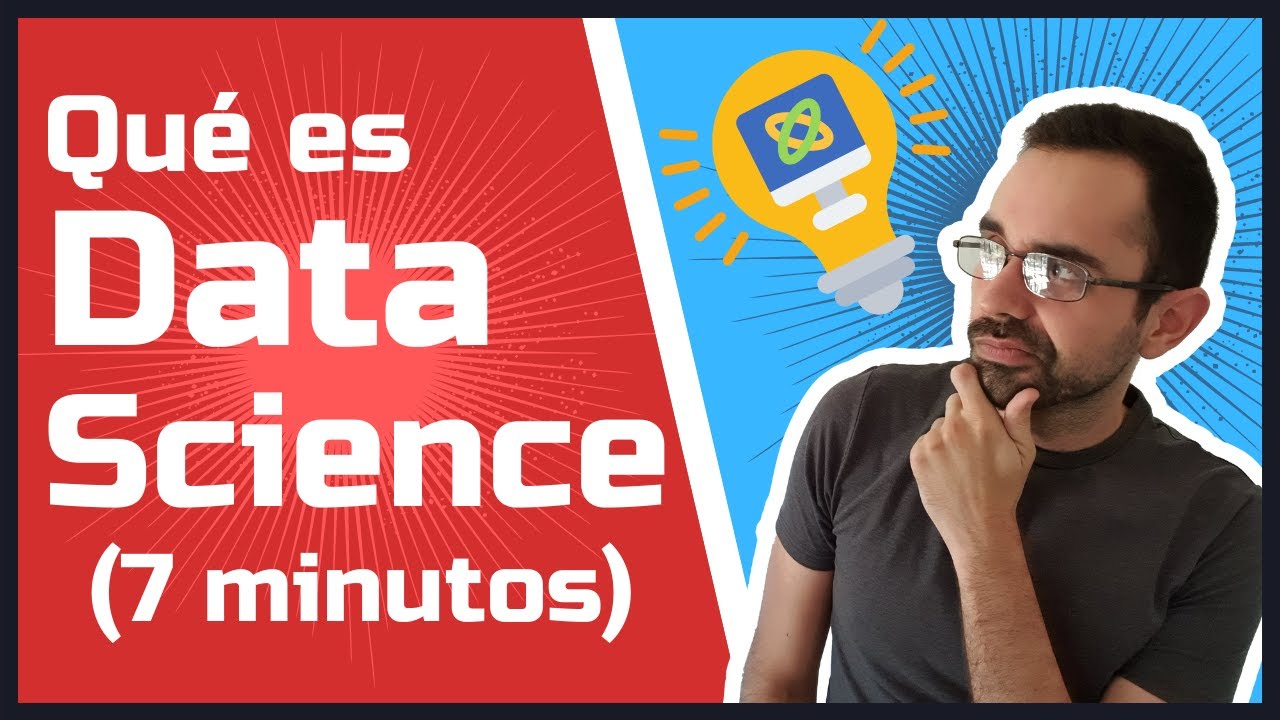
CIENCIA DE DATOS en 7 Minutos ⚡️💻 ¿Qué es Data Science Español?
