《與楊立昆的對話:人工智能是生命線還是地雷?》- World Governments Summit
Summary
TLDR本视频讲述了人工智能(AI)当前激动人心的进展及其对世界的深远影响。从自动驾驶系统到医疗保健和科学研究,AI的应用正在加速科技进步。视频还探讨了AI在内容审核、社交网络中的关键作用,并强调了AI作为解决方案而非问题的重要性。此外,讨论了大型AI模型的开发成本,以及开源技术如何使发展中国家和小型企业能够利用AI推动创新。视频最后强调了AI的未来潜力,包括改善全球知识共享和安全性,同时指出了达到人类级智能所需的技术突破和挑战。
Takeaways
- 🤖 人工智能正在帮助我们变得更聪明,它在短期内已经在交通、医疗保健、药物设计等领域发挥作用。
- 🚗 现代汽车配备了驾驶辅助系统,这些系统能自动识别障碍并停车,预示着未来汽车将实现自动驾驶。
- 🔬 在科学和技术的进步方面,人工智能承诺将加速材料科学和化学等领域的发展。
- 🌐 目前最大的人工智能应用之一是社交网络上的内容审核,这是一个复杂的问题,但AI在解决虚假信息和仇恨言论方面扮演着解决方案的角色。
- 💻 要在人工智能研究的前沿,需要庞大的超级计算机资源,例如至少10,000个GPU。
- 🌍 对于开发中国家和小型公司来说,通过微调开源技术和基础模型,可以实现对人工智能的利用,而不需要巨大的投资。
- 📡 人工智能未来将被视为基础软件平台,类似于互联网,这意味着它的许多部分将基于开源软件。
- 👓 我们将更多地依赖人工智能助手,它们将通过我们的智能手机或智能眼镜等设备提供信息和帮助。
- 🚀 人工智能领域的未来发展将需要新的架构和技术突破,例如提高视频处理能力和改进记忆系统。
- 🌟 对政府和决策者的建议:需要建立人工智能主权,提供教育和训练,并创建国家级的计算资源以促进人工智能生态系统的发展。
Q & A
为什么开源的AI系统很重要?
-开源的AI系统可以被任何人定制,也可以移植到不同的硬件上,应用范围更广。类似互联网的开源软件,开源AI系统可以得到更快的进步。
当前最大的语言模型与猫的大脑相比如何?
-当前最大的语言模型的参数数量相当于猫大脑神经元连接数的一半左右,也就是猫的智力水平的一半。
我们现在距离通用人工智能还有多远?
-通用人工智能可能还需要几十年时间才能实现,至少10年以上。人们总是高估技术进步的速度。
实现通用AI还需要哪些突破?
-需要从视频中学习世界运行规律的新神经网络结构,需要记忆与推理的能力,还需要分层规划与控制的能力。
当前AI最大规模训练需要多少算力?
-前沿的AI研究至少需要数万张GPU,一个有竞争力的规模是16000张GPU,成本在10亿美元以上。
未来算力需求会减少吗?
-硬件和算法都在进步,系统可以使用更低精度,智能设备会内置小型神经网络芯片,未来算力需求会有所降低。
开源与专有AI系统的区别是什么?
-开源系统应用范围更广,更容易定制,但专有系统集成度更高,使用更简单。开源AI最终会超过专有系统。
为什么说生成对抗网络不是AI的未来?
-如果AI系统能从视频中学习世界运行规律,它们不大可能是生成对抗网络,这种监督学习更适合未来的AI。
各国应如何实现AI主权?
-各国应共享少数开源基础模型,同时投资教育和算力资源,在此基础上开发自定义的AI应用。
人工智能技术给人类带来的威胁有多大?
-目前的人工智能实际上还远未达到令人担心的水平。但在更长远的未来,机器的智能可能会超过人类。
Outlines
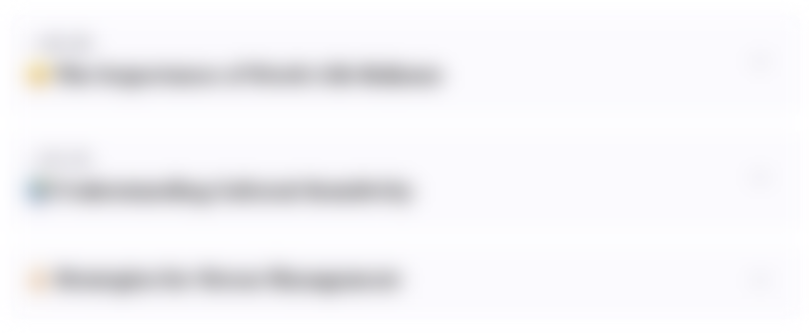
This section is available to paid users only. Please upgrade to access this part.
