Preventing Floods with Data Science - DSI + @GOTO2040
Summary
TLDRThe CMAP group developed a machine learning model to automate the identification and mapping of stormwater infrastructure across northeastern Illinois, aimed at supporting the Chicago Metropolitan Agency for Planning (CMAP). The project addresses climate change impacts, such as flooding, by improving urban drainage systems. Using aerial imagery, the team trained a segmentation model to classify features like ponds and wetlands. While successful in some areas, challenges remain with complex infrastructure like detention basins. Future work includes enhancing the model with additional data and improving accuracy for better urban planning.
Takeaways
- π The project aims to improve stormwater management in Chicago and surrounding areas to combat the impacts of climate change, such as flooding and reduced water quality.
- π Climate change is leading to extreme weather patterns in the Midwest, which increases the risk of flooding and environmental harm in the region.
- π The goal is to automate the identification and mapping of stormwater infrastructure, like ponds, wetlands, and detention basins, across northeastern Illinois.
- π The project partners with CMAP (Chicago Metropolitan Agency for Planning) to help plan and develop more efficient stormwater systems for future growth.
- π One of the challenges is the lack of a centralized inventory of stormwater infrastructure, especially for natural systems like wetlands, which makes it hard to identify and map them.
- π The group used aerial imagery from the USDA's National Agricultural Imagery Program to train a deep learning segmentation model to identify infrastructure in the images.
- π The segmentation model categorizes each pixel in an image, making it suitable for identifying the various types of stormwater infrastructure by drawing shapes around them.
- π The model showed good performance with the most prevalent infrastructure types, such as ponds and wetlands, but had difficulty distinguishing between ponds, lakes, and rivers.
- π Dry-bottom detention basins were challenging for the model to classify due to their varying appearance in different weather conditions, requiring additional data for more accurate identification.
- π Future improvements include adding more data, particularly elevation data, refining model parameters, and using pre-trained models tailored to aerial imagery for better results.
Q & A
What is the primary motivation behind the project?
-The primary motivation is to mitigate the effects of climate change in the Chicago area by ensuring that infrastructure development is accompanied by proper stormwater drainage systems to prevent flooding and other environmental issues.
Why is stormwater management a key concern for Chicago?
-Chicago faces increasing extreme weather patterns due to climate change, which leads to flooding, reduced water quality, and other environmental challenges. Additionally, rapid urban sprawl exacerbates the need for efficient stormwater systems to accommodate new developments.
What is the role of the Chicago Metropolitan Agency for Planning (CMAP) in this project?
-CMAP is a government organization tasked with watershed monitoring and future planning. The project aims to help CMAP by automating the identification and mapping of stormwater infrastructure, a task they previously struggled with due to a lack of comprehensive data.
What types of stormwater infrastructure are being targeted in the project?
-The project focuses on identifying and mapping four main types of infrastructure: ponds, wetlands, dry-bottom turf detention basins, and dry-bottom prairie detention basins.
What challenges did the team face in collecting and analyzing data?
-The team faced challenges with data wrangling due to different data formats, lack of a centralized stormwater infrastructure database, and difficulties with projection issues in point data. Additionally, visualizing and analyzing the data was complicated by its initial format designed for use in ArcGIS.
Why did the team choose a segmentation model for the project?
-A segmentation model was chosen because it is well-suited to identify and classify each pixel in an aerial image, allowing the model to map stormwater infrastructure types accurately based on shape data.
What performance issues did the model encounter?
-The model performed well in identifying ponds and wetlands but struggled with dry-bottom detention basins, which vary in appearance depending on environmental conditions. The model also had difficulty distinguishing between ponds, lakes, and rivers due to similar visual characteristics.
How does the Intersection over Union (IoU) metric relate to model performance?
-The IoU metric is used to measure the overlap between the model's predictions and the actual labeled areas in the data. Maximizing the IoU helps to assess the accuracy of the model in correctly identifying stormwater infrastructure.
What are the next steps for improving the model?
-Next steps include correcting data projection issues, obtaining higher resolution aerial imagery, incorporating elevation data to improve accuracy, and expanding the model to handle additional types of stormwater infrastructure. Reducing background-only images in training data will also help the model focus on actual infrastructure.
What is the significance of elevation data in improving the model?
-Elevation data can help the model distinguish features like dry-bottom detention basins, which are depressions in the landscape that capture rainwater. Including elevation data would allow the model to better identify these features based on their topographical characteristics.
