Productionizing GenAI Models – Lessons from the world's best AI teams: Lukas Biewald
Summary
TLDRThe discussion emphasizes the critical need for robust evaluation frameworks in generative AI applications to ensure effective model performance and decision-making. The speaker highlights the pitfalls of informal assessments and the importance of incorporating user feedback throughout the development process. By starting with lightweight prototypes and implementing diverse evaluation techniques, organizations can better correlate performance metrics with user experience. The talk underscores that systematic evaluation is essential for improving AI applications, inviting attendees to engage with the speaker's organization for further insights and solutions in deploying AI successfully.
Takeaways
- 😀 Effective evaluation frameworks are crucial for the successful deployment of generative AI models.
- 🤖 Relying on 'vibes' for model assessment can lead to poor decision-making and confusion over performance metrics.
- 🔍 A robust evaluation system allows teams to differentiate between various model accuracies and performance levels.
- 🛠️ Starting with lightweight prototypes and incorporating user feedback is essential for agile development in AI.
- 📊 Different evaluation techniques are necessary to ensure comprehensive assessment across all aspects of an AI application.
- 🏆 Correlating metrics with user experience is a significant challenge but vital for understanding model performance.
- 📈 Many organizations track thousands of metrics to gain insights into their models, highlighting the complexity of AI evaluation.
- 🤔 Questions about model fine-tuning often indicate a lack of established evaluation frameworks within teams.
- 👥 Engaging end-users early in the development process can enhance the relevance and effectiveness of AI applications.
- 🎉 Collaboration and sharing experiences at conferences can foster learning and improvement in AI practices.
Q & A
What is the primary focus of the discussion in the video?
-The primary focus is on the importance of establishing robust evaluation frameworks for generative AI models to ensure their effective deployment and continuous improvement.
What problem arises from relying solely on 'vibes checks' for decision-making in AI model development?
-'Vibes checks' do not provide quantitative measures of performance, making it difficult to determine the effectiveness of different models and leading to potentially poor decision-making.
What did the speaker learn from the CEO regarding model evaluation a year after their initial discussion?
-The CEO recognized the necessity of comprehensive evaluation practices to facilitate transitions between models and improve overall decision-making.
What common pitfall does the speaker identify in enterprise product development?
-The speaker identifies a tendency for organizations to focus on perfecting initial steps instead of releasing early prototypes to users, which hinders timely feedback and iterative development.
How does the speaker suggest organizations incorporate end-user feedback?
-The speaker emphasizes the need to gather and integrate user feedback in various forms to inform the iterative development of AI applications.
What types of evaluation techniques do organizations typically implement according to the speaker?
-Organizations implement a range of evaluation techniques, including simple tests for critical accuracy, quick real-time tests, and extensive nightly evaluations for comprehensive feedback.
Why is it challenging to align metrics with user experience in AI applications?
-Aligning metrics with user experience is difficult due to the complexity of user interactions and the diverse ways an AI application can fail, making it hard to track the most relevant performance indicators.
What metric tracking behavior do organizations exhibit in high-stakes applications like self-driving cars?
-In high-stakes applications, organizations often track thousands or even tens of thousands of metrics to ensure comprehensive oversight of model performance and user experience.
What does the speaker imply about the evaluation capabilities of many attendees?
-The speaker suggests that many attendees feel their evaluation frameworks are inadequate, indicating a widespread challenge in effectively assessing generative AI applications.
What opportunity does the speaker provide for attendees interested in improving their AI applications?
-The speaker invites attendees to visit their booth for discussions on how to enhance their AI application evaluation processes and share their experiences.
Outlines
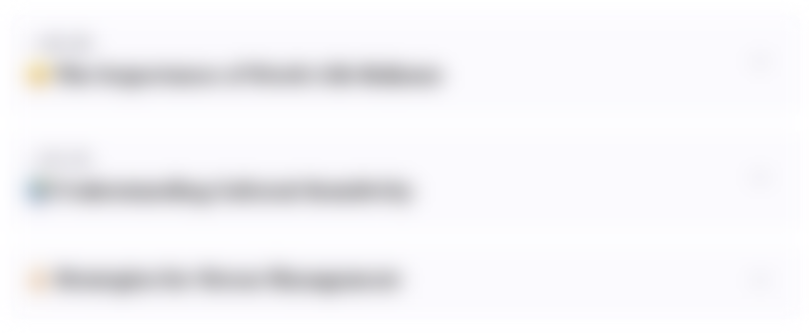
This section is available to paid users only. Please upgrade to access this part.
