Toward a Deeper Understanding of Our Climate System Through Data Science | Emily Gordon
Summary
Please replace the link and try again.
Please replace the link and try again.
Please replace the link and try again.
Outlines
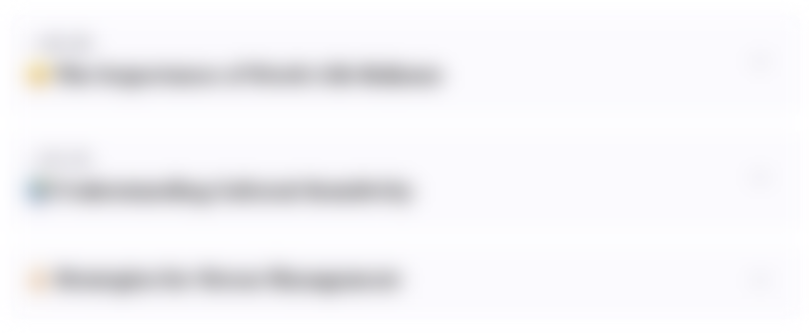
This section is available to paid users only. Please upgrade to access this part.
Upgrade NowMindmap
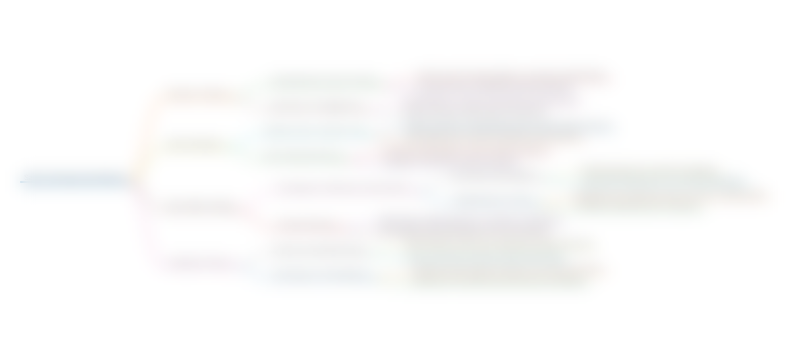
This section is available to paid users only. Please upgrade to access this part.
Upgrade NowKeywords
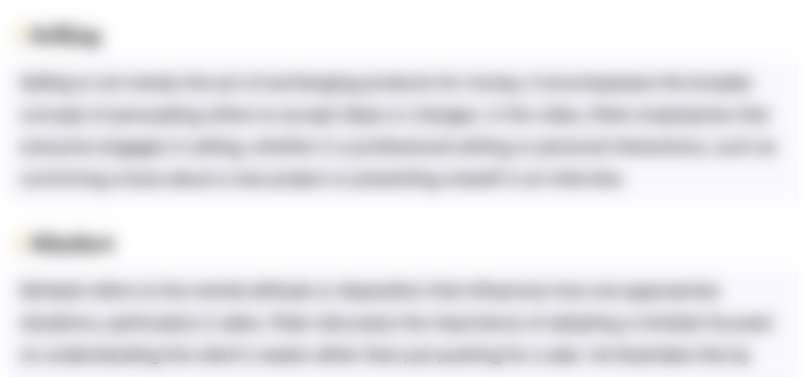
This section is available to paid users only. Please upgrade to access this part.
Upgrade NowHighlights
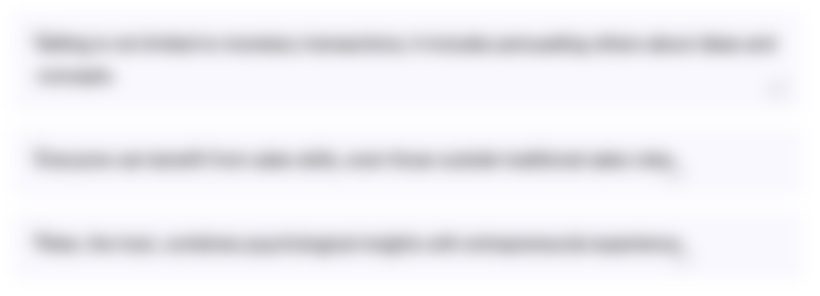
This section is available to paid users only. Please upgrade to access this part.
