AI Radioroom Episode 1 | Exploring 0G Labs’ Solutions to Challenges in AI
Summary
TLDRIn this AI radio interview, Michael from ZeroG G Labs discusses the vision behind their modular AI chain, aiming to provide ultra-high performance and programmable data availability for decentralized storage networks. With a focus on AI, the project seeks to eliminate barriers between centralized and decentralized systems, ensuring transparency and efficiency, crucial for societal use cases like traffic control and manufacturing systems. The conversation also touches on the potential of on-chain AI, the importance of blockchain for societal trust, and Michael's personal journey from tech and business to co-founding ZeroG G Labs.
Takeaways
- 🚀 Michael from ZeroG G Labs is developing a modular AI chain aimed at high performance and programmable data availability and decentralized storage.
- 🌐 The project is inspired by the need for data throughputs in the tens of gigabytes per second to support onchain AI training, which is significantly higher than the current best-in-class throughputs.
- 🔍 The goal is to remove barriers between centralized and decentralized systems in terms of performance and cost, considering physical limitations like the speed of light.
- 💡 Michael's journey includes a background in deep tech, business, and finance, culminating in the establishment of a company focused on workplace well-being, which scaled to 650 people and $100 million in contract at ARR.
- 🤖 The co-founders of ZeroG G Labs are highly regarded computer scientists with whom Michael connected through a classmate, leading to the establishment of the company about a year ago.
- 🔑 The project's unique selling points are its performance, programmability, and AI focus, with a modular approach that allows for customization of data storage and security.
- 🔄 The technology is designed to be infinitely scalable, with plans to release a second phase of the main net that will allow horizontal scaling of consensus layers.
- 🛡️ Concerns about centralized control of AI models and the potential for misuse or mistraining are driving the need for transparent and verifiable AI systems, which blockchain can provide.
- 🔮 Michael believes that onchain AI is currently underhyped in the broader context but is gaining attention in the web 3 space, with societal use cases being particularly important.
- 🤖 Michael uses AI in his everyday life for tasks like writing answers for interviews, brainstorming, and automating tasks such as coding messages into a CRM system.
- 🌟 The average user would benefit from adopting a decentralized AI model for complete visibility, verifiability, and transparency, especially in use cases that require high trust and accountability.
Q & A
What is the main focus of ZeroG G Labs' project?
-ZeroG G Labs is focused on developing a modular AI chain that provides ultra-high performance, programmable data availability, and decentralized storage network, aiming to bridge the performance and cost barriers between centralized and decentralized systems.
What inspired Michael to start his venture in the AI and blockchain space?
-Michael was inspired by the need for a system that could handle data throughput at tens of gigabytes per second for onchain training and the desire to create an environment where AI models are transparent, verifiable, and controlled by decentralized entities.
What is the significance of the throughput capabilities of ZeroG G Labs' network?
-The high throughput capabilities, tens of gigabytes per second, are crucial for supporting advanced applications like onchain AI training, which requires significantly more data transfer rates than currently offered by most data availability networks.
How does ZeroG G Labs' project address the issue of centralized control in AI?
-By creating a decentralized storage network, ZeroG G Labs allows for open and transparent AI that is not controlled by centralized entities, ensuring that AI models can be monitored and verified for societal use cases.
What is the background of Michael that led him to his current project?
-Michael has a diverse background, having worked as an engineer and technical product manager at Microsoft and SAP Labs, consulted for Fortune 500 companies, worked in portfolio construction at Bridgewater Associates, and built a web 2 company at Stanford.
What was the turning point for Michael to dive into the Web 3 space?
-The turning point was in late 2022 when his classmate Thomas introduced him to the founders of Conflux, who wanted to start a more globally scaled project in Web 3, aligning with Michael's interest in blockchain since 2013.
What are the unique features of ZeroG G Labs' AI chain?
-The AI chain offers high performance, programmability, and an AI focus with modularity, allowing users to determine storage duration, location, data type, and security levels.
How does ZeroG G Labs plan to scale their consensus layers?
-They plan to horizontally scale consensus layers, making it possible to increase throughput by adding more servers, similar to adding an AWS server, which will be implemented in the pH two of the main net by the end of the year.
What are Michael's thoughts on the current hype around onchain AI?
-Michael believes that within Web 3, onchain AI is starting to get overhyped, but from a broader perspective, not enough people in Web 2 are discussing it, indicating that the technology is still underhyped in the larger context.
How does Michael envision the future use of onchain AI in everyday life?
-He sees onchain AI being used in various everyday scenarios, such as personal AI agents for tasks like writing responses, brainstorming, and automating processes like coding messages into a CRM system for better follow-ups.
What are the benefits for an average user to adopt a decentralized AI model over existing solutions?