Upgrade NowMindmap
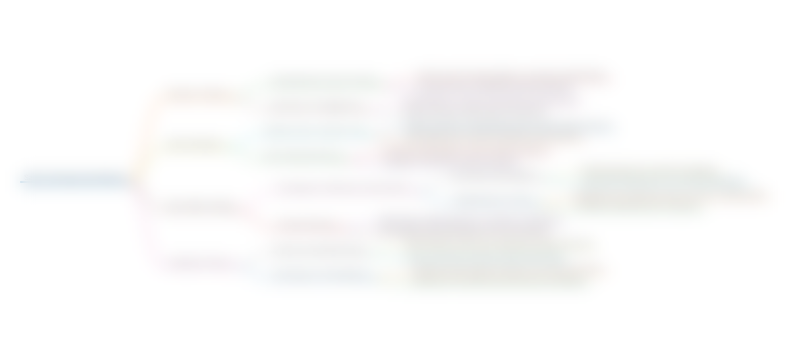
This section is available to paid users only. Please upgrade to access this part.
Upgrade NowKeywords
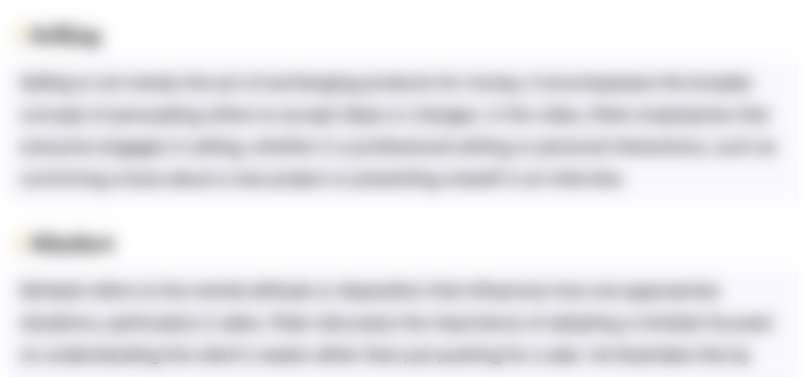
This section is available to paid users only. Please upgrade to access this part.
Upgrade NowHighlights
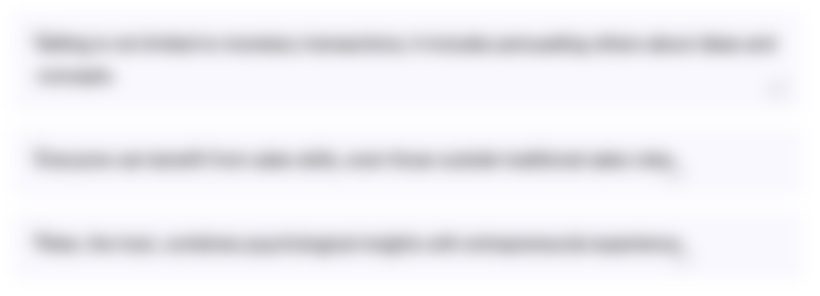
This section is available to paid users only. Please upgrade to access this part.
Upgrade NowTranscripts
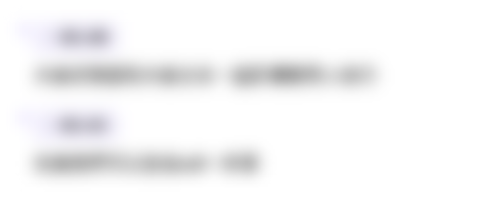
This section is available to paid users only. Please upgrade to access this part.
