When ChatGPT is confidently wrong
Summary
TLDRThe video script warns about the pitfalls of relying on ChatGPT for technical accuracy. Despite its impressive capabilities, ChatGPT can confidently provide incorrect information, as demonstrated by its fabricated response to a question about the first book on SQL. The script emphasizes the need for fact-checking and highlights the difference between convincing and accurate answers, urging caution when using AI as a source of truth.
Takeaways
- 🤖 ChatGPT can be confidently wrong: It generates answers that seem plausible but may not be accurate.
- 💡 Misplaced trust in ChatGPT: Users often believe its outputs without verifying due to a misunderstanding of how generative AI works.
- 📚 Incorrect historical information: ChatGPT provided a fabricated book title and details on SQL's history that were not true.
- 🔍 Importance of fact-checking: The script emphasizes the necessity of verifying ChatGPT's outputs, as they can be misleading.
- 📘 Fabricated citations: ChatGPT created a nonexistent article and author, which highlights the need to cross-reference sources.
- 📘 Misleading publisher information: It wrongly attributed a publisher and report number to a non-existent book, showing AI's limitations in accuracy.
- 🔄 AI's iterative learning: The script acknowledges that AI like ChatGPT is improving but is not yet infallible.
- 📝 Risk of using unverified information: Incorporating ChatGPT's incorrect information in professional work can have serious repercussions.
- 🖼️ Comparison to image generative AI: Just as with image generation, text generation by AI can be convincing but incorrect.
- 🧠 AI's lack of understanding: AI does not truly understand concepts but generates based on patterns in data, which can lead to inaccuracies.
- 🚫 Not a source of truth: ChatGPT should not be used as the sole source of technical information without external validation.
Q & A
What is the main concern raised by the speaker about using ChatGPT for technical content creation?
-The main concern is that ChatGPT can provide answers that look true but aren't, leading to potentially serious implications when used as a technical source of truth.
Why did the speaker purchase ChatGPT+ subscription?
-The speaker purchased the ChatGPT+ subscription because they found ChatGPT amazing and loved it, making the purchase one of the quickest and easiest they've made in years.
What is the example given in the script to illustrate ChatGPT's potential inaccuracies?
-The example is about the first book on SQL, where ChatGPT incorrectly stated the book was 'A Guide to the IBM Relational Database Interface' by Don Chamberlin and Robert Boyce, published in 1974, which does not exist.
What is the fundamental issue with generative AI like ChatGPT when providing information?
-The fundamental issue is that generative AI can generate realistic and convincing answers based on analyzed data, but this does not guarantee accuracy.
How does the speaker describe ChatGPT's behavior when asked for a source of the incorrect SQL book information?
-ChatGPT provided a fabricated source, 'The Birth of SQL' article by Donald Chamberlin in IEEE Annals of the History of Computing Journal, which is non-existent.
What is the role of fact-checking in using ChatGPT for technical information?
-Fact-checking is crucial because ChatGPT can sound extremely convincing even when it is wrong, and relying solely on its output without verification can lead to misinformation.
Why does the speaker compare ChatGPT to generative AIs for images like DALL-E or Stable Diffusion?
-The comparison is made to illustrate that, like image generative AIs, ChatGPT can create outputs that seem plausible but are incorrect, and recognizing inaccuracies in text is more challenging than in images.
What is the speaker's view on the future improvement of generative AI like ChatGPT?
-The speaker believes that generative AI is getting better all the time and that future responses from ChatGPT will continue to evolve, though the accuracy of those responses remains to be seen.
According to the script, what should be done with the information obtained from ChatGPT?
-The information should be fact-checked and not taken at face value, as ChatGPT can confidently provide incorrect information.
What is the implication if someone uses ChatGPT's incorrect information in their technical writing or presentations?
-It could damage their credibility and professionalism if they are called out for using incorrect or fabricated sources.
How does the speaker describe the process of ChatGPT generating responses?
-ChatGPT generates responses by consuming and analyzing massive amounts of data to create realistic-sounding results based on the given prompts.
