동영상이 이제 정말 자연스럽게 생성됩니다. Runway, Pika Lab, Stable Video Diffusion 모두 이겨버린 구글... 압도적 성능의 이유는 시공간 결합?
Summary
TLDRIn a recent announcement, Google Research unveiled a groundbreaking service in text-to-video and image-to-video generation, though not yet available for public use. This service, detailed in a comprehensive paper, showcases the ability to create highly realistic and diverse video content from images and text prompts, surpassing existing offerings from companies like PicApp and Runway. With a focus on Google's superior performance in video quality and text alignment, the video highlights the innovative use of space-time diffusion models for video generation. This advancement suggests potential future integrations into devices and platforms, such as the speculated inclusion in the next version of Gemini, indicating a significant leap forward in AI-driven video creation technology.
Takeaways
- 💻 Google Research announced a new text-to-video and image-to-video service, though not immediately available for public use, highlighting advancements in AI-generated content.
- 📸 The service can generate images in specific styles and even change certain parts of an image, indicating a high level of detail and customization in content creation.
- 🎥 Google's technology, referred to as Lumière, emphasizes generating videos with a comprehensive understanding of the video's context, allowing for more natural and story-based expressions.
- 📚 The approach uses a spacetime diffusion model for video generation, enhancing the ability to create realistic and contextually accurate videos from text or images.
- 🔍 Features include the ability to create naturally rotating objects and detailed scenarios, like an elephant walking underwater, showcasing the model's capacity to handle complex transformations and scenarios.
- 🔥 Google's AI model outperforms existing text-to-video and image-to-video models in terms of video quality and text alignment, setting new standards for the fidelity and accuracy of AI-generated content.
- 📌 The evaluation metrics used in their research (FVD, IS) indicate that Google's model produces videos closer to real-life quality, surpassing other models in realism and diversity.
- 🚀 The technology combines spacetime units with attention mechanisms to understand and generate video content more efficiently, highlighting a significant leap in handling video data.
- 🔧 Google's advancements suggest a future where AI can seamlessly restore or generate high-quality videos from partial or damaged inputs, revolutionizing video production and restoration.
- 📲 Discussions hint at the potential integration of such advanced video generation models into consumer devices and services, following trends in AI development and deployment.
Q & A
What new services did Google Research recently announce?
-Google Research announced new Text-to-Video, Image-to-Video, and Video-to-Video services using their new Lumier diffusion model.
What is unique about Lumier compared to other text-to-video models?
-Lumier uses a spatio-temporal diffusion model to generate more coherent videos with natural camera movement compared to other models that generate blurry or disjointed frames.
How does Lumier achieve higher video quality than other models?
-Lumier models the entire 5-second context of a video and generates frames conditioned on that context. This allows it to achieve higher video quality than models that generate frames independently.
How does Lumier outperform other models in benchmarks?
-Lumier achieves significantly better metrics like FVD and IS than baseline models, indicating it generates videos much closer to real ones and with higher quality and diversity.
What are some example use cases for Lumier?
-Lumier could be used to create realistic video effects and animations from text prompts or incomplete footage, such as filling in missing sections or changing attributes like costumes.
When will Lumier be available as a public service?
-Google has not announced plans to release Lumier publicly yet. It was published as a research paper to preview capabilities that may be productized in the future.
How might diffusion models impact synthetic media?
-Widespread diffusion models like Lumier could enable creation of highly realistic fake videos. This may increase risks of misuse, requiring new authentication methods.
What are the societal impacts of advancing text-to-video models?
-Text-to-video models like Lumier raise concerns about creation of misinformation and erosion of trust in video evidence. But they also enable new creative possibilities.
How might Lumier integrate with other Google services?
-Lumier could allow creation of custom video content in apps like YouTube, Google Docs, etc. But risks of misuse need to be managed.
What are the next steps in advancing text-to-video models?
-Researchers are focused on improving video coherence, resolution, and diversity while also developing responsible deployment practices to manage societal impacts.
