Joost Huiskens | Mobile Healthcare
Summary
TLDRIn a dynamic discussion led by Joost Huiskens, the challenges of implementing artificial intelligence (AI) in healthcare are explored. The focus shifts from innovation creation to effective application, emphasizing the need for clinical relevance and data extraction methods. Key topics include the importance of randomized studies, ethical considerations, and the necessity of incorporating diverse stakeholders in algorithm evaluation committees. Huiskens highlights the gap between AI capabilities and real-world patient care, advocating for transparency, trust, and structured processes to enhance decision-making in clinical settings, ultimately aiming to improve patient outcomes through analytics.
Takeaways
- 😀 The primary challenge in implementing AI in healthcare is demonstrating clinical relevance, which requires extensive studies and patient analysis.
- 🤔 Randomized studies are essential for proving the effectiveness of AI algorithms, but they pose significant logistical challenges.
- 📊 Data extraction is a crucial aspect of utilizing AI, and effective data management is necessary to overcome its inherent difficulties.
- 🔄 Standardization of data across neonatal intensive care centers in the Netherlands is vital for improving AI applications and sharing outcomes.
- 👥 An algorithm evaluation committee should include diverse stakeholders such as patients, healthcare providers, data scientists, and ethicists to ensure comprehensive assessment.
- 🏥 Hospitals face significant hurdles in applying AI effectively due to varying levels of data collection and usage practices among healthcare professionals.
- 💡 AI and analytics can significantly enhance a physician's efficiency and decision-making capabilities, rather than replacing them.
- ⚖️ Regulatory frameworks for AI in healthcare are becoming stricter, and adherence to these regulations is essential for safe implementation.
- 🔍 Transparency in AI processes is critical for healthcare professionals to understand and trust AI recommendations in patient care.
- 🌍 Collaboration across institutions and sharing insights from larger trials can foster better AI applications and improve patient outcomes.
Q & A
What is the main challenge highlighted regarding the implementation of artificial intelligence (AI) in healthcare?
-The primary challenge is not the creation of innovations but rather their application and implementation in clinical settings. There's a significant gap between the potential of AI and its practical use in patient care.
How is clinical relevance established for AI algorithms in healthcare?
-Clinical relevance can be demonstrated through randomized studies that compare patient outcomes with and without exposure to the algorithm. However, this approach can be challenging as it may require analyzing thousands of patients.
What role does data extraction play in implementing AI in healthcare?
-Data extraction is essential for gathering the necessary information to inform AI algorithms. Challenges exist in ensuring that data is collected consistently and accurately from clinical practices.
Who should be included in an algorithm evaluation committee?
-An evaluation committee should consist of patients, healthcare providers, data scientists, ethicists, and statisticians to ensure a comprehensive review of the algorithms' clinical applicability.
What ethical considerations are mentioned regarding the use of AI in healthcare?
-Ethical considerations include the need for transparent processes in the development and application of AI algorithms to ensure patient safety and the efficacy of care.
How can the healthcare community address the challenges of data consistency?
-Standardization of data collection practices across neonatal intensive care centers in the Netherlands is proposed to facilitate better data integration and analysis.
What are the implications of regulatory frameworks on AI in healthcare?
-Regulatory frameworks will require that AI algorithms used in patient care are treated as medical devices, which means they must undergo rigorous testing and approval processes to ensure safety and effectiveness.
Why is trust important in the acceptance of AI among healthcare providers?
-Trust is crucial because healthcare providers need to understand and believe in the reliability of AI insights to incorporate them into their decision-making processes. If they do not comprehend the AI's workings, they are less likely to act on its recommendations.
What strategies are suggested for improving the implementation of AI in clinical settings?
-Strategies include creating clear guidelines for data governance, ensuring transparency in algorithm processes, and involving healthcare professionals in the development and evaluation phases to enhance usability and acceptance.
How can the integration of analytics improve patient care?
-Analytics can lead to better decision-making by providing insights from large datasets, ultimately enhancing patient care quality and outcomes. The focus should be on making healthcare providers more efficient in their roles rather than on replacing them.