Upgrade NowTranscripts
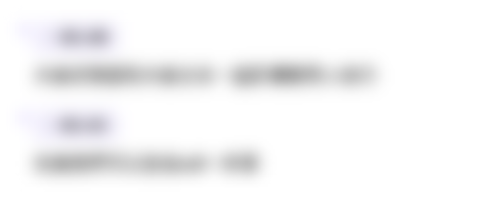
This section is available to paid users only. Please upgrade to access this part.
Upgrade NowBrowse More Related Video
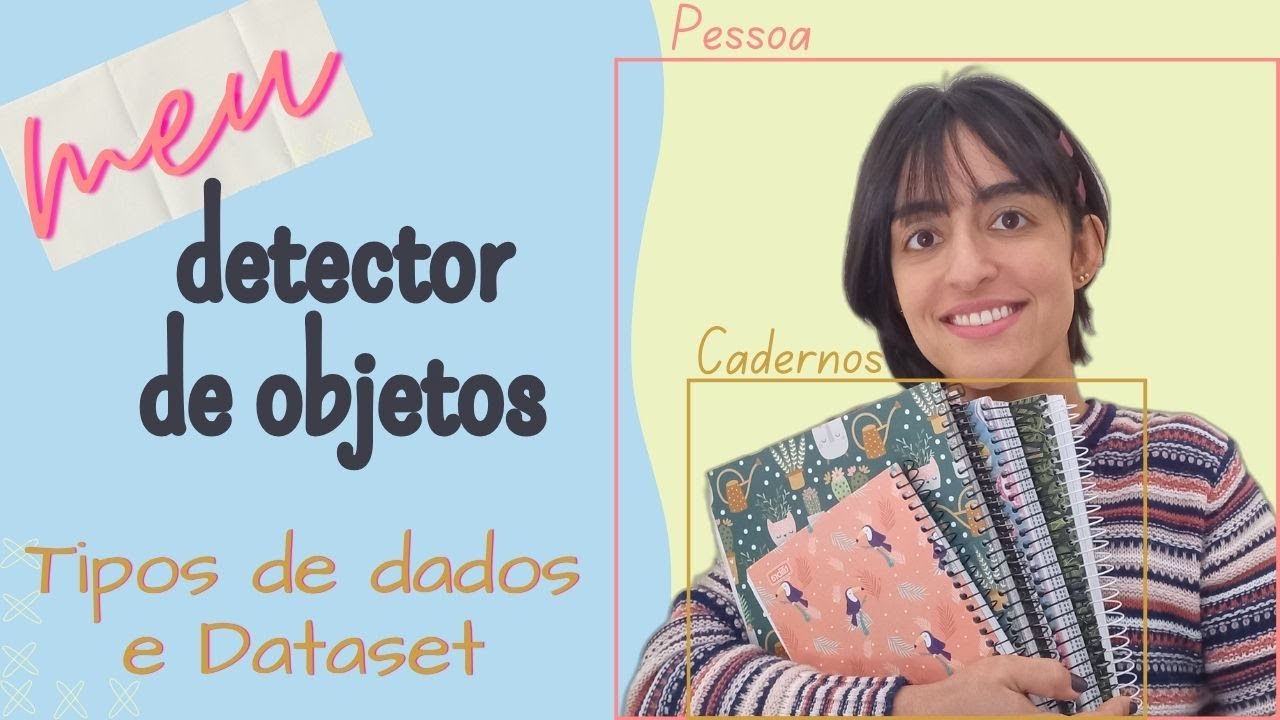
Aprendizado de Máquina (Machine Learning) | Entendendo Datasets e Tipos de Dados #1
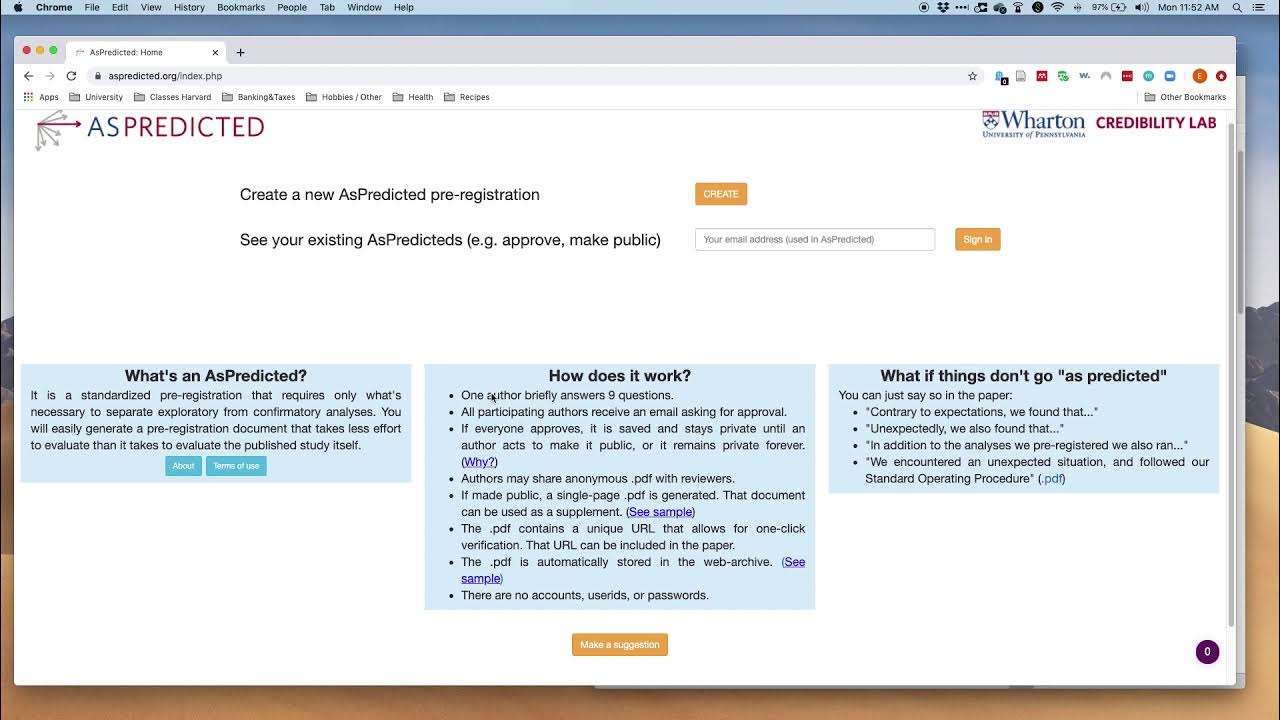
How to preregister a study on as predicted

