一口气了解英伟达,芯片新王凭什么是他?
Summary
TLDR本视频深入探讨了Nvidia的崛起及其在AI领域的主导地位。从创始人黄仁勋的早期生活开始,讲述了Nvidia是如何从一个专注于3D图形处理芯片的小公司,成长为全球AI训练行业的霸主。视频详细回顾了Nvidia在游戏、加密货币、元宇宙和人工智能等全球科技创新中的关键作用,特别是其在AI硬件和CUDA编程平台上的创新,使其在深度学习和机器学习领域占据了几乎垄断的地位。最后,视频还探讨了Nvidia的市场策略、股价表现和技术挑战,以及它如何应对新兴的竞争对手和市场变化。
Takeaways
- 😀英伟达从1993年成立至今,已经成长为全球显卡行业的龙头老大
- 👌英伟达成功把握住了人工智能和大模型训练这波红利,成为科技行业最大的赢家
- 🤔英伟达CPU可以以4%的成本和1.2%的功耗完成大模型训练
- 😮英伟达的核心业务从游戏转向了数据中心,后者现占56%的营收
- 📈英伟达在Q2的营收高达110亿美元,远超华尔街预期
- 🔥AI训练都需要大量英伟达GPU卡,已成为衡量公司计算力的标准配备
- 🏭英伟达从硬件、软件、服务全面布局,构筑强大生态圈
- ⚠️英伟达的市盈率高达200倍,股价处于高位
- 🌊芯片行业的迭代速度太快,很难形成长期护城河
- 😎英伟达需要在AI浪潮中再战十年,才能守住龙头地位
Q & A
英伟达是如何从一个小公司成长为全球GPU巨头的?
-英伟达从1993年成立开始,经历了多次技术和商业上的风险与失败。但凭借杰出的研发实力、CUDA编程平台的建立、抓住比特币挖矿热潮等关键机遇,以及AI计算需求的爆发,逐步成为GPU市场的主导者。
CUDA对英伟达的发展有什么重要意义?
-CUDA使英伟达的GPU变成可编程的,从而拓展了其应用范围。这为英伟达搭建了完整的生态,使其硬件与软件高度融合,形成了强大的护城河。
为什么AI运算必须依赖GPU?
-GPU拥有大量并行计算核心,特别适合AI模型训练中需要的海量并行简单计算。其算力远超过CPU,已经成为AI训练的标准配置。
芯片行业的特点是什么?
-芯片行业迭代速度极快,门槛高昂,需要大量人才和投入。芯片性能每18-24个月就会翻番,很难依靠单一技术或产品形成持久护城河。研发实力才是核心竞争力。
英伟达如何在芯片行业获得垄断地位?
-通过建立CUDA生态,以及保持高达25%的研发投入占比,英伟达能够保持GPU性能迭代的速度,始终领先竞争对手。这种高强度的技术积累,才是其垄断地位的基石。
英伟达股价飙升的原因是什么?
-英伟达抓住了AI浪潮的机遇,成为AI训练标准配置,未来发展前景广阔。基金和机构为避免漏买,纷纷高价扫货,推高了估值。
英伟达可能面临哪些竞争压力?
-谷歌、Facebook等都在自主研发AI芯片,争夺这片蓝海。另外英伟达并购ARM失败,也暴露了其扩张遭到科技巨头的联合抵制。
黄仁勋对英伟达的发展起到了什么关键作用?
-作为创始人和CEO,黄仁勋对CUDA编程平台的远见,以及高比例研发投入的决策,使英伟达形成了强大的GPU生态并获得AI时代红利。
英伟达的命运会重演3dfx的覆灭吗?
-尽管芯片迭代速度极快,但英伟达成功搭建起GPU+软件生态,并持续巨额投入研发。这为其提供了比3dfx更可靠的护城河,英伟达有望继续扩大优势。
英伟达的四大业务板块中,哪个份额最大?
