Hunting Crypto Trading Bots Using Volume Seasonality
Summary
TLDR本视频深入探讨了加密货币市场中成交量的季节性变化,重点分析了一周中不同日子和一天中不同时间的成交量行为。利用2018年1月1日至2022年10月的币安数据,演示了如何通过对原始成交量数据进行规范化处理来揭示其季节性特征。分析发现,工作日的成交量普遍高于周末,特别是UTC时间的12点到16点之间,市场活跃度最高。此外,每个常见时间框架的第一分钟成交量较高,这可能揭示了算法交易者的活动模式。视频最后提出了对这些发现的理论解释,并邀请观众提供反馈和讨论。
Takeaways
- 😀 The video analyzes cryptocurrency trading volume seasonality - how volume changes based on day of week and time of day
- 😎 Weekends have lower trading volume than weekdays, showing less activity from professional/institutional traders
- 🚀 Peak trading hours are roughly 12:00 - 16:00 UTC across different cryptocurrency pairs
- ⏰ The first minute of each hour/15 min/5 min period tends to have a spike in trading volume
- 🤔 This spike may be due to trading algorithms placing orders at bar close, which then execute in the next bar
- 😯 By analyzing volume spikes, you can detect consensus opinion of algorithmic traders
- 📈 The raw trading volume data needs to be normalized before analyzing seasonality
- 😴 A 30-day median is used to normalize the daily data
- 💤 A 168-hour (1-week) median is used to normalize the hourly data
- 🥱 A 10080-minute (1-week) median is used to normalize the minute-level data
Q & A
加密货币市场的成交量在一周中的哪些日子最低?
-在周末,即星期六和星期日,加密货币市场的成交量显著下降,低于工作日。
如何对原始成交量数据进行规范化处理以揭示季节性变化?
-通过取原始成交量与其30天滚动中位数的比值,然后对该比值取自然对数来规范化成交量数据。
为什么要对成交量数据进行规范化处理?
-因为原始未经处理的成交量数据不是稳定的,其行为随时间变化不一致,通过规范化处理可以使数据趋于稳定,便于分析。
加密货币市场在一天中的哪些小时成交量最高?
-从UTC时间的中午12点到下午4点(12:00-16:00)成交量最高,这一时段市场最为活跃。
周末与工作日的成交量有何不同?
-周末的成交量较低,表明周末的市场活动减少,这可能反映了机构活动在周末减少的趋势。
每小时成交量的规范化处理是如何进行的?
-通过使用168小时(一周)的滚动中位数来规范化每小时的成交量,以消除周末效应的影响。
在分钟级别的数据分析中,哪些时间点的成交量最高?
-在每小时的第一分钟成交量最高,此外,每15分钟和每5分钟的开始也会出现较高的成交量。
高频交易算法在市场成交量中扮演什么角色?
-高频交易算法在常见时间框架的第一分钟产生较高成交量,这表明大部分成交量可能来自于算法交易。
为什么在常见时间框架的第一分钟会看到成交量的高峰?
-这是因为交易系统通常会在每个时间段结束时分析数据,并在下一个时间段开始时立即下达市场订单,导致成交量激增。
如何利用第一分钟的成交量高峰来理解市场动态?
-如果第一分钟的成交量有明显方向,可以作为判断交易算法当前共识意见的一个指标。
Outlines
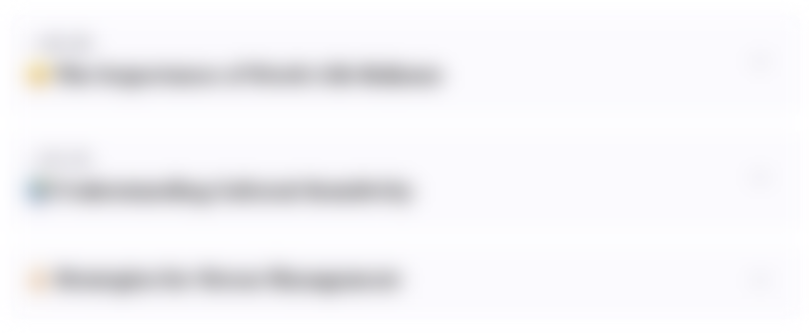
This section is available to paid users only. Please upgrade to access this part.