Upgrade NowBrowse More Related Video
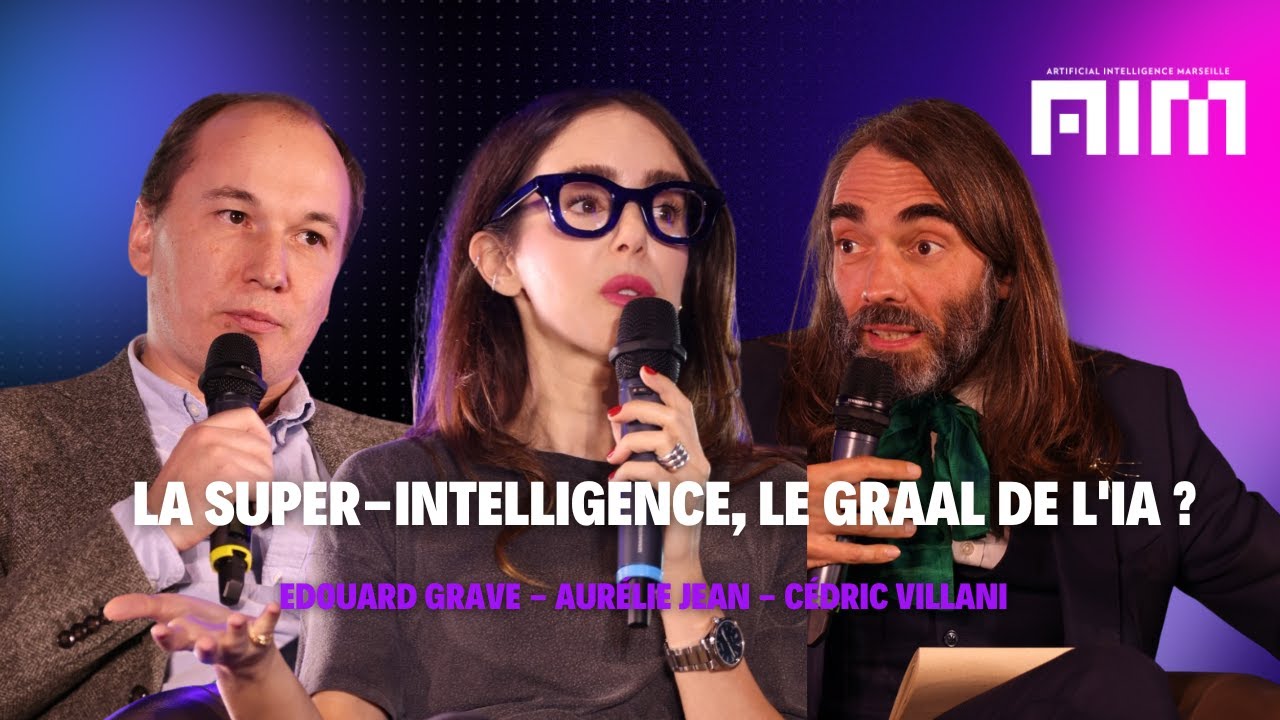
La super-intelligence, le Graal de l'IA ? | Artificial Intelligence Marseille
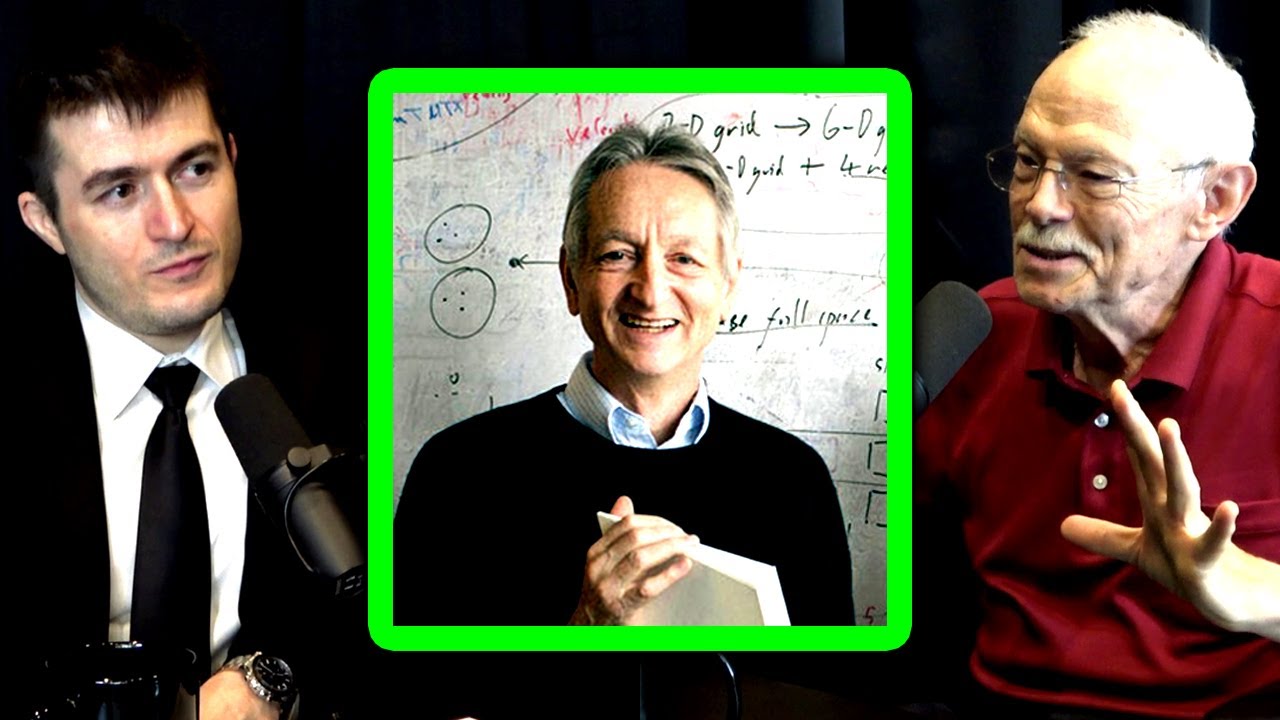