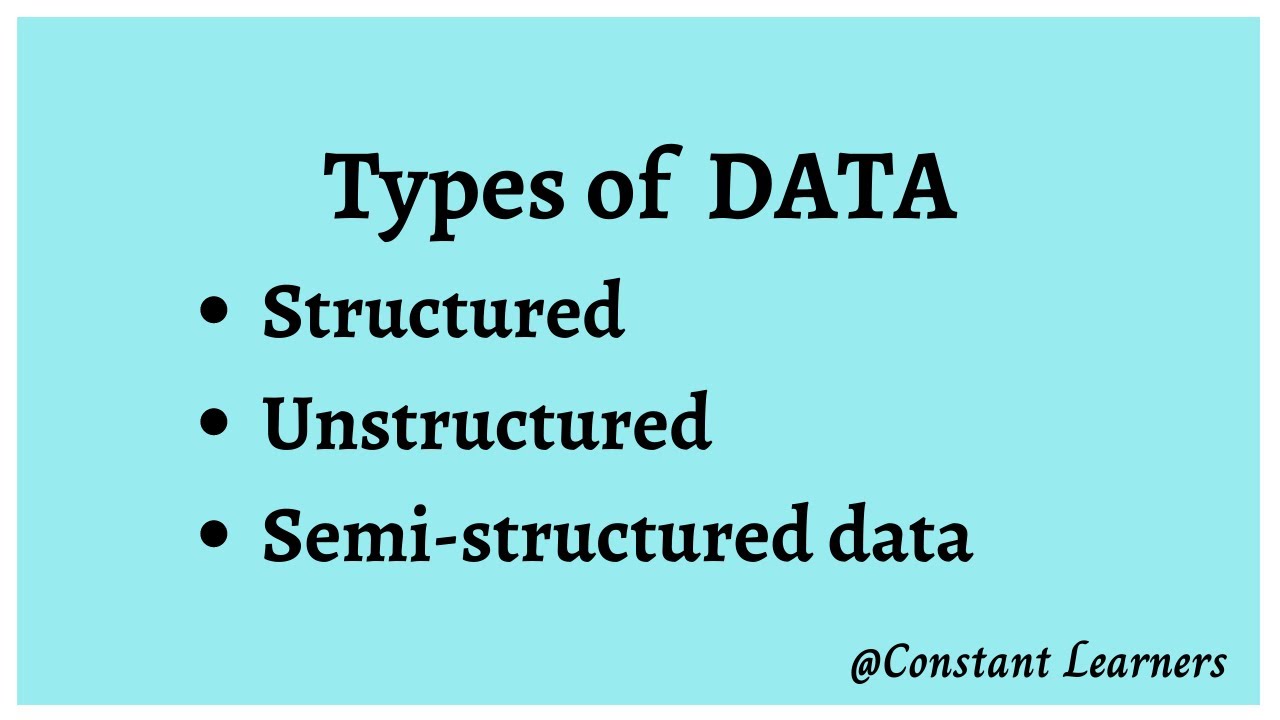
2. What is data? Different types of data? Structured | Semi-structured | Unstructured data