Upgrade NowMindmap
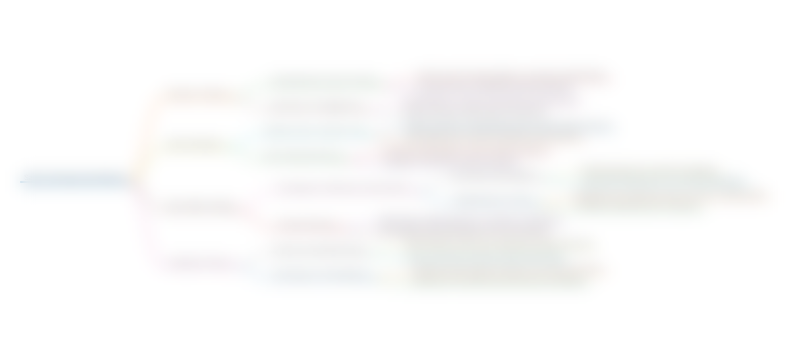
This section is available to paid users only. Please upgrade to access this part.
Upgrade NowKeywords
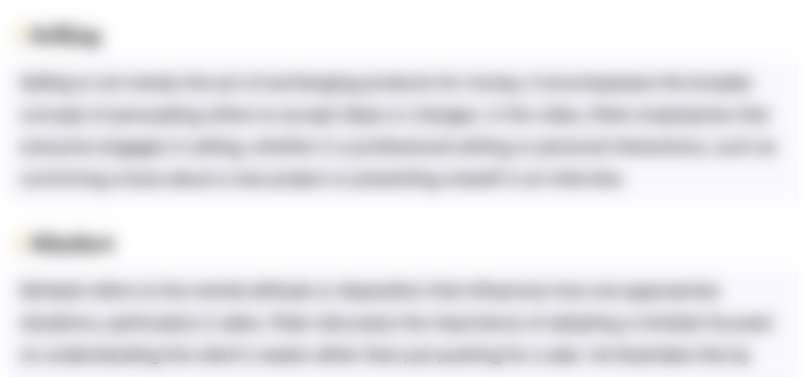
This section is available to paid users only. Please upgrade to access this part.
Upgrade NowHighlights
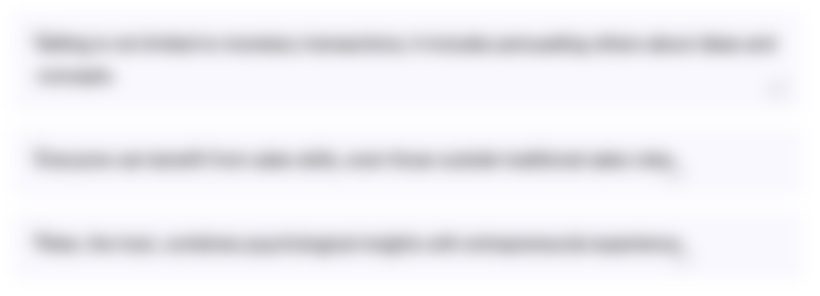
This section is available to paid users only. Please upgrade to access this part.
Upgrade NowTranscripts
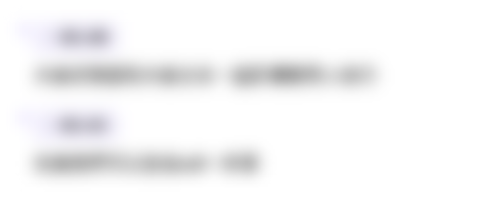
This section is available to paid users only. Please upgrade to access this part.
Upgrade NowBrowse More Related Video
5.0 / 5 (0 votes)