Outlines
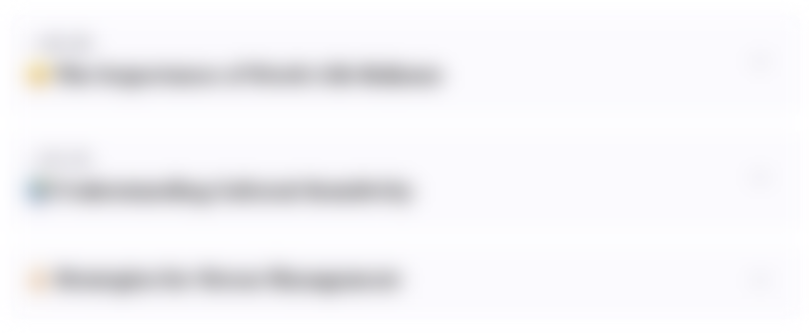
This section is available to paid users only. Please upgrade to access this part.
Upgrade NowMindmap
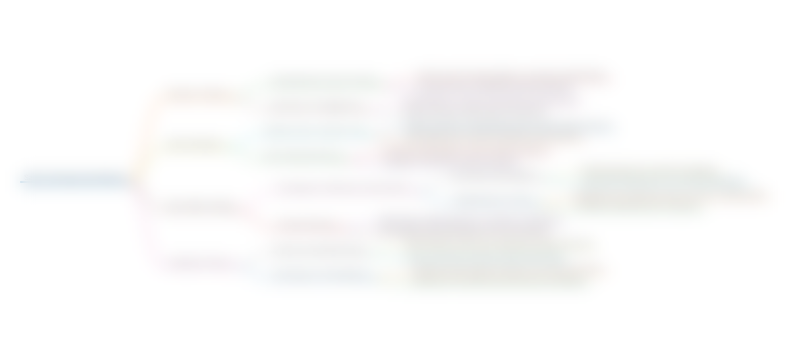
This section is available to paid users only. Please upgrade to access this part.
Upgrade NowKeywords
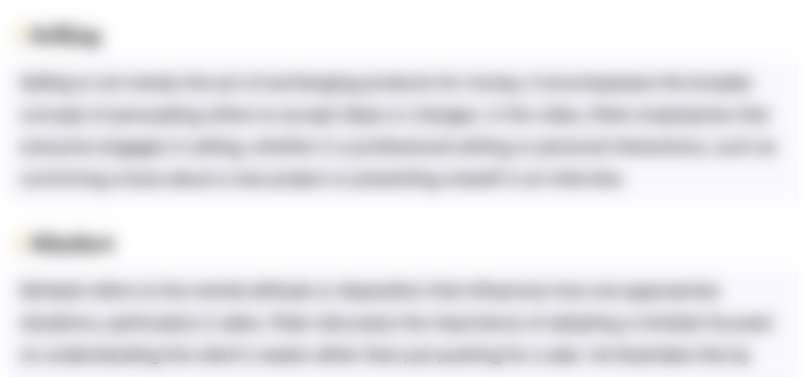
This section is available to paid users only. Please upgrade to access this part.
Upgrade NowHighlights
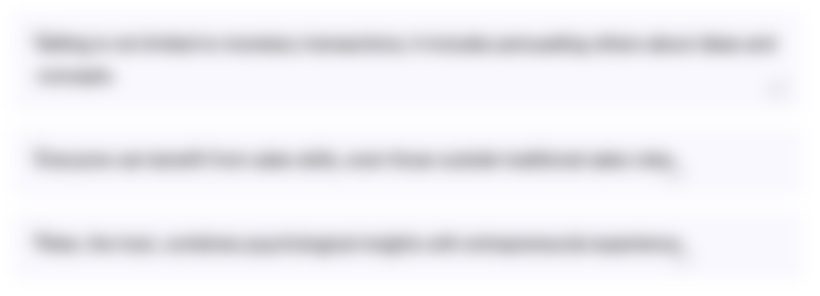
This section is available to paid users only. Please upgrade to access this part.
Upgrade NowTranscripts
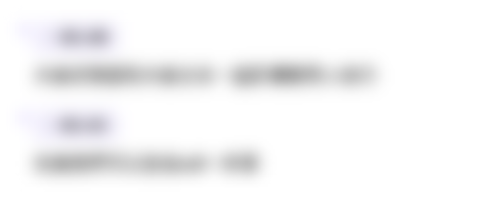
This section is available to paid users only. Please upgrade to access this part.