Geoffrey Hinton is a genius | Jay McClelland and Lex Fridman
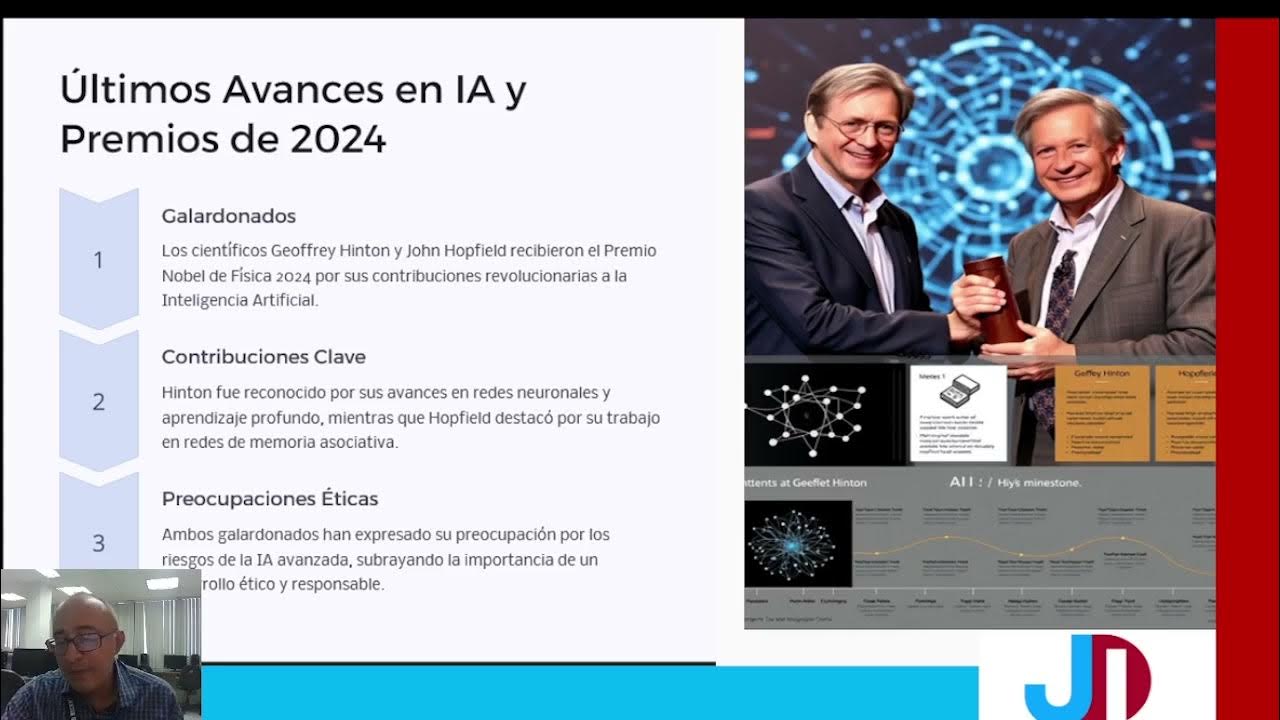
Inteligencia Artificial General (AGI) Y Supe inteligencia Artificial (ASI):Perspectivas y desafíos