-2022年,英伟达的数据中心板块收入占比已达56%,超过游戏板块的33%,成为英伟达的最大贡献者。
Outlines
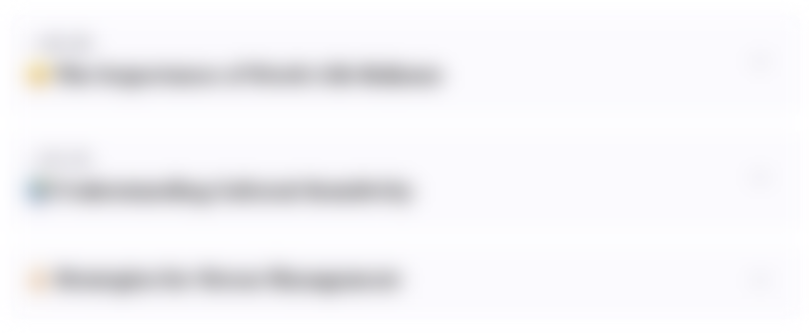
此内容仅限付费用户访问。 请升级后访问。
立即升级Mindmap
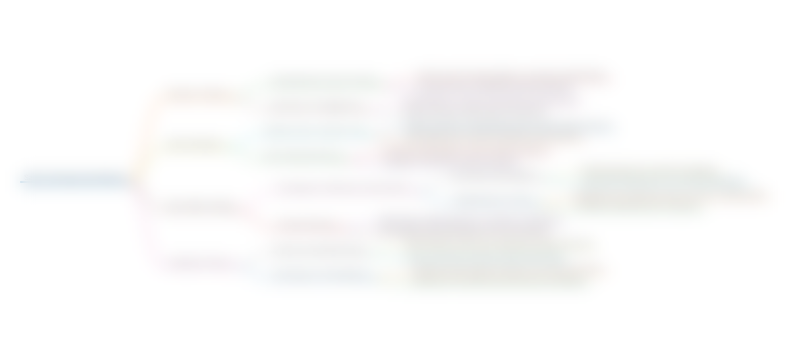
此内容仅限付费用户访问。 请升级后访问。
立即升级Keywords