Upgrade NowMindmap
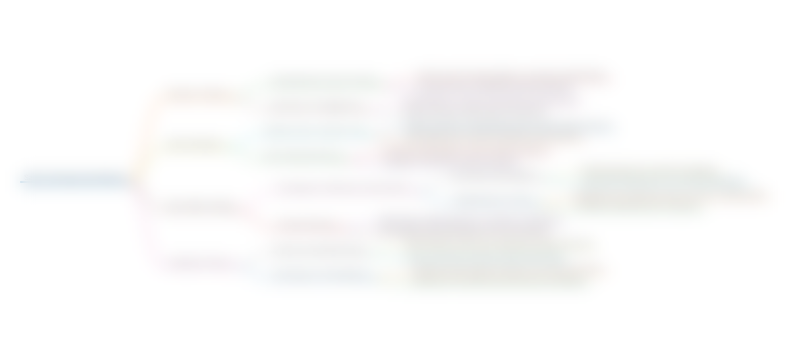
This section is available to paid users only. Please upgrade to access this part.
Upgrade NowKeywords
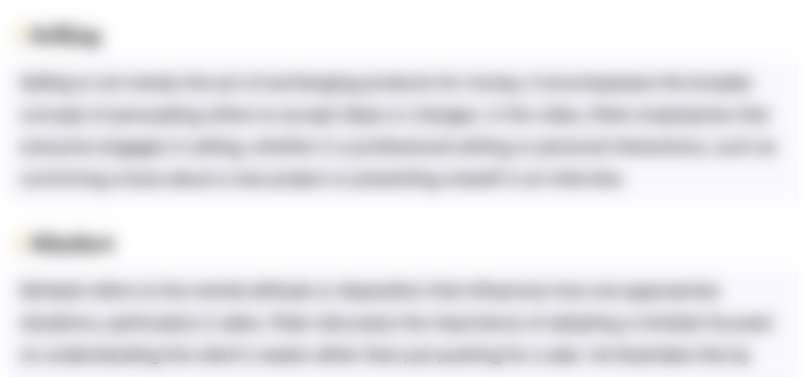
This section is available to paid users only. Please upgrade to access this part.
Upgrade NowHighlights
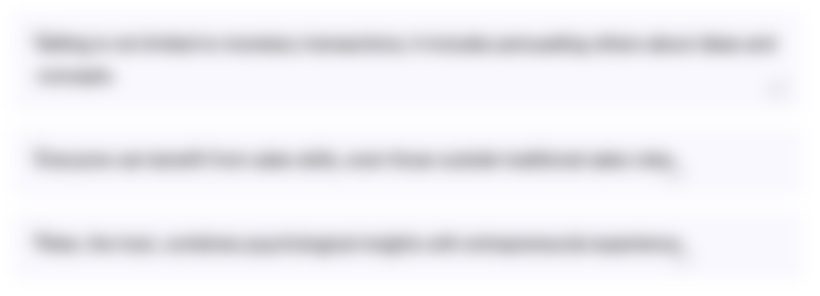
This section is available to paid users only. Please upgrade to access this part.
Upgrade NowTranscripts
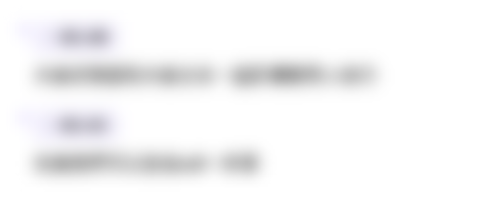
This section is available to paid users only. Please upgrade to access this part.
