Sora Creator “Video generation will lead to AGI by simulating everything” | AGI House Video
Summary
TLDR该视频脚本介绍了一个名为Sora的创新视频生成技术,它通过深度学习和Transformer模型,能够生成高分辨率、一分钟长的视频。Sora技术不仅推动了内容创作的革新,还被视为通向人工智能的重要一步。视频中展示了Sora生成的多样化视频样本,包括复杂的3D场景、物体持久性以及特殊效果等,突显了其在创意表达和模拟现实世界的潜力。同时,讨论了Sora的技术细节,如数据训练、模型扩展性和未来的可能性,以及它在艺术创作和娱乐产业中的潜在应用。
Takeaways
- 🌟 视频生成技术的进步,特别是AI生成的视频,是通向AGI(人工通用智能)的关键路径之一。
- 📹 AI视频生成模型Sora能够创建高清、一分钟长的视频,这在视频生成领域是一个巨大的飞跃。
- 🤖 Sora通过学习理解物理世界的复杂性,包括对象的持久性和3D空间的理解。
- 🎨 Sora的应用前景广泛,包括内容创作、特殊效果和动画制作等,为创作者提供了新的创作工具。
- 🔄 Sora使用Transformer模型,通过在大量不同类型的视频和图像上进行训练,学习生成多样化的内容。
- 🧠 通过增加计算能力和数据,Sora的性能和生成的视频质量将不断提升。
- 🎥 Sora在生成复杂场景和动物行为方面表现出色,显示出对物理世界的深入理解。
- 🏙️ Sora能够生成具有一致3D几何结构的高质量航拍视频。