-Decentralized AI models offer complete visibility, verifiability, and transparency, which is crucial for users who need to know exactly what data and context their AI is using, especially for sensitive applications like educational tools for children.
Outlines
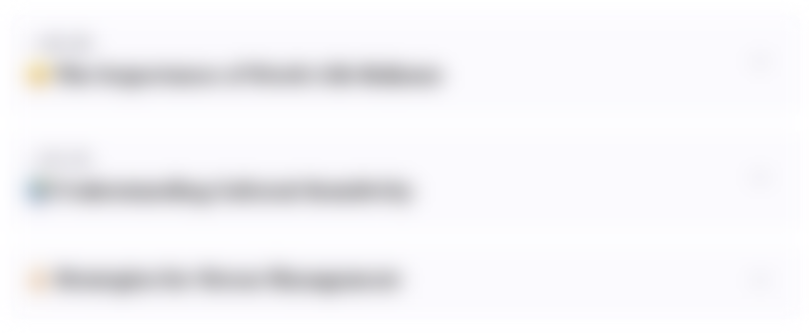
This section is available to paid users only. Please upgrade to access this part.
Upgrade NowMindmap
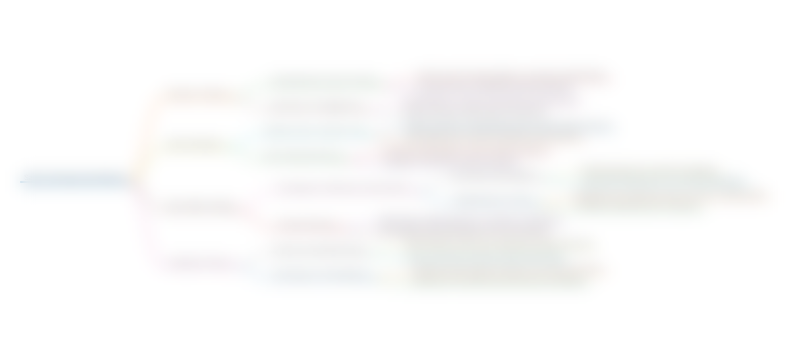
This section is available to paid users only. Please upgrade to access this part.
Upgrade NowKeywords
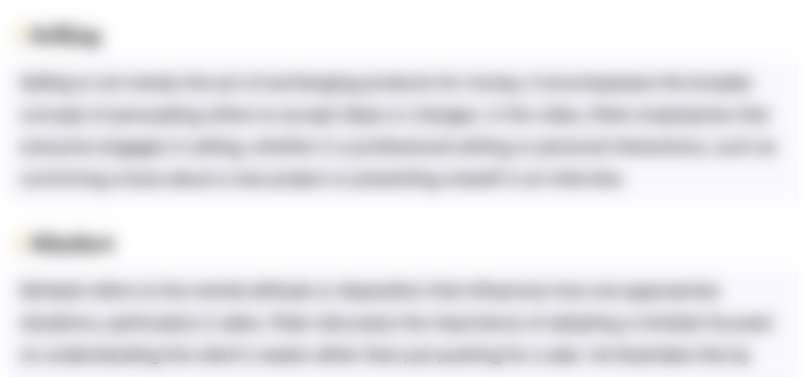
This section is available to paid users only. Please upgrade to access this part.
Upgrade NowHighlights
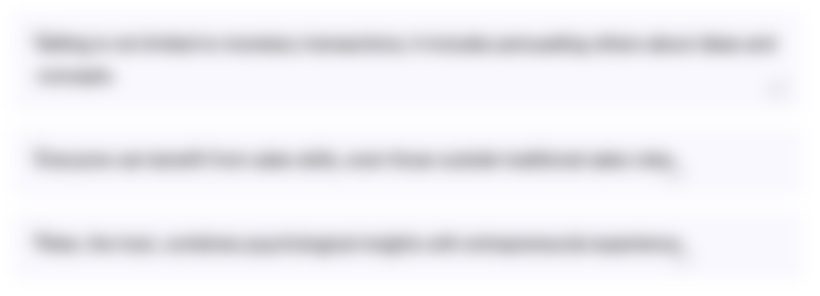
This section is available to paid users only. Please upgrade to access this part.
Upgrade NowTranscripts
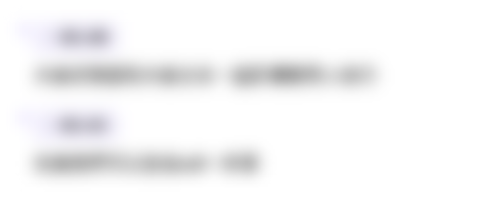
This section is available to paid users only. Please upgrade to access this part.
Upgrade NowBrowse More Related Video
5.0 / 5 (0 votes)