Upgrade NowBrowse More Related Video
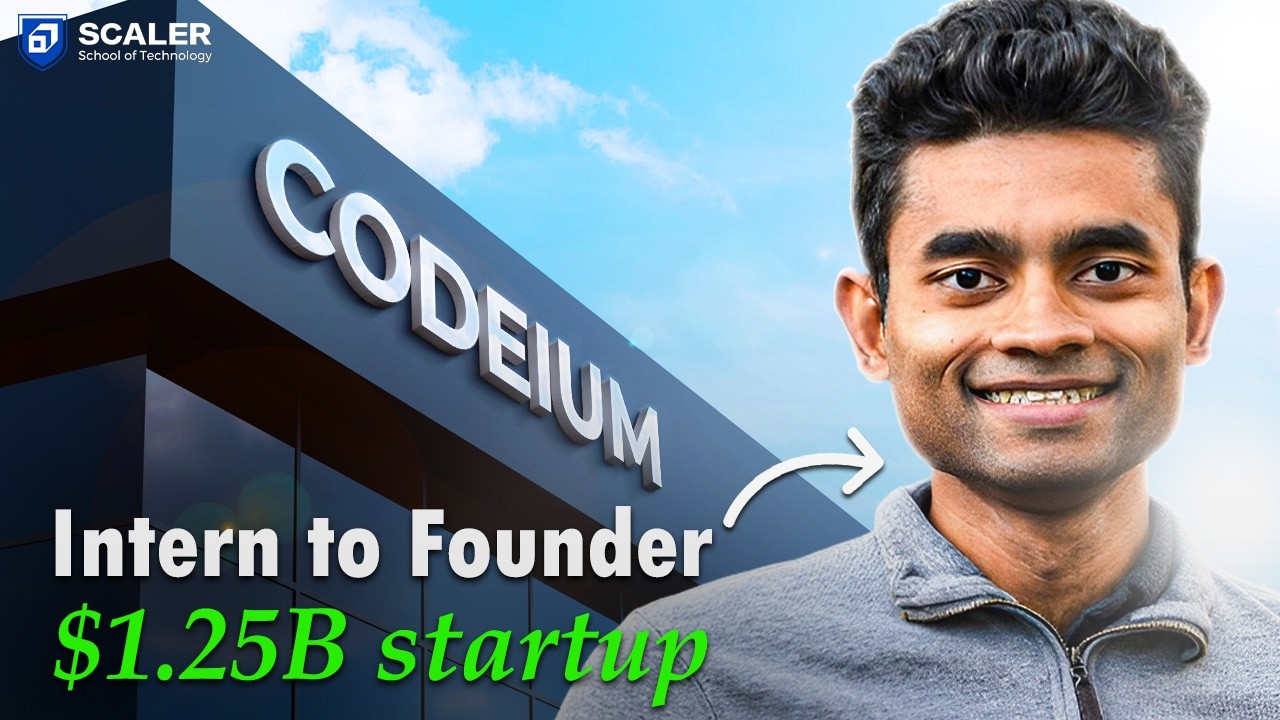
How An Internship Led To Billion Dollar AI Startup
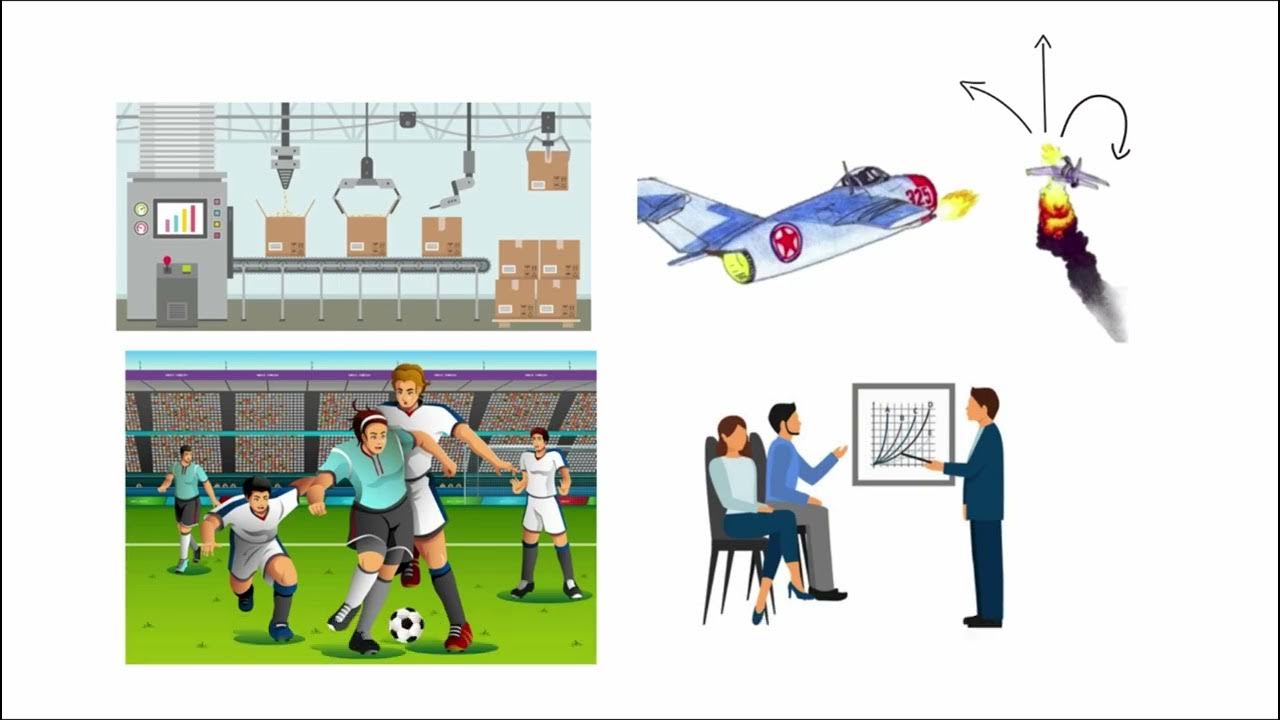
Penjelasan Model AOPL