- 🔄 Sora展示了在视频中插值不同对象的能力,创造出无缝过渡的视觉效果。
- 📈 Sora项目的目标是提升视频生成的质量,同时保持方法的简单性和可扩展性。
- 🚧 尽管Sora取得了显著进展,但在处理某些物理交互和基础物理概念方面仍有待提高。
Q & A
Aji house是如何尊重像在场的各位这样的人物的?
-Aji house通过邀请像在场的各位这样的人物参与,共同创造和体验新技术,来表达对他们的尊重和重视。
视频中提到的Sora项目是什么?
-Sora是一个视频生成项目,它利用团队和众多杰出合作者的力量,通过高级技术手段,能够创造出具有复杂细节和物理世界理解的视频内容。
Sora项目在视频生成方面取得了哪些成就?
-
Outlines
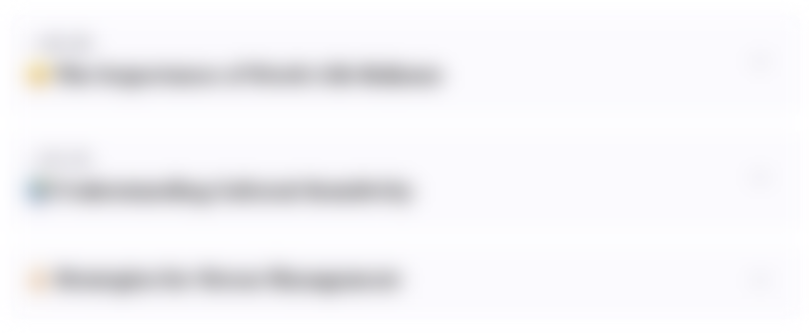
此内容仅限付费用户访问。 请升级后访问。
立即升级Mindmap
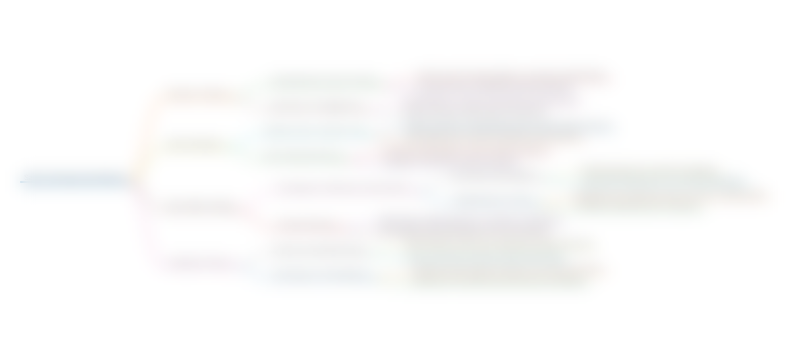
此内容仅限付费用户访问。 请升级后访问。
立即升级Keywords
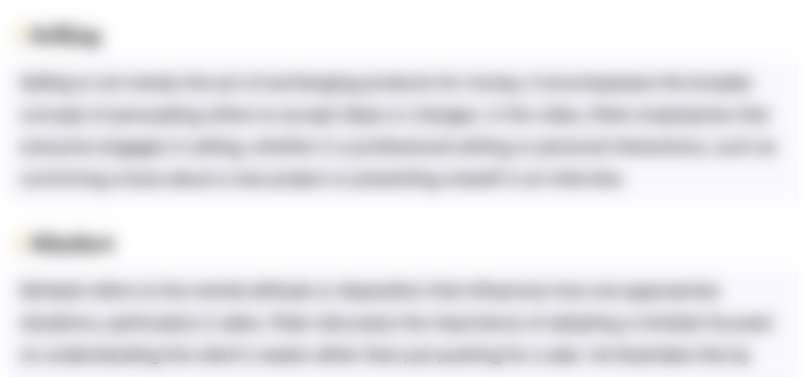
此内容仅限付费用户访问。 请升级后访问。
立即升级Highlights
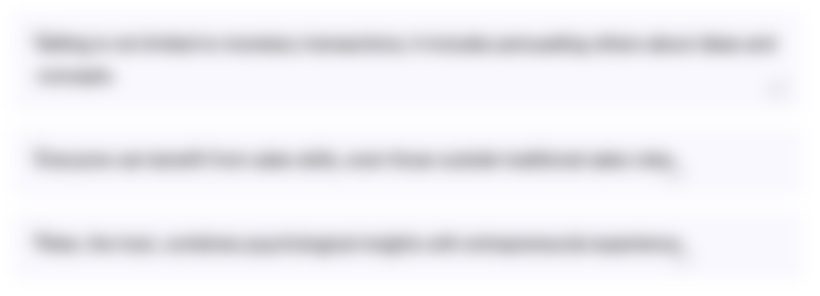
此内容仅限付费用户访问。 请升级后访问。
立即升级Transcripts
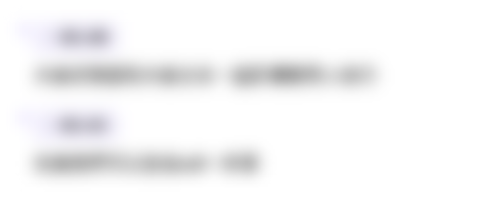