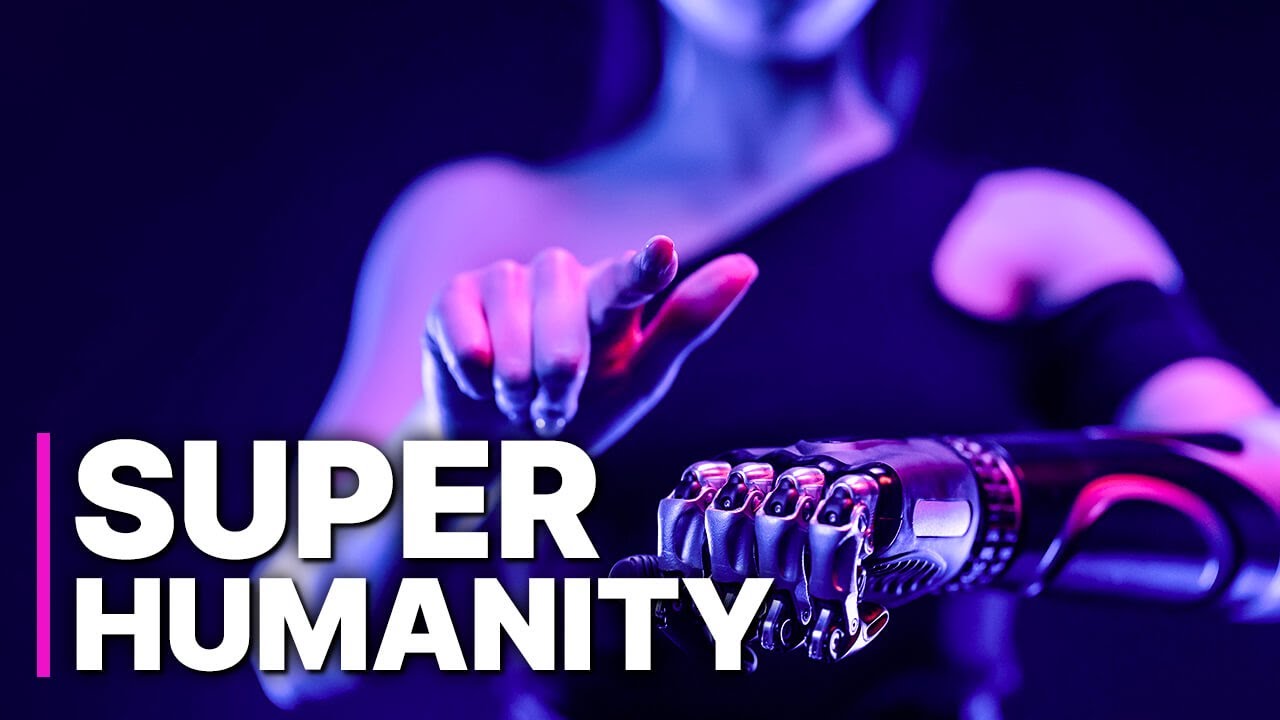
Super Humanity | Transhumanism
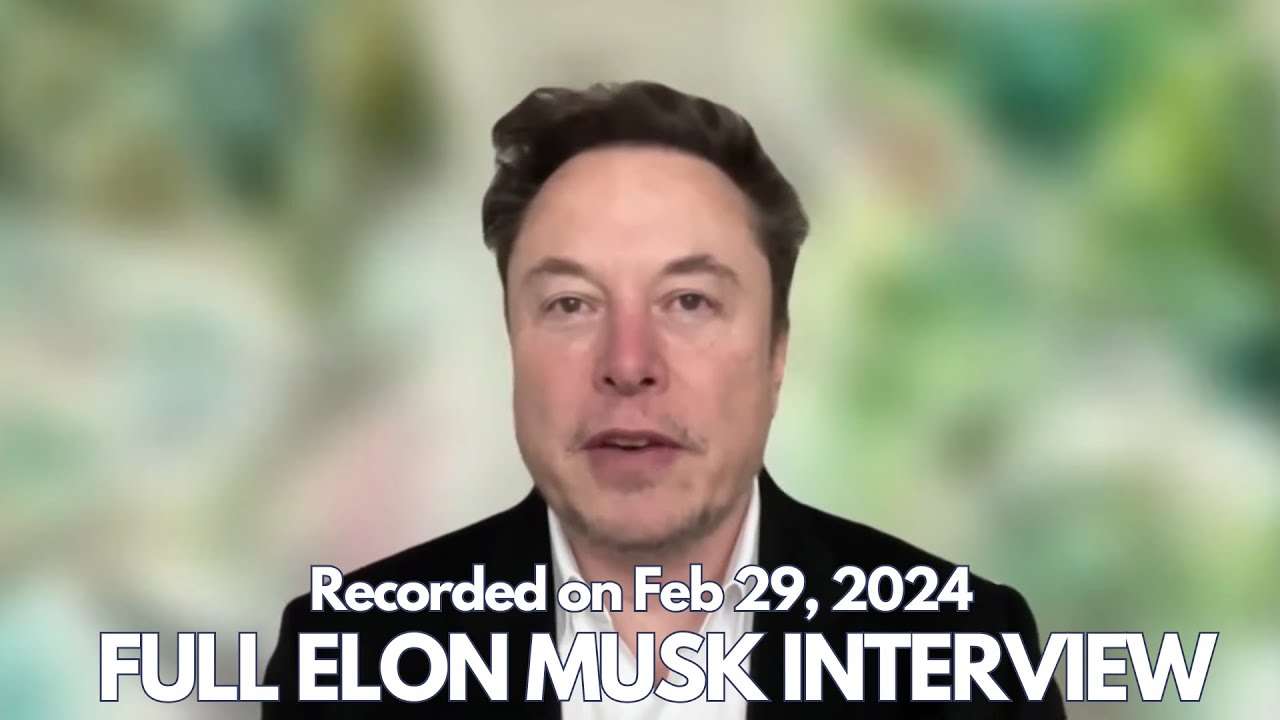
“I’ve NEVER seen anything like this” - Elon Musk drops a bombshell