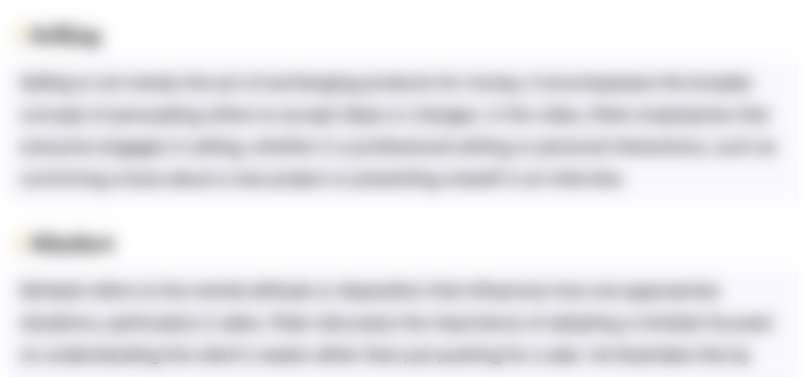
此内容仅限付费用户访问。 请升级后访问。
立即升级Highlights
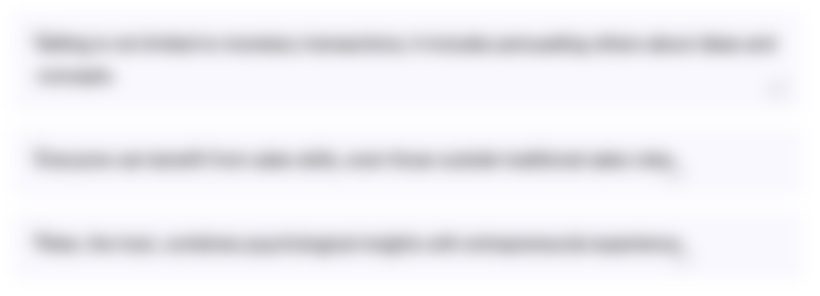
此内容仅限付费用户访问。 请升级后访问。
立即升级Transcripts
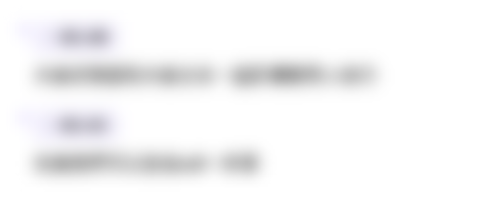
此内容仅限付费用户访问。 请升级后访问。
立即升级浏览更多相关视频