Upgrade NowBrowse More Related Video
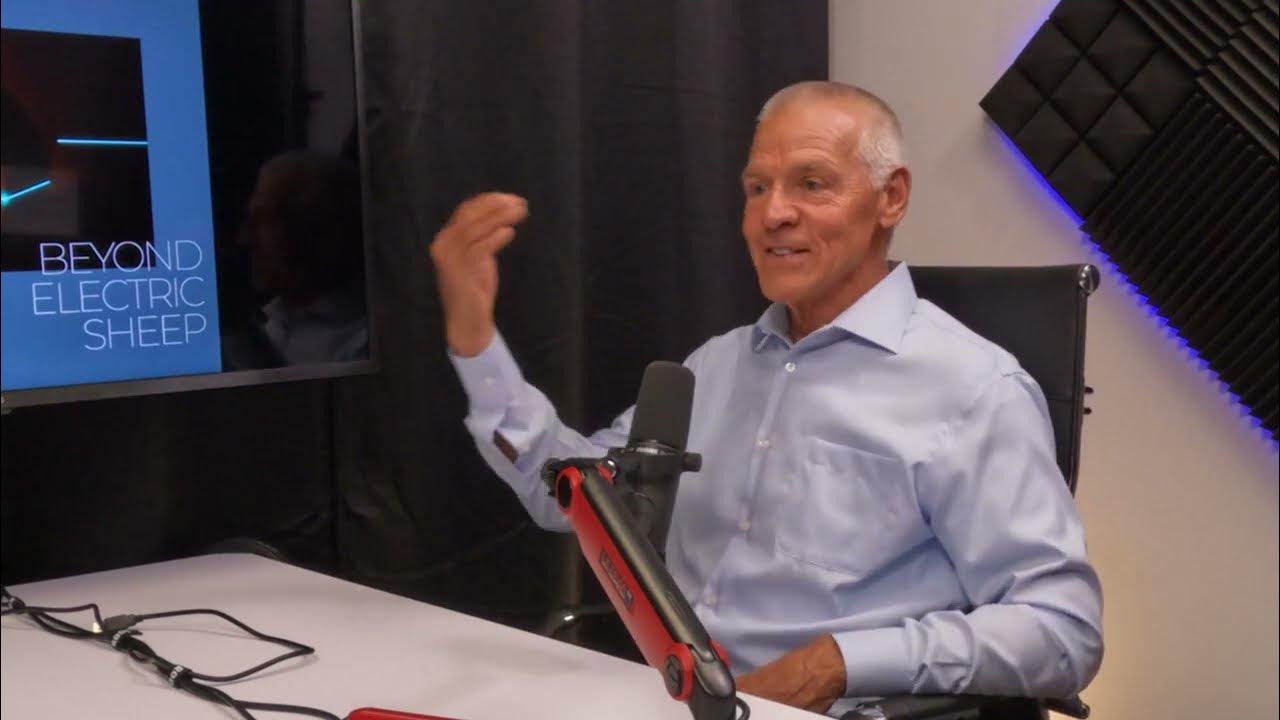
Episode 19 – AI in Clinical Medicine - Beyond Electric Sheep - A Podcast on AI

Evidence Based Medicine, Kuliah Fartok 2021
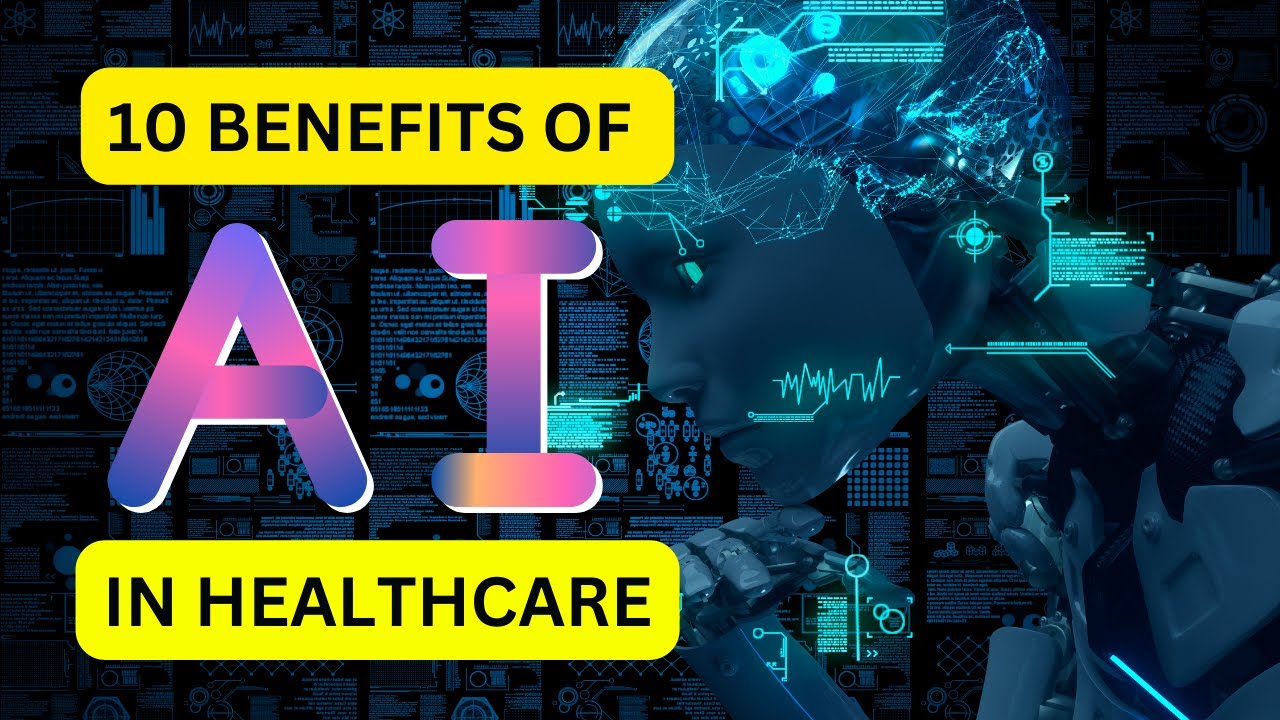