in Interview (Feb 29, 2024)
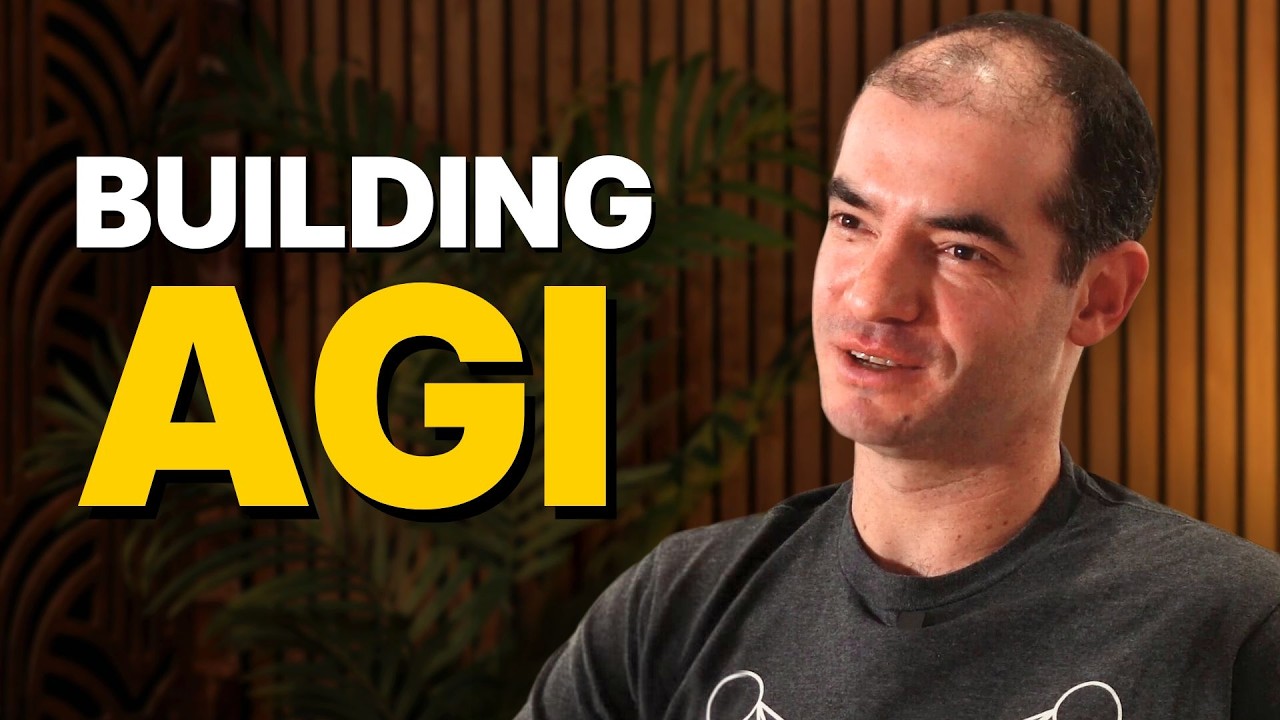
Ilya Sutskever (OpenAI Chief Scientist) - Building AGI, Alignment, Spies, Microsoft, & Enlightenment
5.0 / 5 (0 votes)