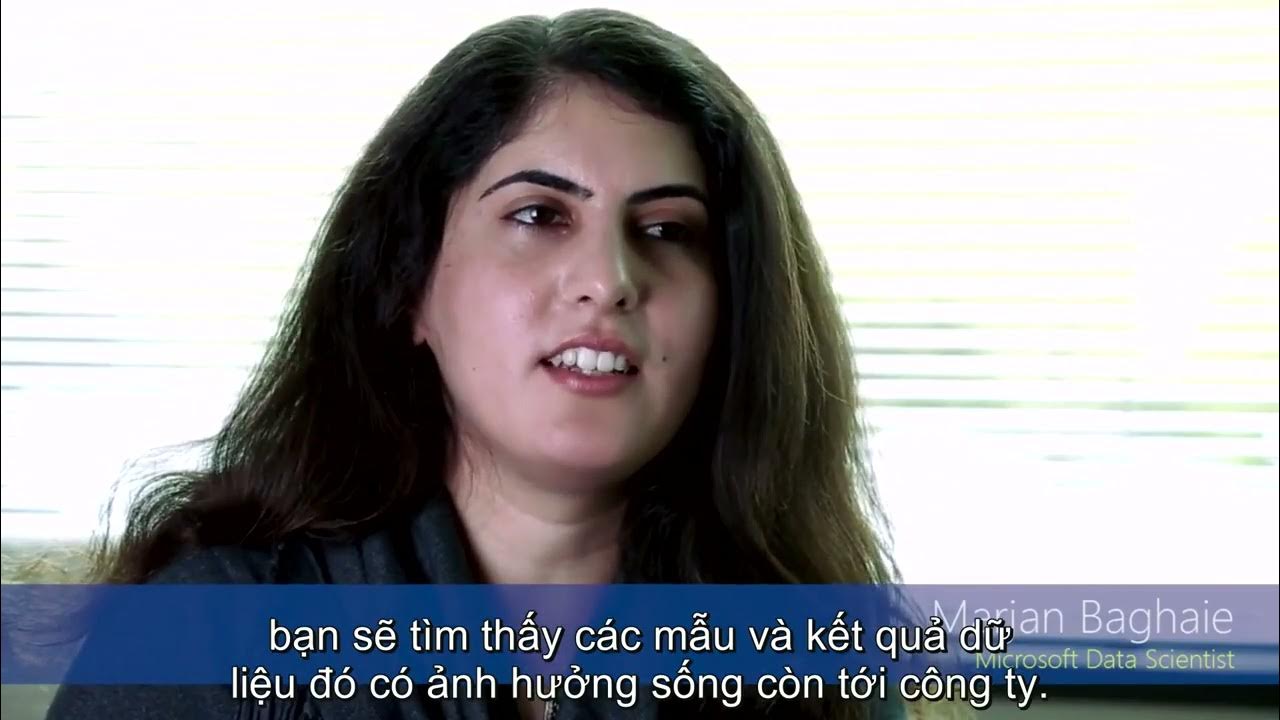
01 Điều gì khiến tôi mong muốn trở thành một nhà khoa học dữ liệu
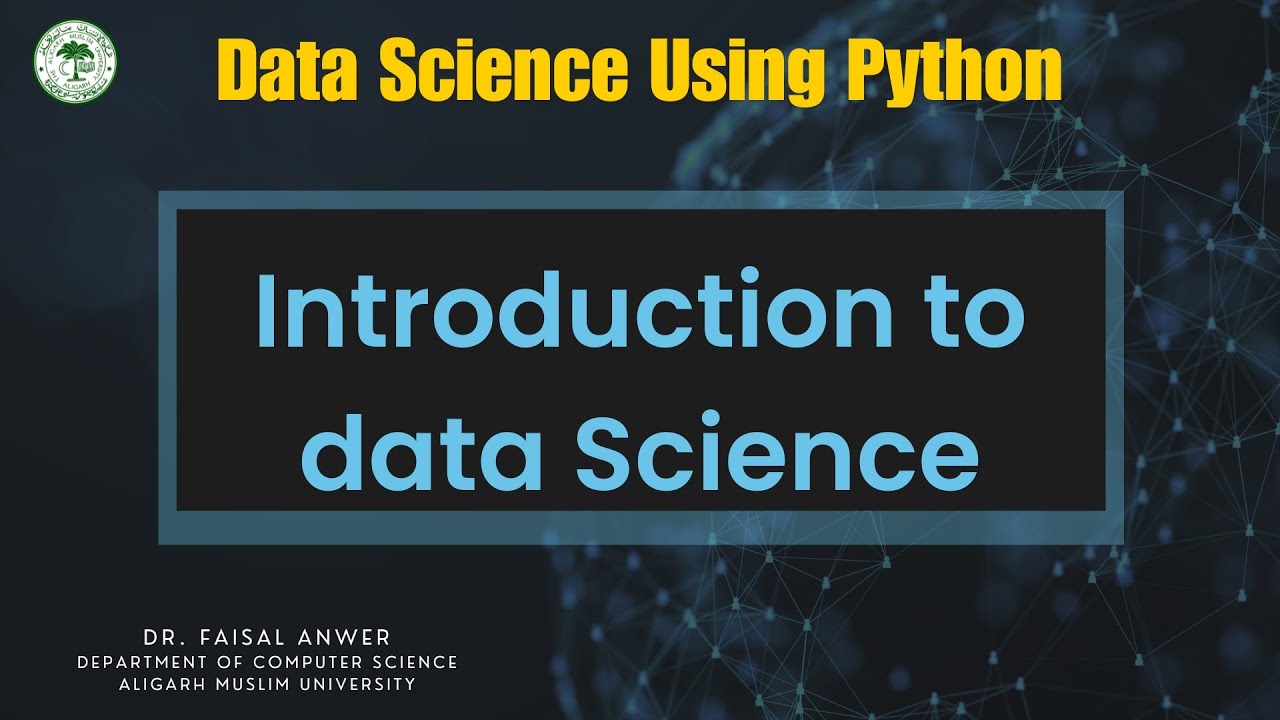