Outlines
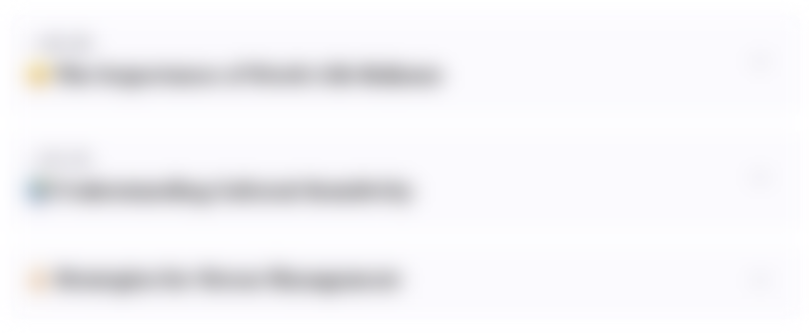
This section is available to paid users only. Please upgrade to access this part.
Upgrade NowMindmap
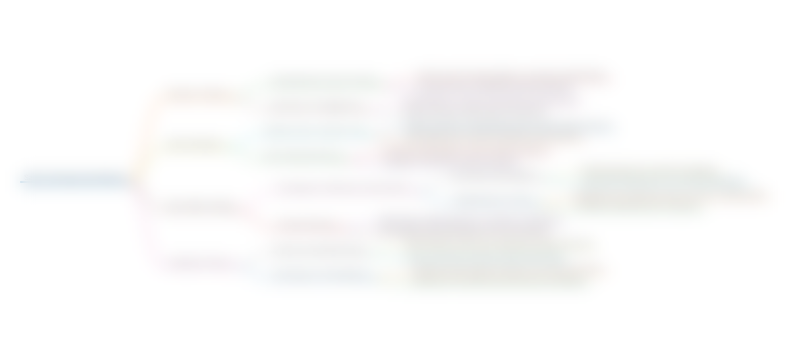
This section is available to paid users only. Please upgrade to access this part.
Upgrade NowKeywords
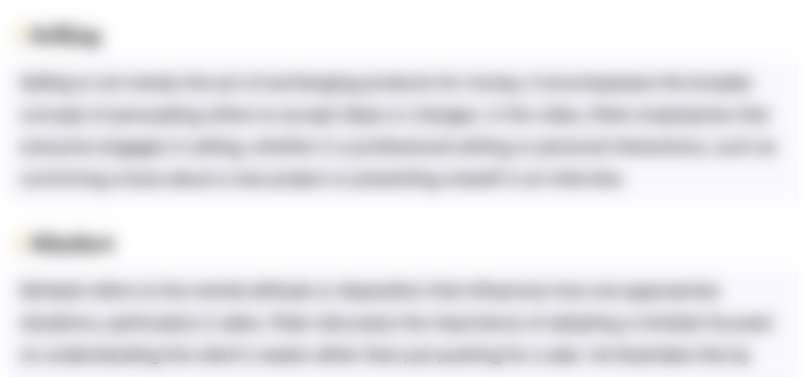
This section is available to paid users only. Please upgrade to access this part.
Upgrade NowHighlights
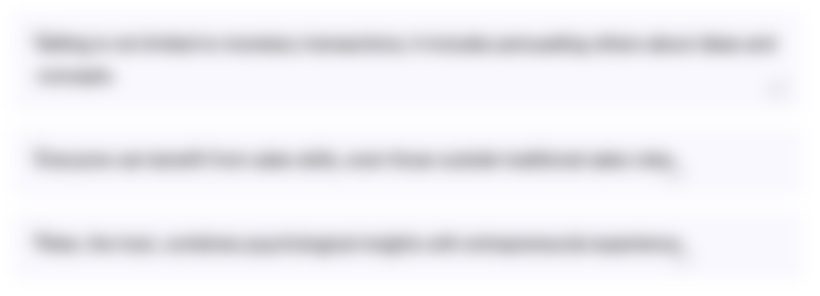
This section is available to paid users only. Please upgrade to access this part.
Upgrade NowTranscripts
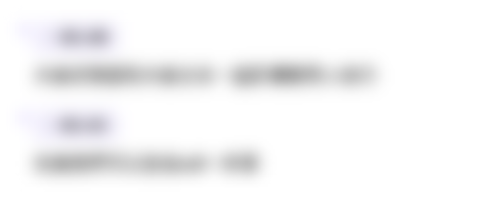
This section is available to paid users only. Please upgrade to access this part.