此内容仅限付费用户访问。 请升级后访问。
立即升级浏览更多相关视频
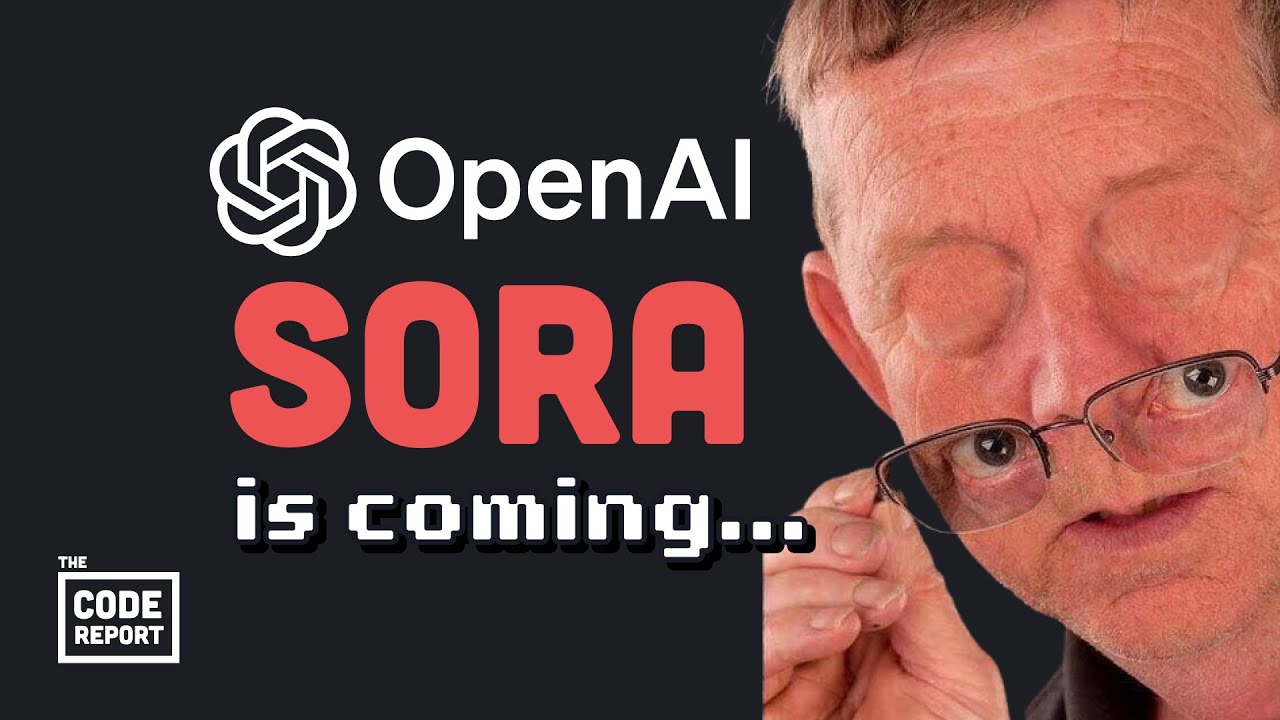
OpenAI shocks the world yet again… Sora first look
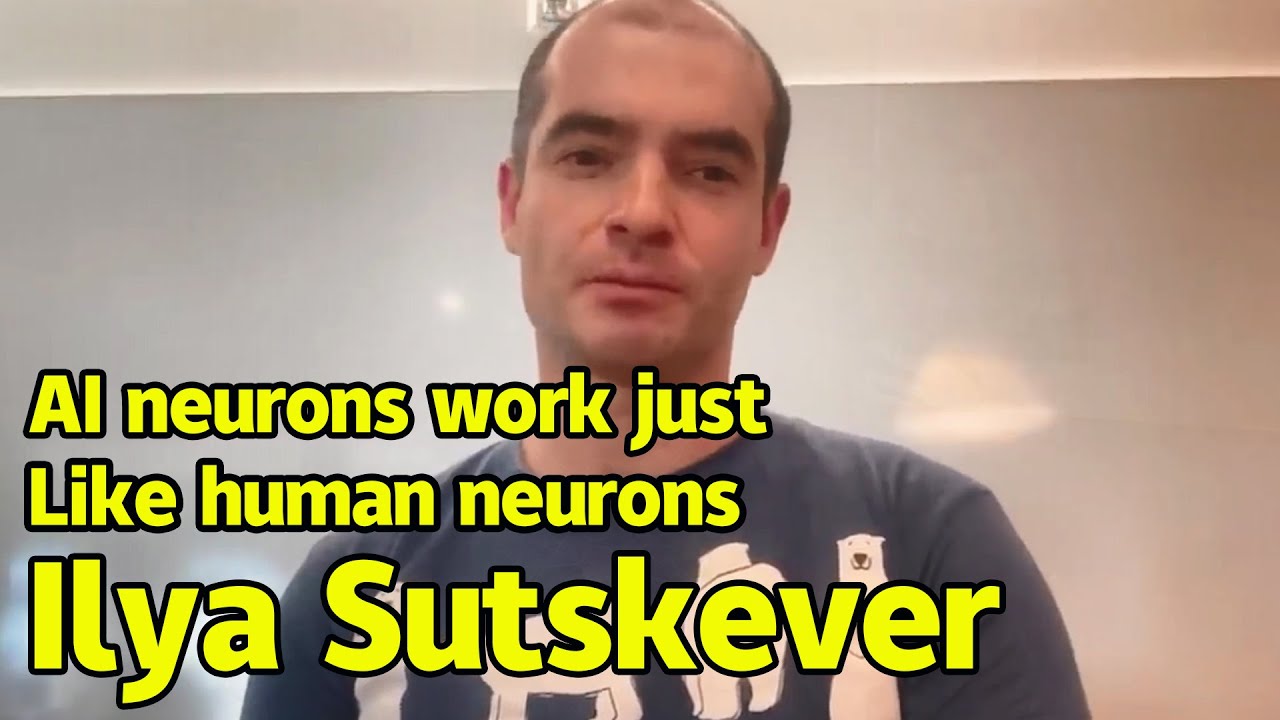
Ilya Sutskever | AI neurons work just like human neurons | AGI will be conscious like humans
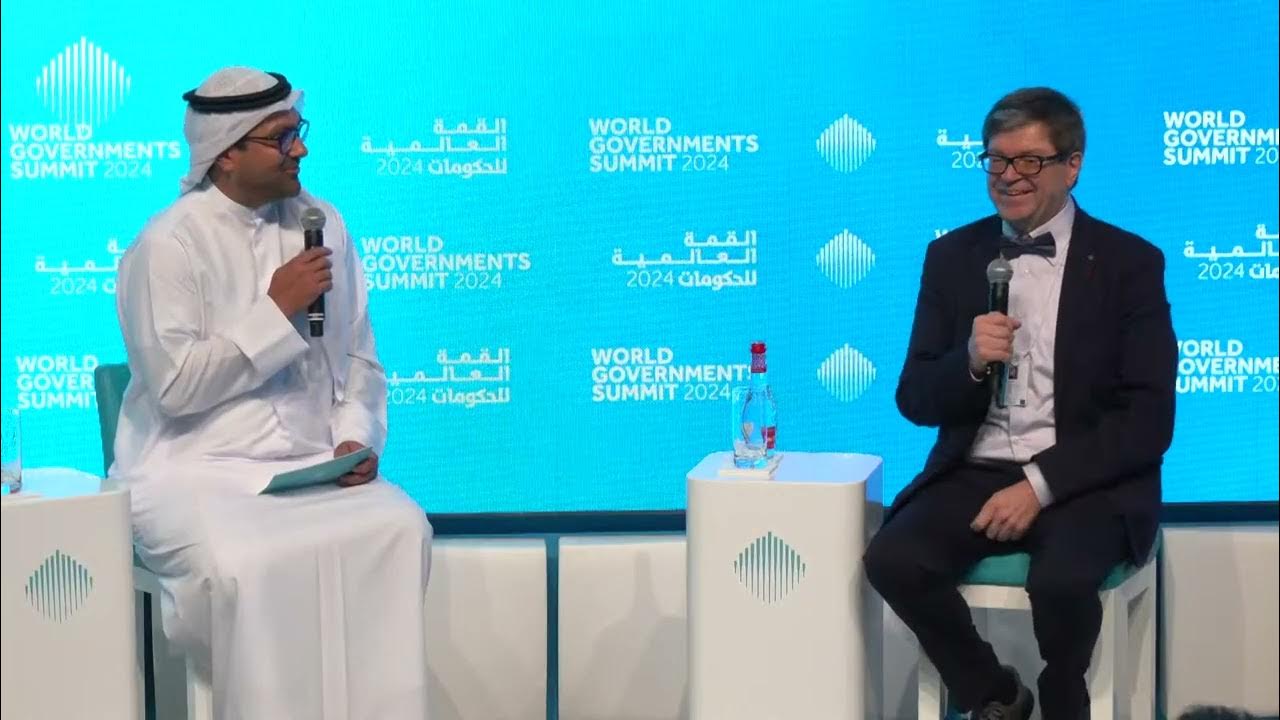
《與楊立昆的對話:人工智能是生命線還是地雷?》- World Governments Summit