Introduction to data Science
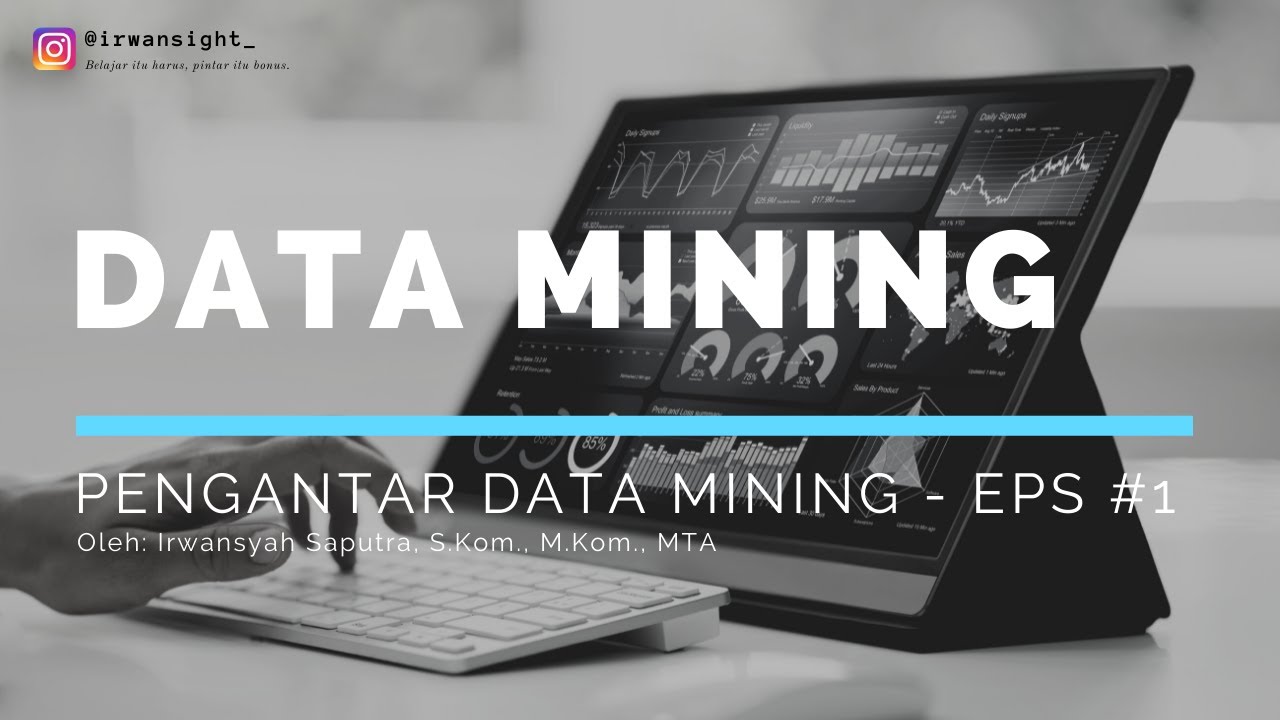
Pengantar Data Mining - #1 Intuisi Kenapa Harus Menggunakan Data Mining
5.0 / 5 (0 votes)