Upgrade NowBrowse More Related Video
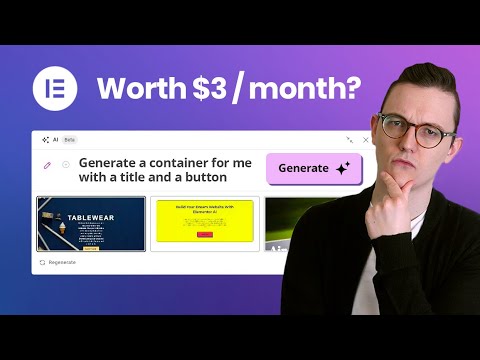
Elementor AI after 6 months. Is it worth it?
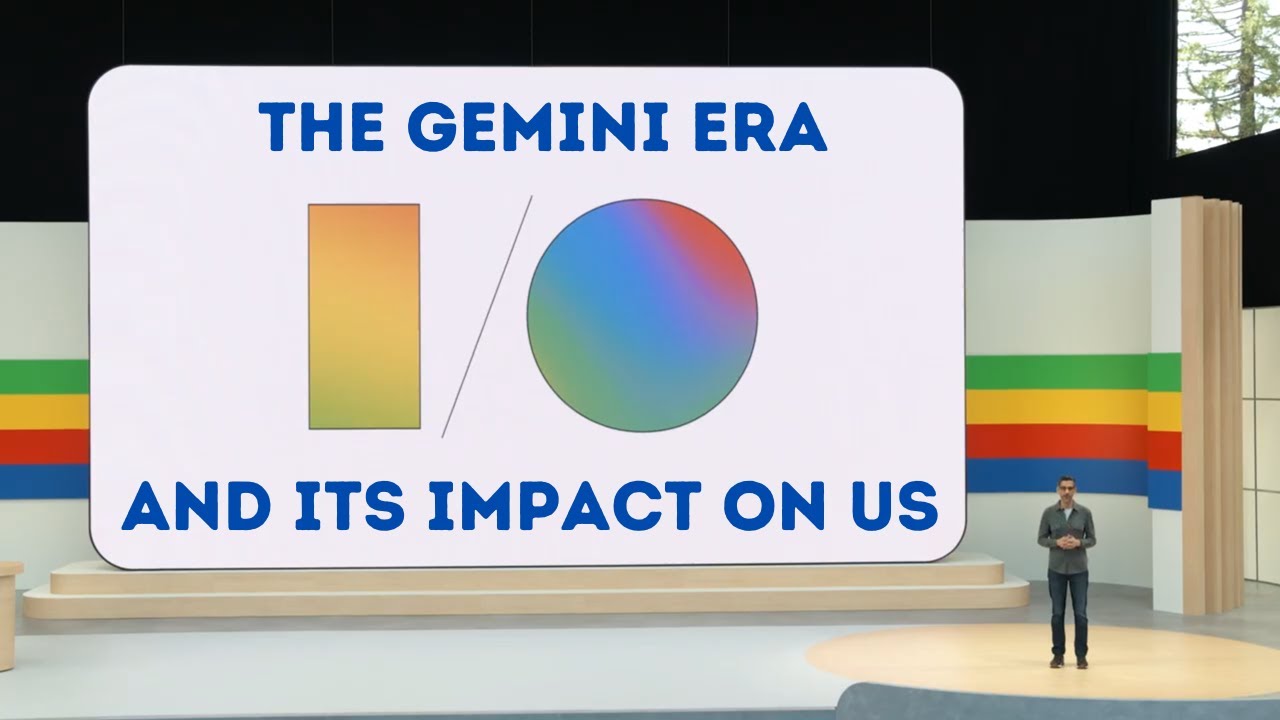
Google Keynote 2024 - The Gemini Era - And its Impact on Us - Google brings AI in our Daily Lives
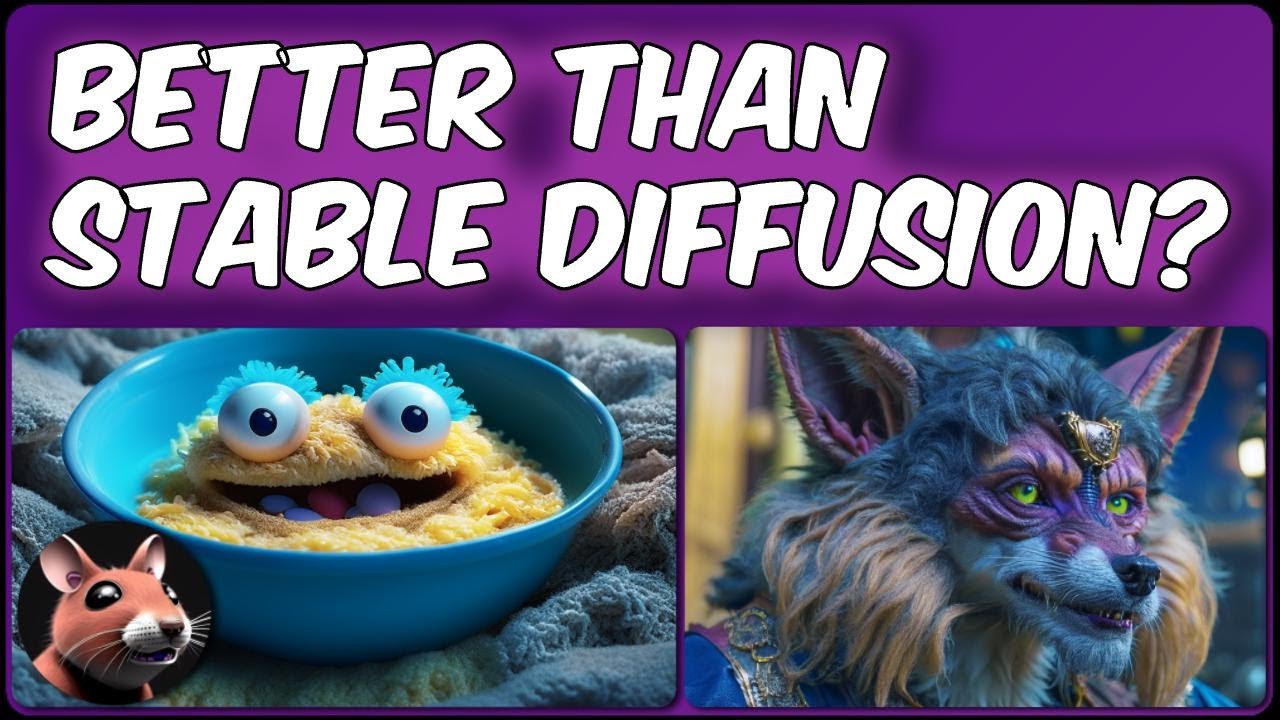
Is Kandinsky-2 is better than Stable Diffusion?

Master Any AI in 2025: Ultimate Comparison Guide (from ChatGPT to Gemini)