The Problem With Food and Climate — and How To Fix It | Jonathan Foley | TED
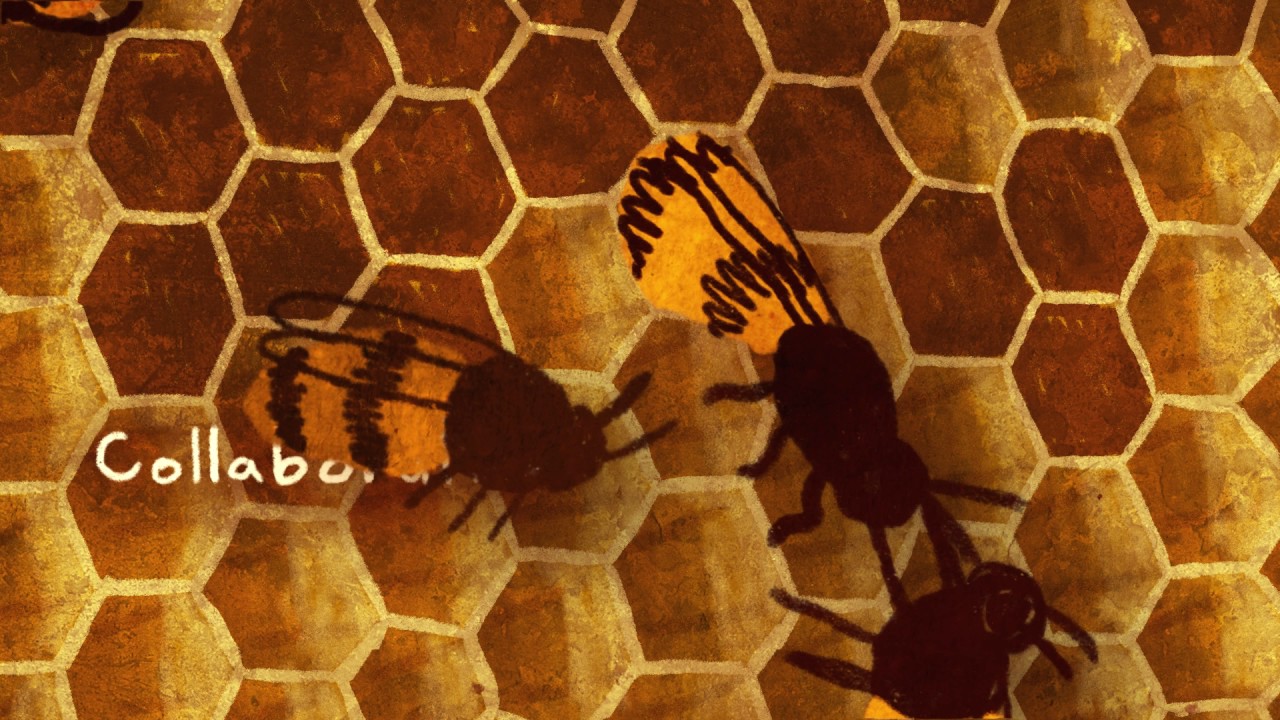
Citizen science - in researching biodiversity
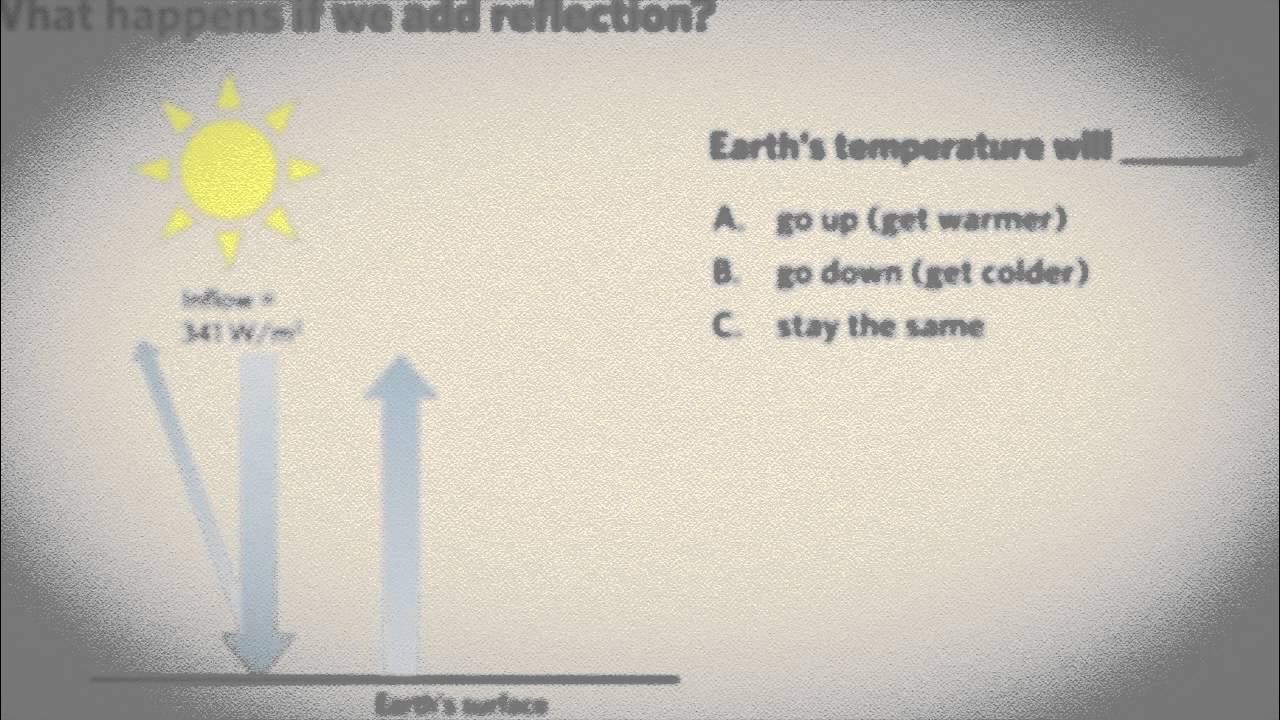