Outlines
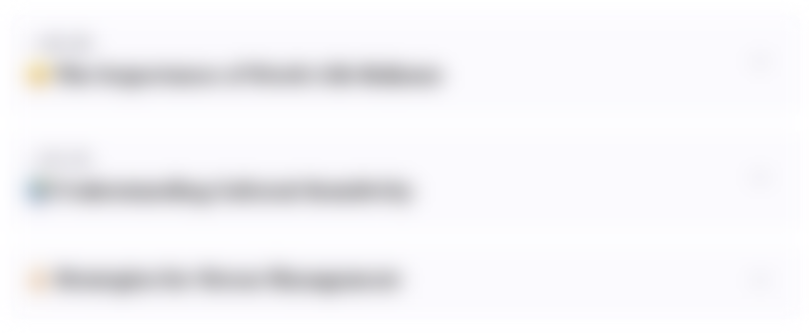
This section is available to paid users only. Please upgrade to access this part.
Upgrade NowMindmap
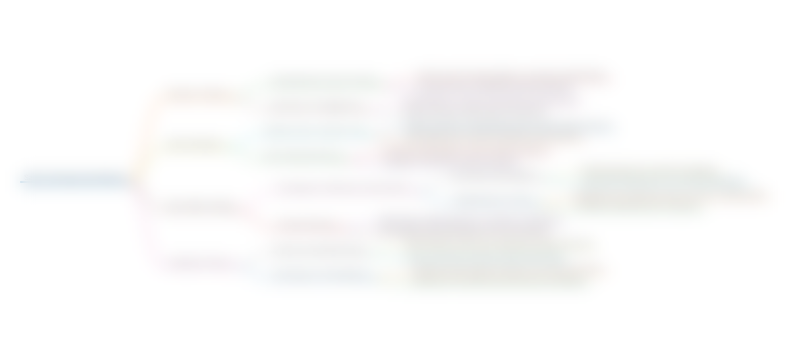
This section is available to paid users only. Please upgrade to access this part.
Upgrade NowKeywords
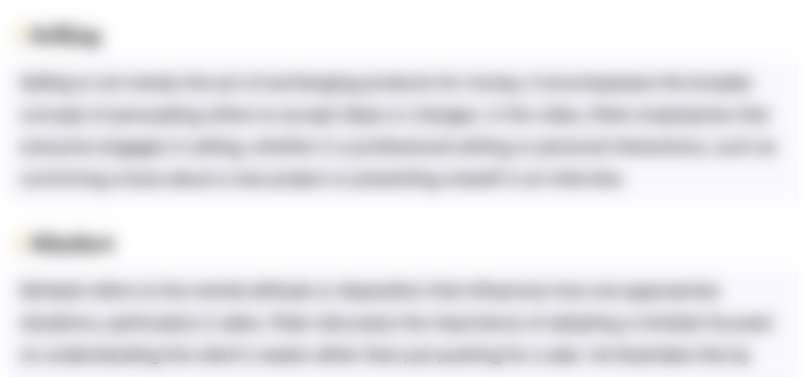
This section is available to paid users only. Please upgrade to access this part.
Upgrade NowHighlights
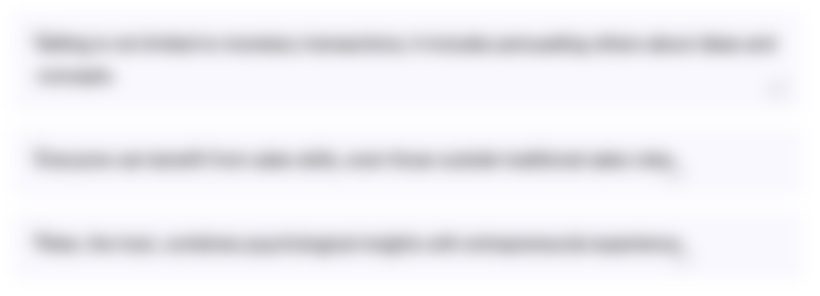
This section is available to paid users only. Please upgrade to access this part.
Upgrade NowTranscripts
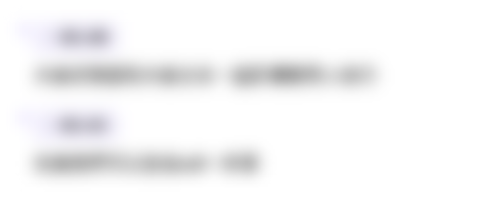
This section is available to paid users only. Please upgrade to access this part.