Outlines
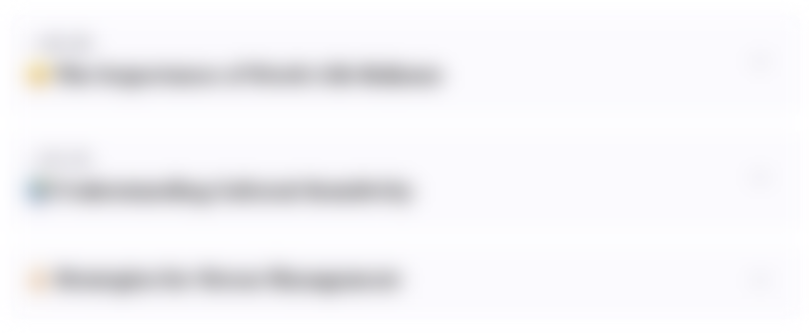
此内容仅限付费用户访问。 请升级后访问。
立即升级Mindmap
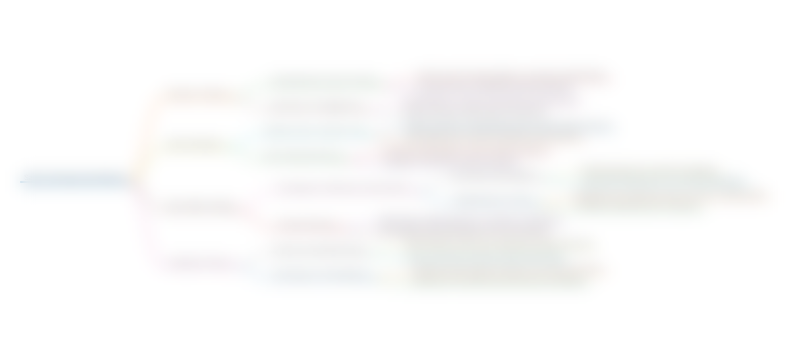
此内容仅限付费用户访问。 请升级后访问。
立即升级Keywords
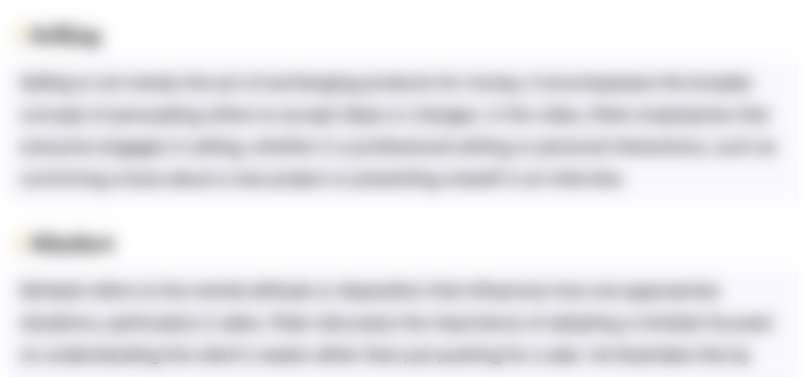
此内容仅限付费用户访问。 请升级后访问。
立即升级Highlights
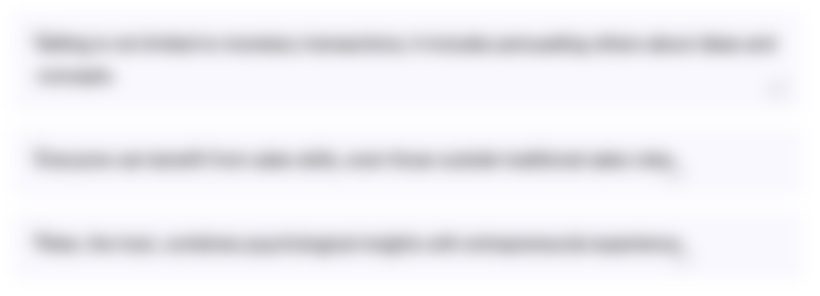
此内容仅限付费用户访问。 请升级后访问。
立即升级Transcripts
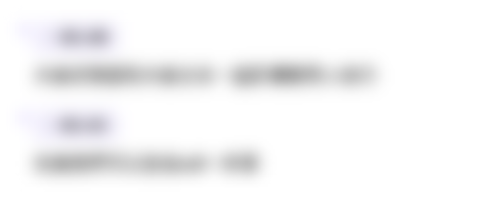
此内容仅限付费用户访问。 请升级后访问。
立即升级5.0 / 5 (0 votes)