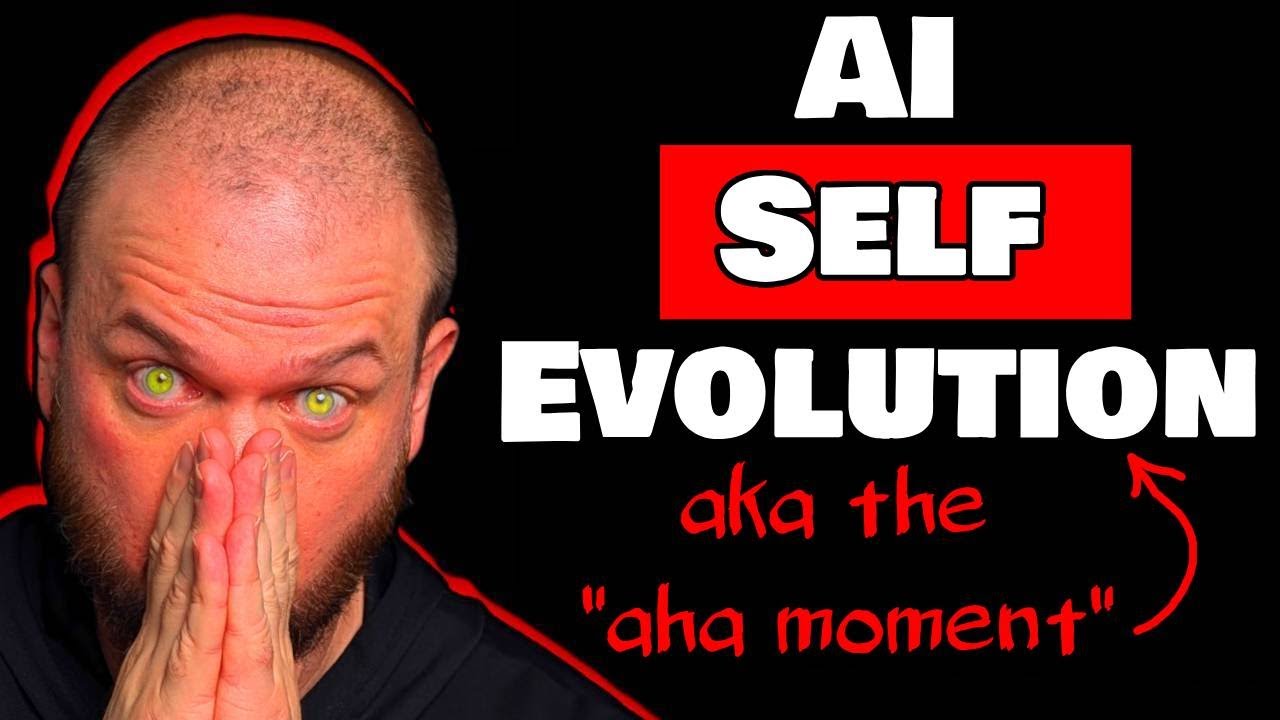
DEEPSEEK DROPS AI BOMBSHELL: A.I Improves ITSELF Towards Superintelligence (BEATS o1)
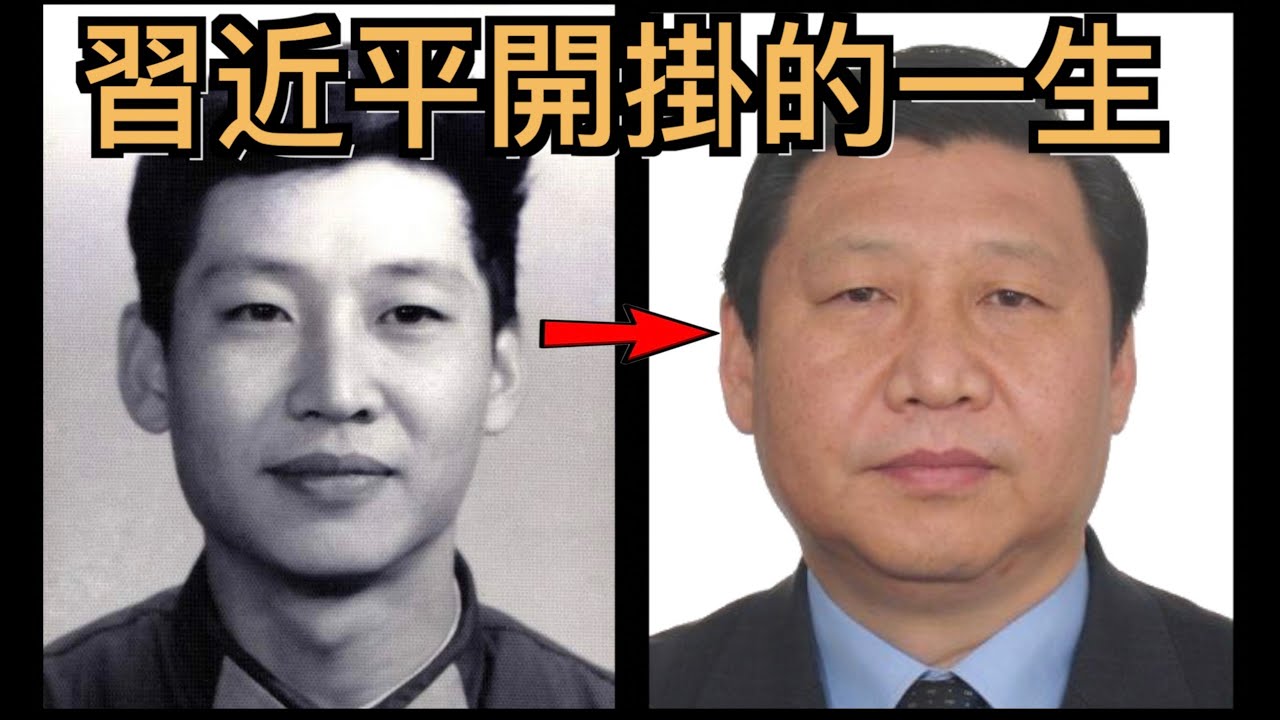
習近平開掛的一生!
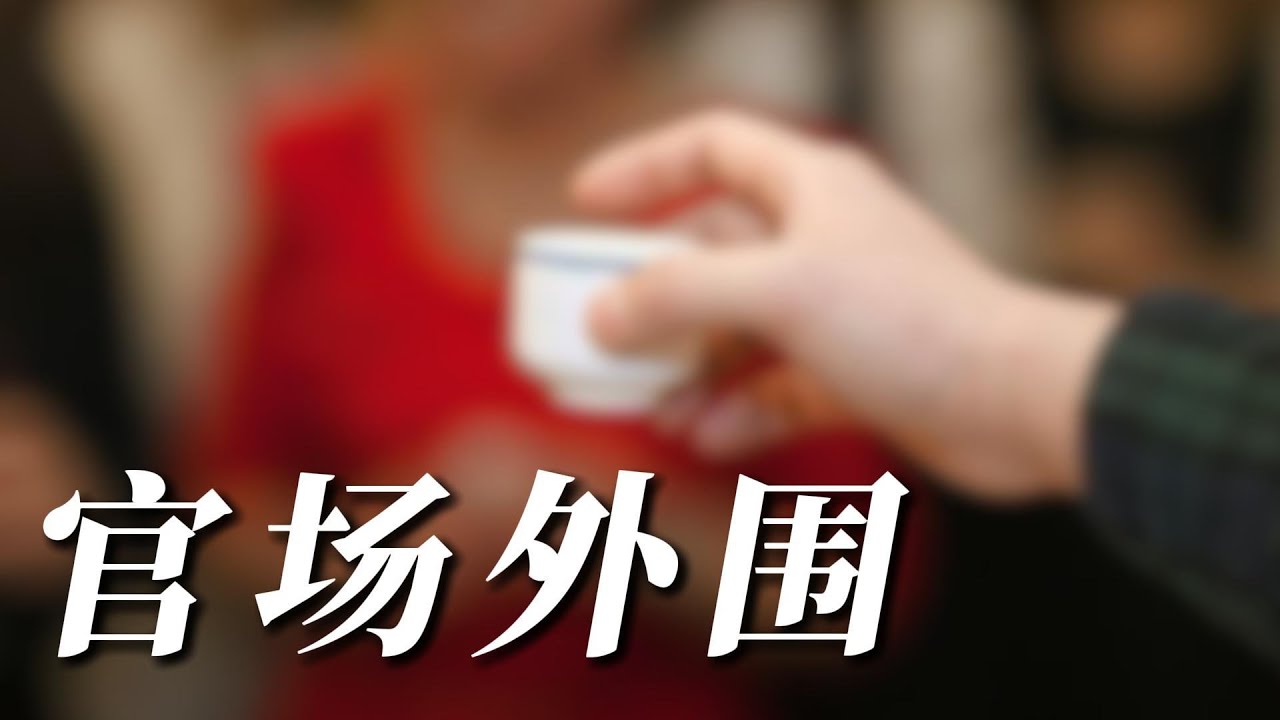