5.1 Introduction to Climate Modeling

NASA | Supercomputing the Climate
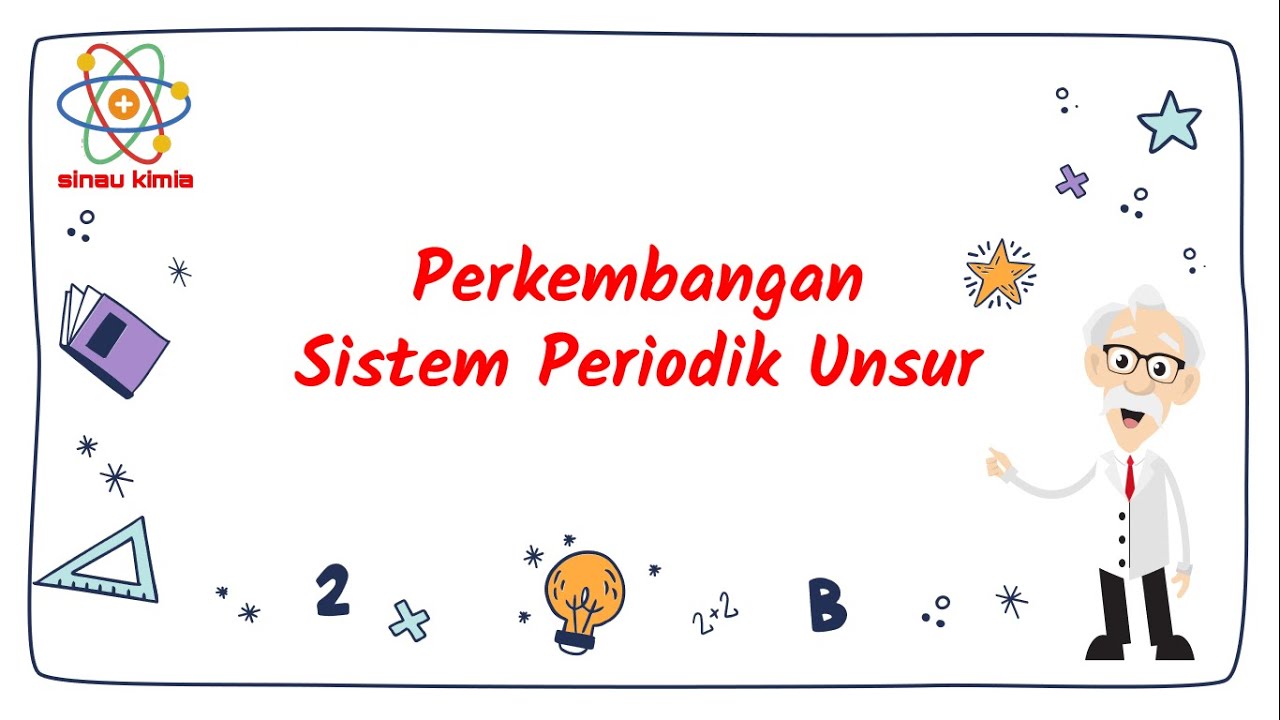
Perkembangan Sistem Periodik Unsur | KIMIA KELAS 10
5.0 / 5 (0 votes)