AWS re:Invent 2023 - Transforming the consumer packaged goods industry with generative AI (CPG203)
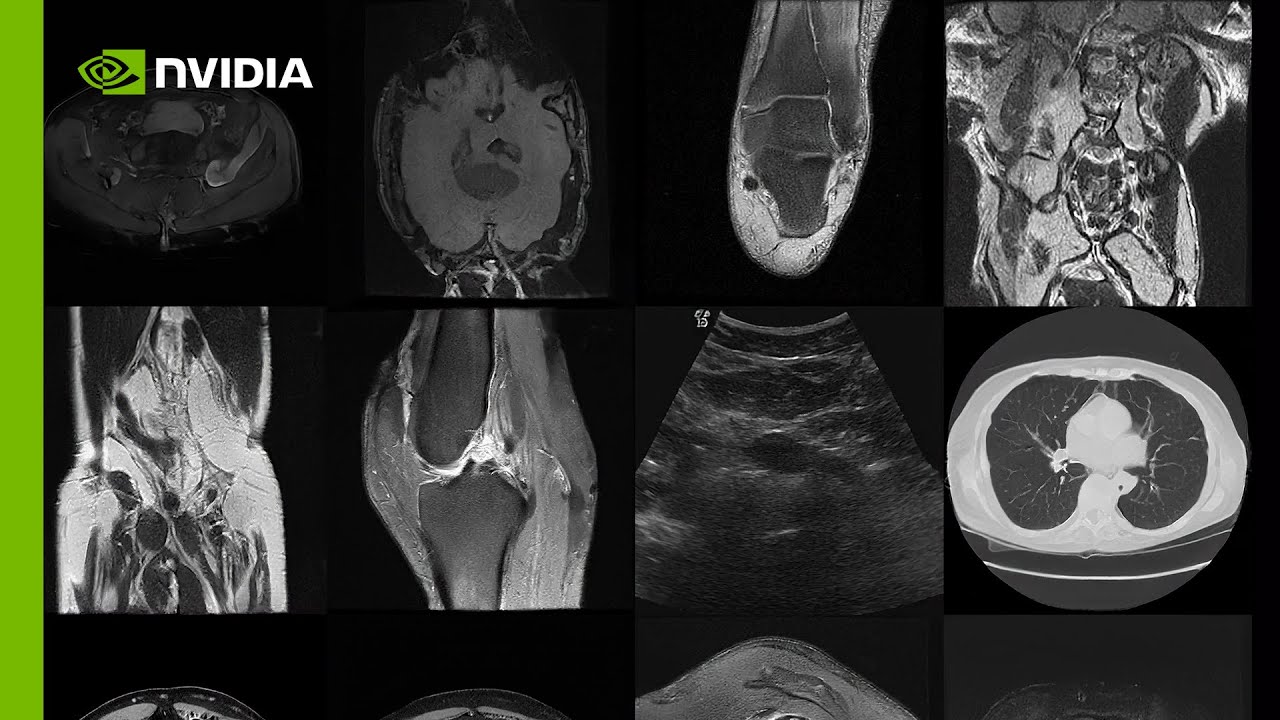
Create Infinite Medical Imaging Data with Generative AI