领导们的外围 ~ 另外浅浅聊聊怎么开始给领导送钱
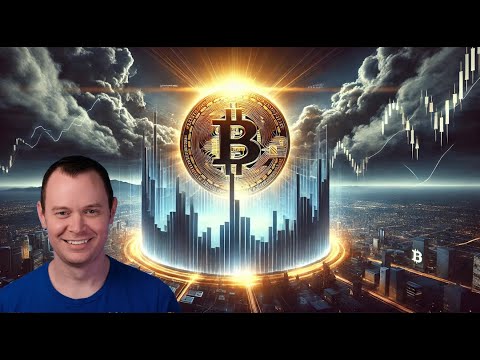
Bitcoin Dominance
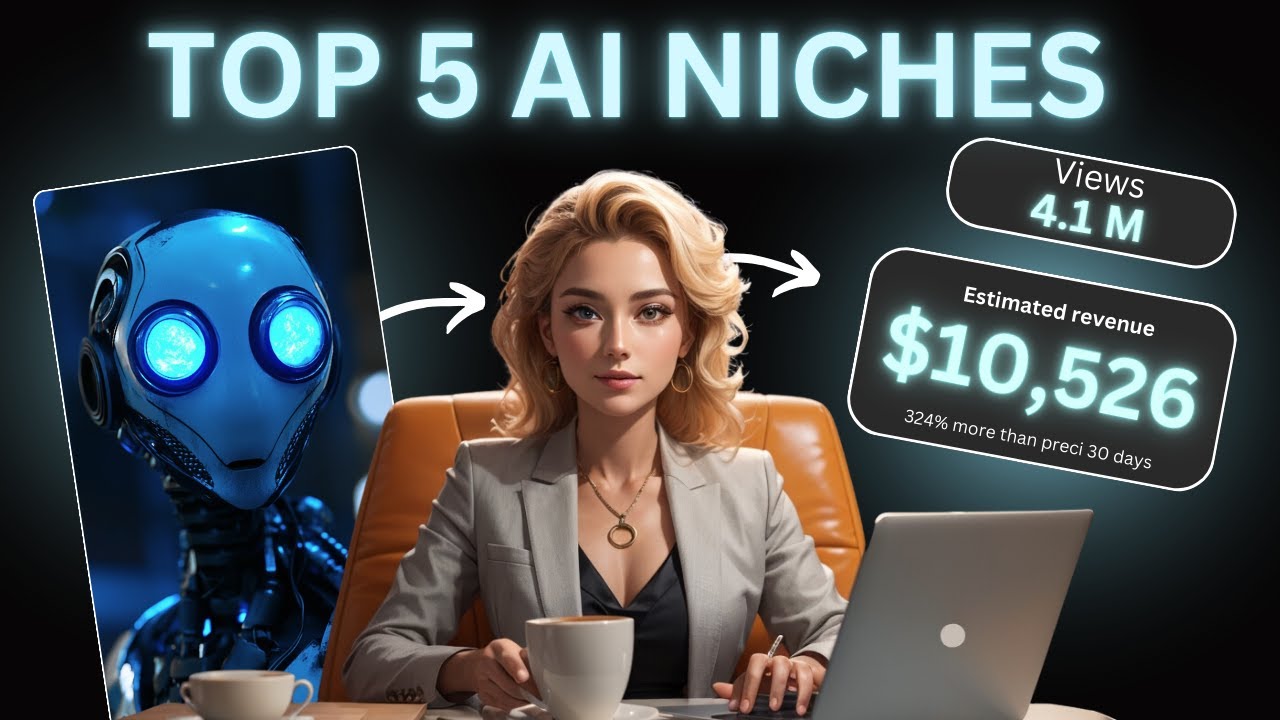
Top 5 Viral Faceless Niches You Can Start In Today | Full Course | Part 2
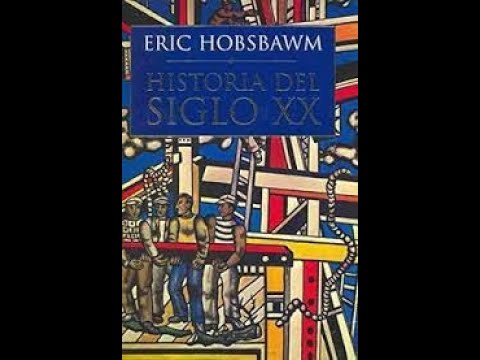