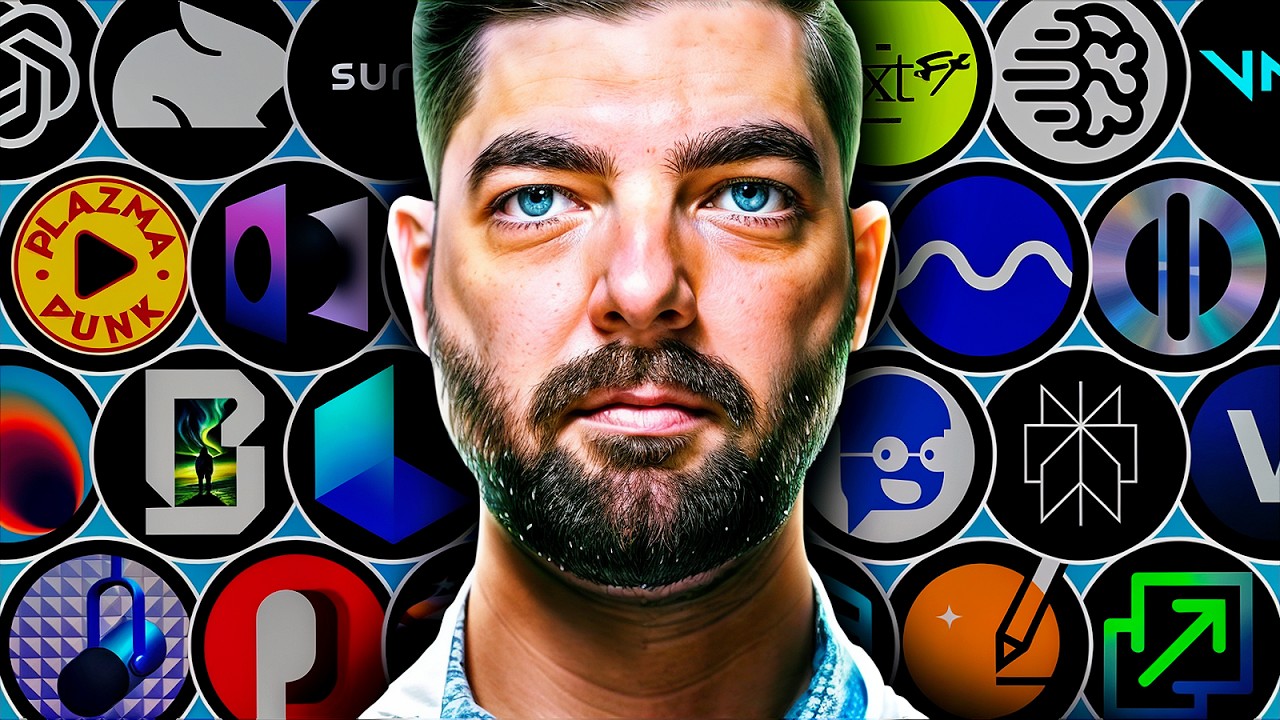
28 AI Tools You'll Be Shocked Are Free
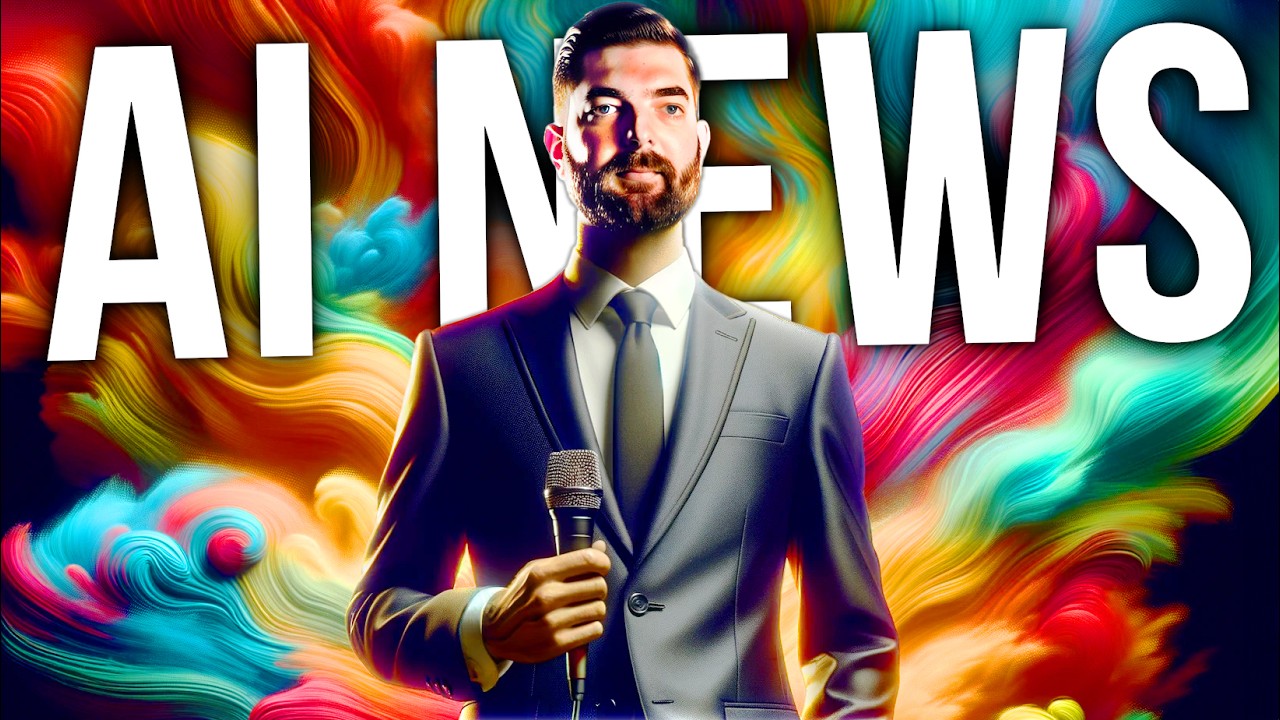
AI News: The Mind-Blowing World of AI Video
5.0 / 5 (0 votes)