Francois Chollet recommends this method to solve ARC-AGI
Summary
TLDRThe speaker discusses the promising integration of discrete program search and deep learning models to enhance automated reasoning (AR). They emphasize that while current program search methods are effective, they often struggle with combinatorial explosion. By combining these methods with a deep learning model that understands task intuition, future solutions could be significantly improved. This innovative approach, still largely unexplored, holds potential for generating high-quality solutions in the coming years, suggesting that the synergy between program search and intuitive AI could revolutionize problem-solving in this domain.
Takeaways
- 😀 Discrete program search is currently the most effective method for solving augmented reality (AR) tasks.
- 🤖 Large language models (LLMs) can develop a strong intuition for solving AR problems.
- 🔍 Combining program search with deep learning can enhance solution development.
- ⚙️ The process of program search often suffers from combinatorial explosion, complicating exploration of solution spaces.
- 💡 Deep learning models can guide the search process by providing insightful suggestions.
- 🧩 Using a planning model can help shape the sketch of potential solutions for AR tasks.
- 📈 There is significant untapped potential in integrating these approaches for AR solutions.
- 🔄 The next step involves leveraging LLMs to improve the efficiency of program searches.
- 🌟 Researchers expect to see high-quality solutions emerge from this integrated approach in the near future.
- 🚀 This domain is ripe for innovation, with many opportunities for breakthroughs in AR problem-solving.
Q & A
What is the main focus of the speaker's discussion?
-The speaker discusses integrating program search techniques with deep planning driven by intuition to solve tasks more effectively.
What is program search, and why is it significant?
-Program search is a method for exploring a massive program space to find solutions. It's significant because it has proven to be effective for certain tasks, particularly in augmented reality (AR).
What are the limitations of traditional program search?
-Traditional program search often involves naive exploration of options, leading to combinatorial explosion, which limits the effectiveness of finding solutions.
How can deep learning enhance program search?
-Deep learning can provide a planning model that intuitively suggests promising paths to explore in the program space, making the search process more efficient.
What does the speaker mean by 'combinatorial explosion'?
-Combinatorial explosion refers to the rapid increase in possible combinations or configurations that can occur as the size of the problem grows, making it difficult to explore all options.
What is the expected outcome of combining program search with deep learning?
-The expected outcome is the generation of higher quality solutions in AR tasks, leveraging the strengths of both methods.
What does the speaker mean by 'low-hanging fruit' in this context?
-'Low-hanging fruit' refers to easily achievable solutions or opportunities that have not yet been fully explored in the integration of program search and deep learning.
Why does the speaker believe there is potential in this area?
-The speaker believes there is potential because few people have tried combining these approaches, indicating many unexplored avenues for high-quality solutions.
What is the role of intuition in solving AR tasks according to the speaker?
-Intuition helps guide the program search by providing suggestions on what to try next, based on a developed understanding of what a solution should look like.
How does the speaker see the future of solutions in this domain?
-The speaker sees a promising future where integrating program search with deep learning will lead to the highest quality solutions in the coming years.
Outlines
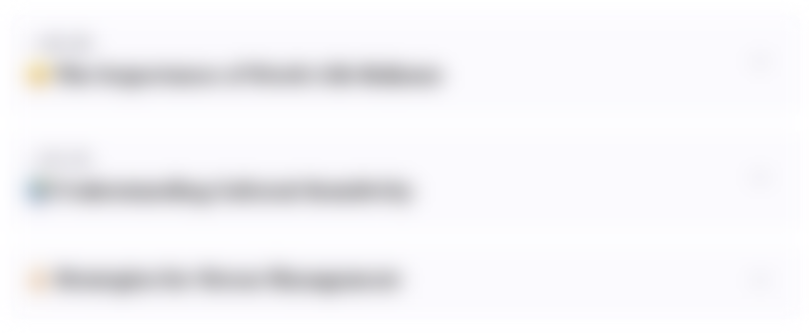
This section is available to paid users only. Please upgrade to access this part.
Upgrade NowMindmap
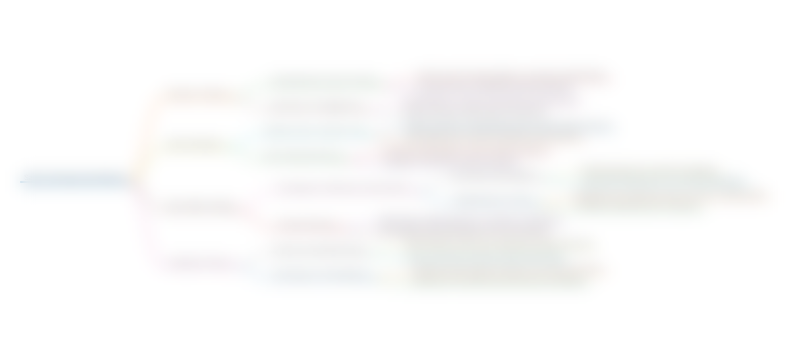
This section is available to paid users only. Please upgrade to access this part.
Upgrade NowKeywords
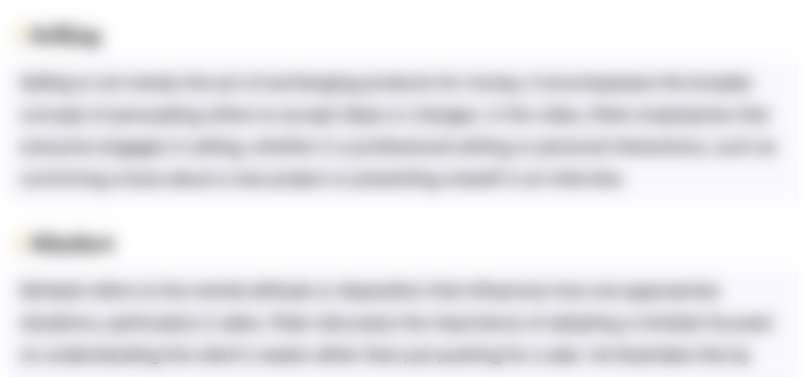
This section is available to paid users only. Please upgrade to access this part.
Upgrade NowHighlights
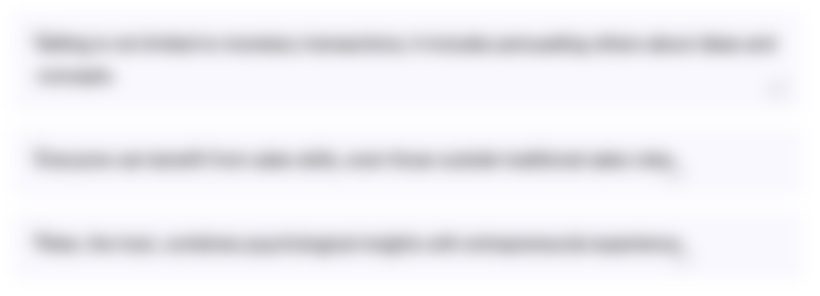
This section is available to paid users only. Please upgrade to access this part.
Upgrade NowTranscripts
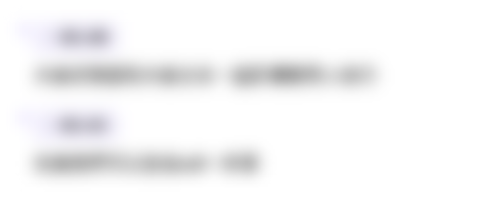
This section is available to paid users only. Please upgrade to access this part.
Upgrade Now5.0 / 5 (0 votes)