Cap. 5 El Enemigo Común (Historia del Siglo XX)
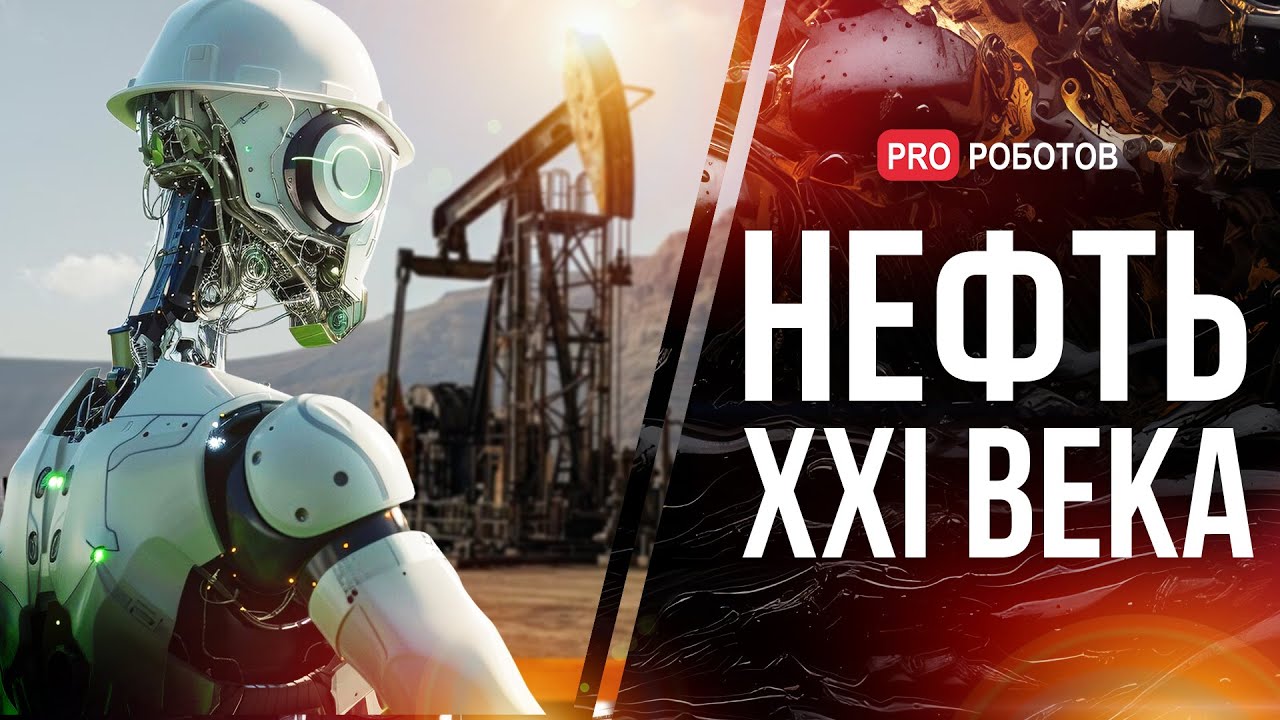
Как ИИ-компании победили Google, Apple и Amazon | Искусственный интеллект: путь к завоеванию мира
5.0 / 5 (0 votes)