Upgrade NowBrowse More Related Video
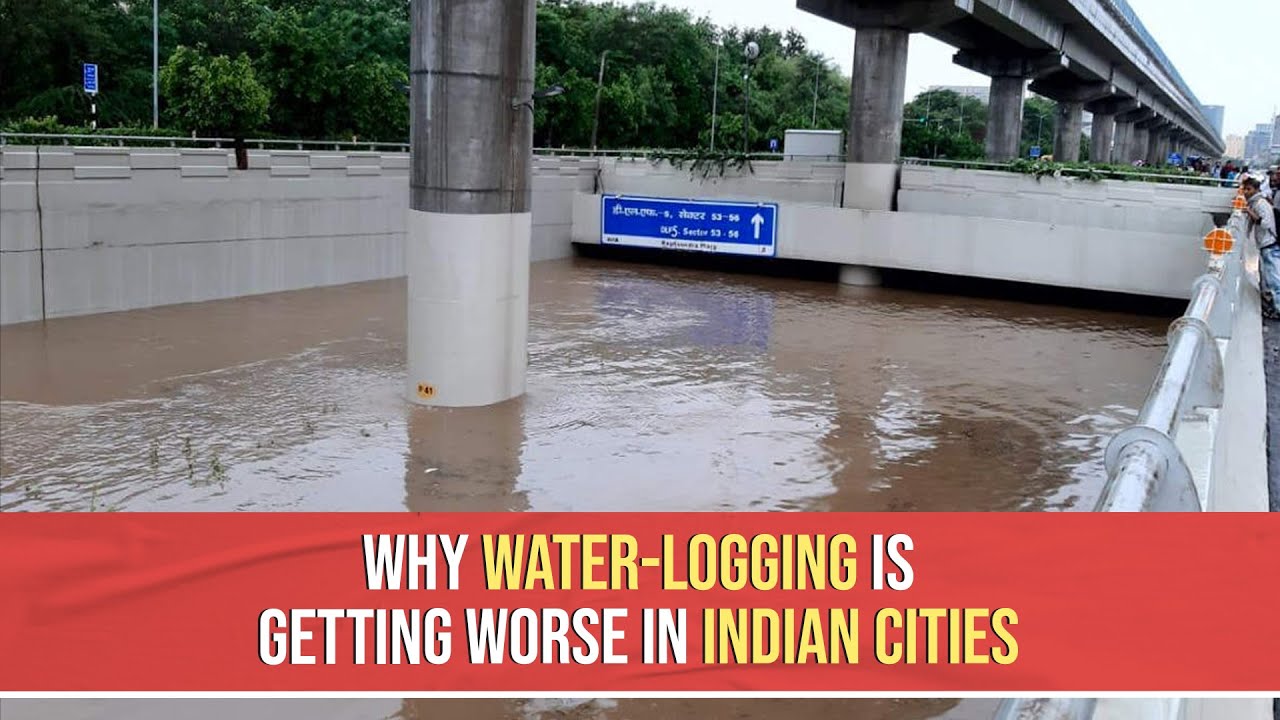
Why Water Logging Is Getting Worse In Indian Cities | IT Explains

Mumbai 3.0: The Future of Urban Planning | Shankar Deshpande | TEDxKESShroffCollege
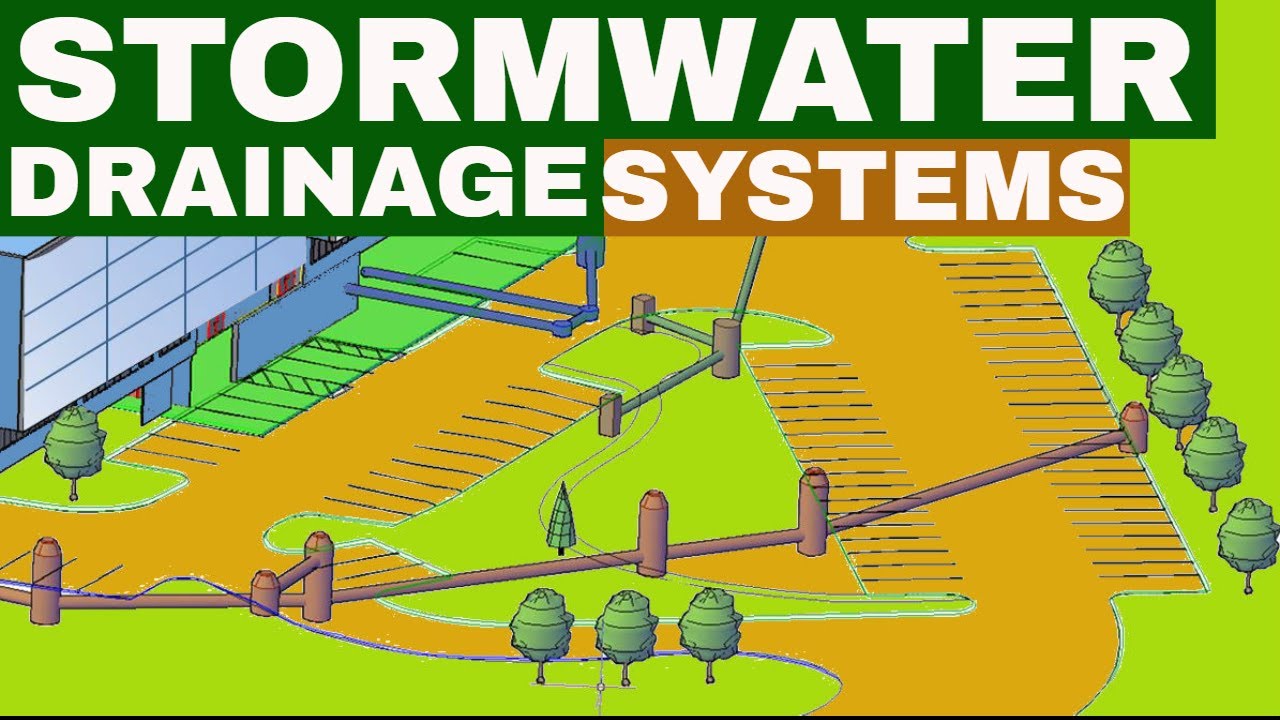