10 Benefits of Artificial intelligence in Healthcare
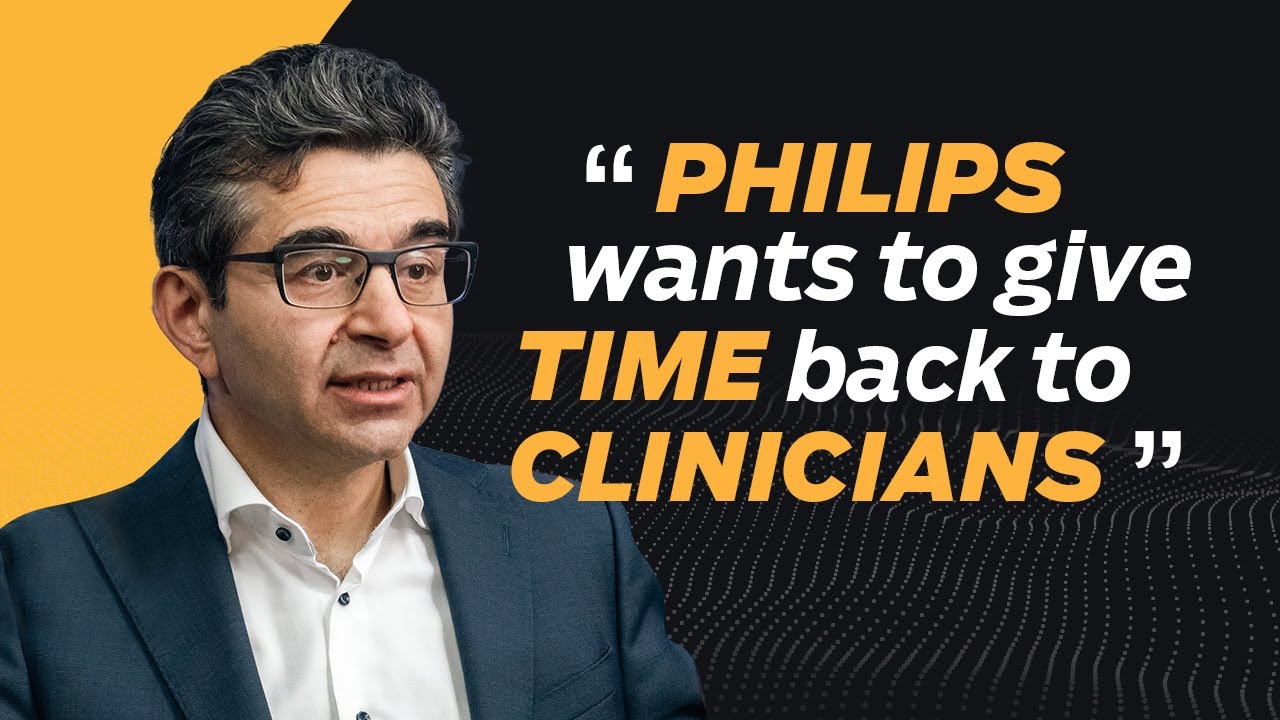
Tell me why time is a barrier to better health?

Smart Nation 2.0: Professor Joseph Sung on artificial intelligence in science

1- Information Management -- Data
5.0 / 5 (0 votes)