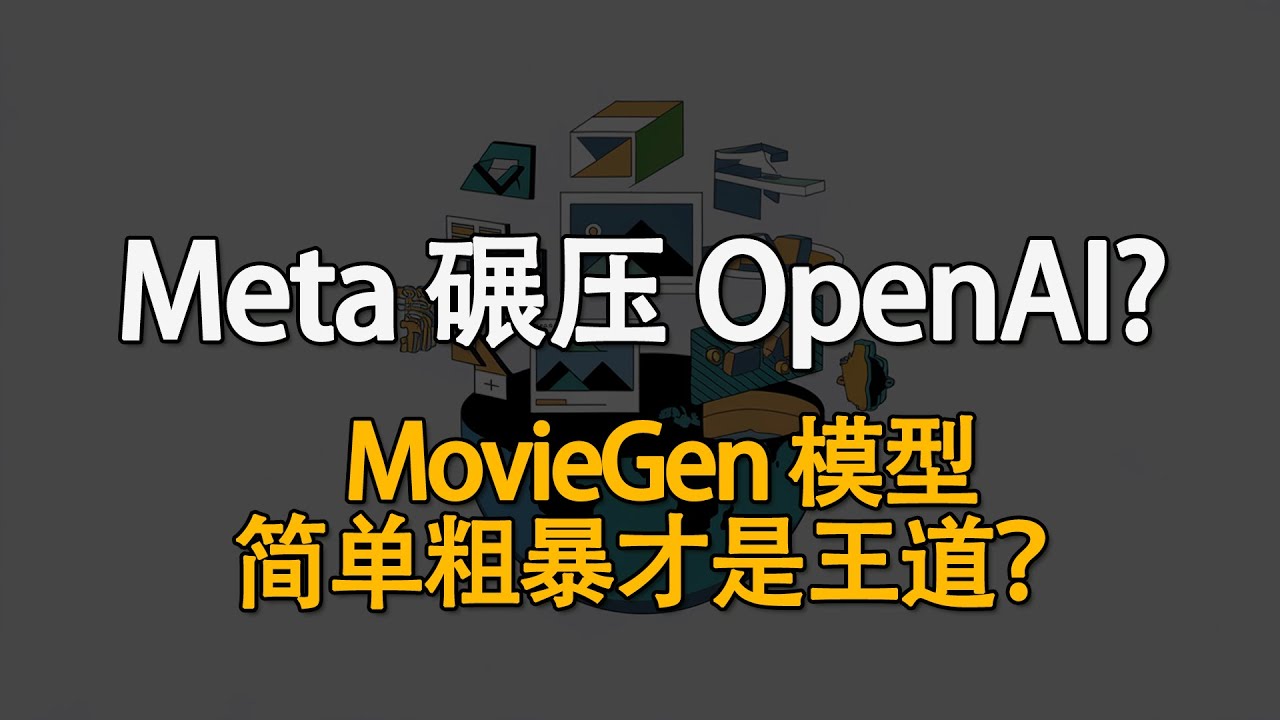
Meta 碾压 OpenAI? MovieGen 模型简单粗暴才是王道?
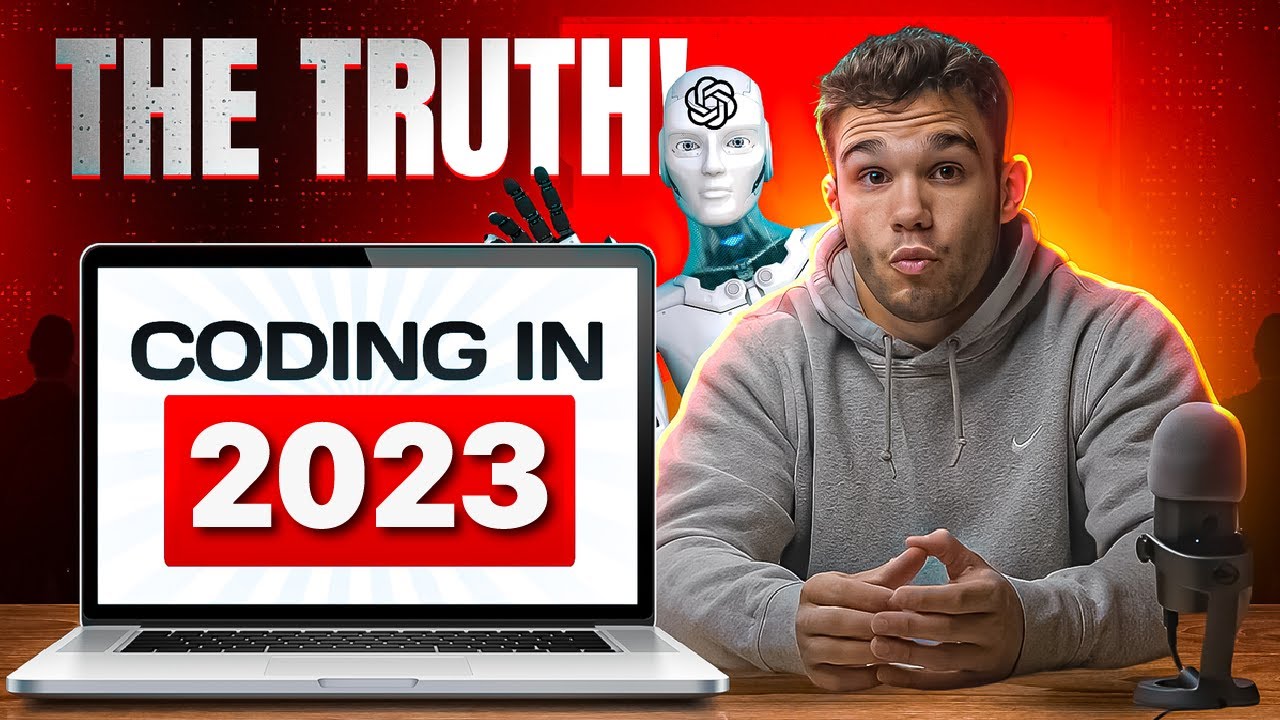
I Spent 6 HOURS Researching The Coding Job Market… Here’s What I Found
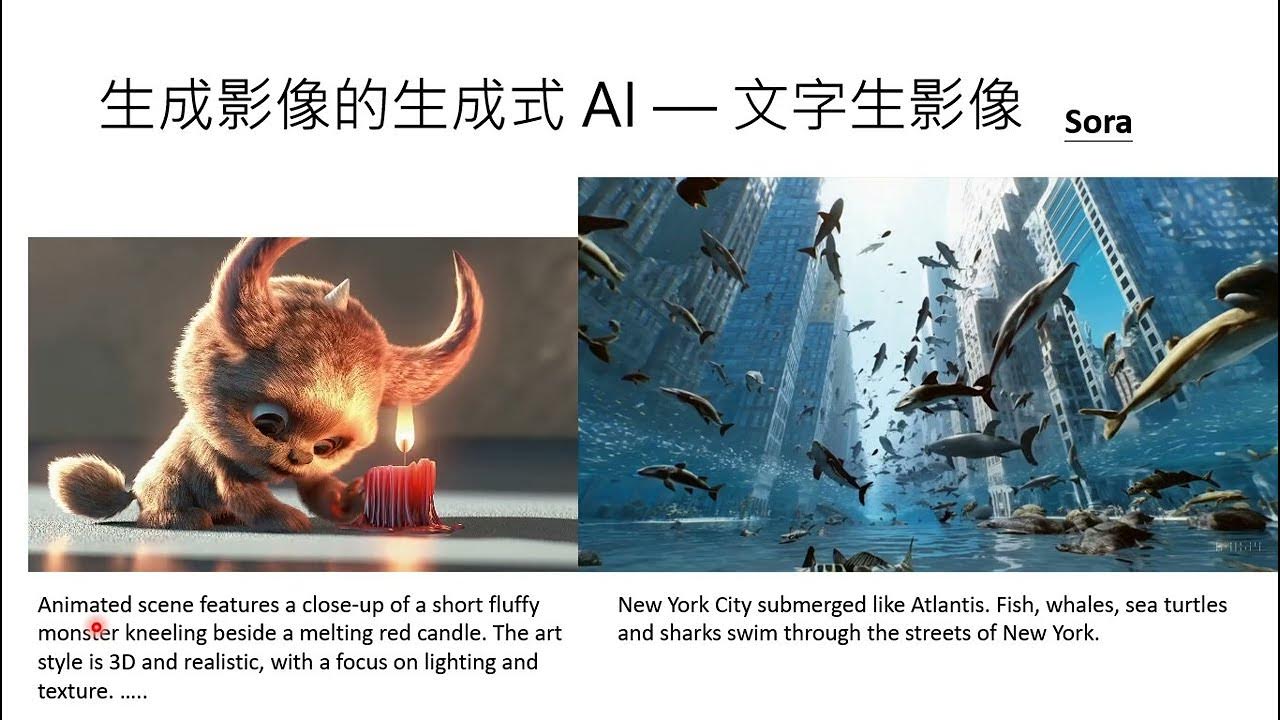
【生成式AI導論 2024】第17講:有關影像的生成式AI (上) — AI 如何產生圖片和影片 (Sora 背後可能用的原理)
5.0 / 5 (0 votes)