Regulasi dan Kebijakan Artificial Intelligence dalam Keamanan Siber
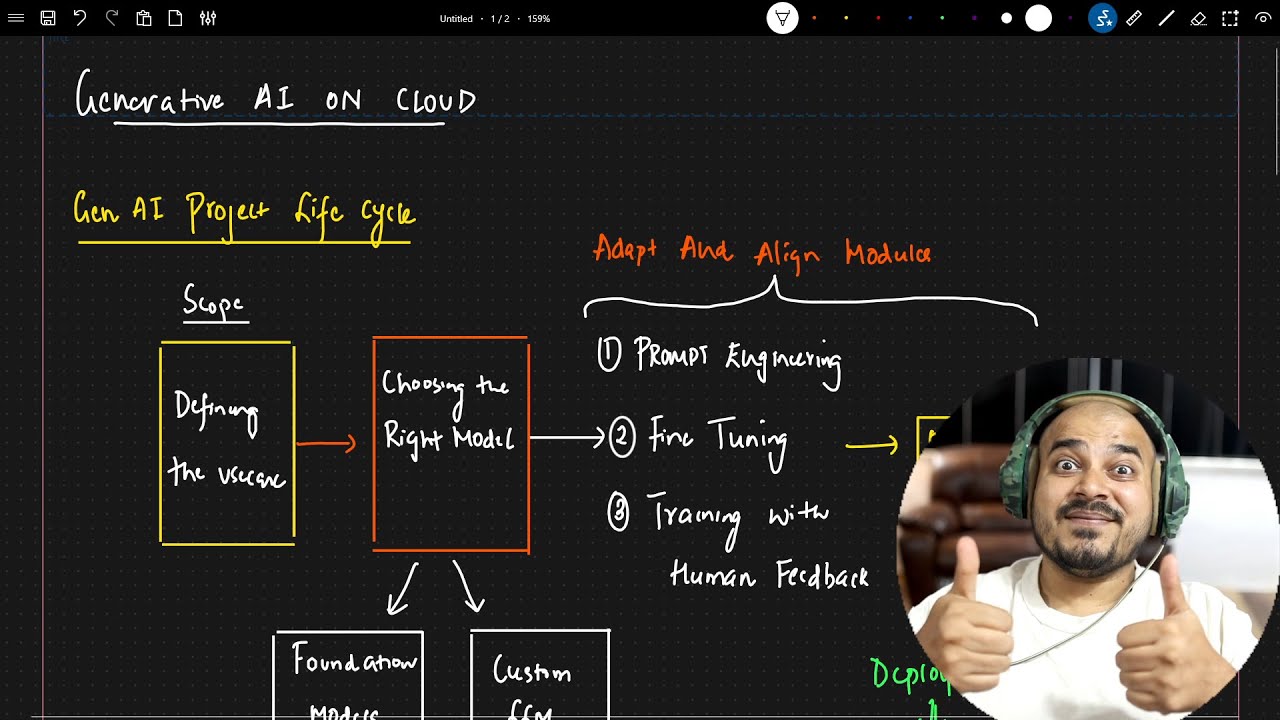
Generative AI Project Lifecycle-GENAI On Cloud
5.0 / 5 (0 votes)