What is a Stormwater Drainage System? | Stormwater Drainage Design
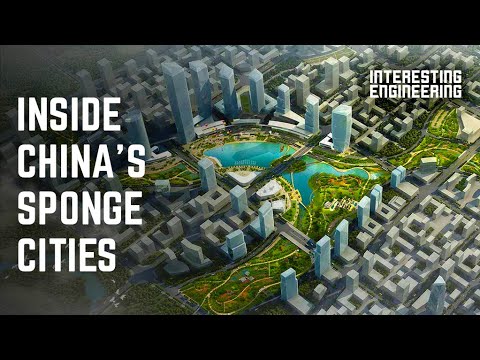
The genius of China's sponge cities
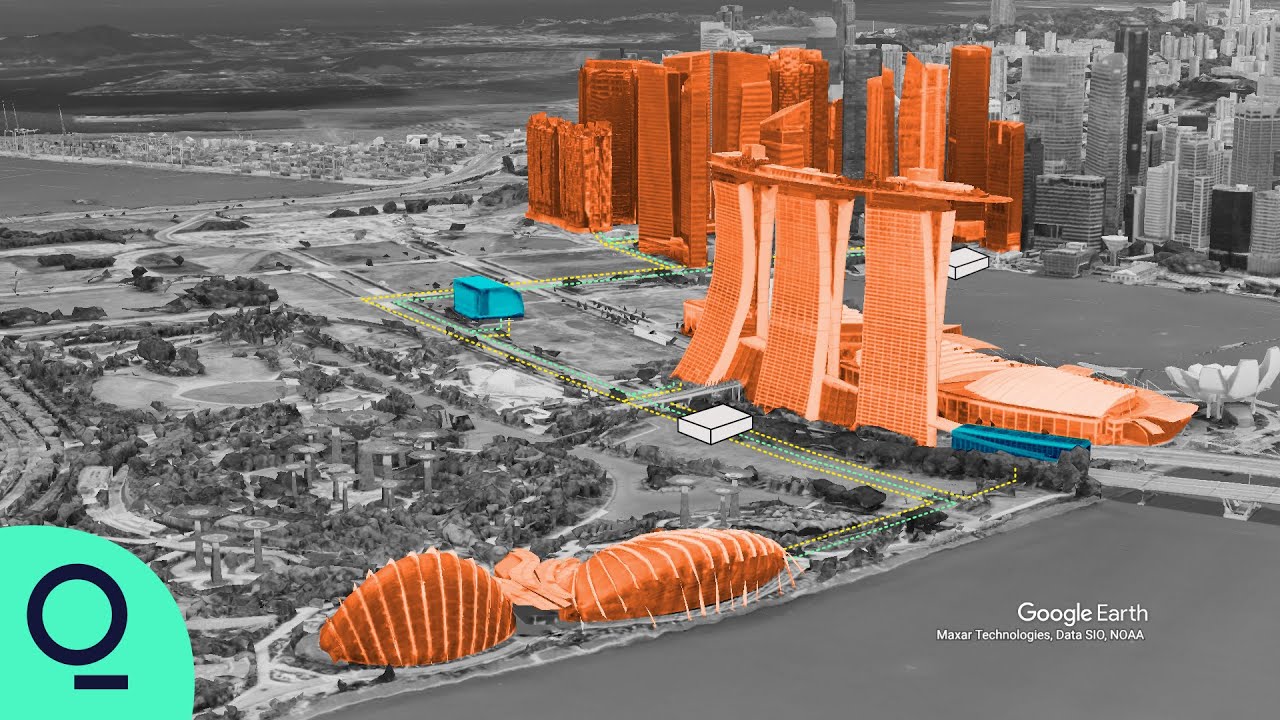
How Singapore Uses Science to Stay Cool

Building Resilient Cities: 7 Entry Points for Action
5.0 / 5 (0 votes)