Hiring Manager Explains: Speak Like a Data Analyst
Summary
TLDRIn this video, Christine, a data director and bootcamp founder, shares insights on using key data analyst terminology to stand out in job interviews and the workplace. She covers technical, business, and analytical phrases such as metrics, dimensions, KPIs, and exploratory analysis, explaining how to use them with both technical teams and non-technical stakeholders. Christine emphasizes the importance of using this lingo in interviews, resumes, and projects to demonstrate confidence and job fit. She also provides tips on enhancing project writeups, interview preparation, and improving resume matching for recruiters.
Takeaways
- 📊 Using data analyst lingo in job interviews and projects helps showcase confidence and industry knowledge.
- 📈 Metrics, also called KPIs, are critical for data analysis, tracking quantitative values like sales revenue or user count.
- 📍 Dimensions provide context to metrics, such as time, location, or product categories, and are used to slice and dice data.
- 🔑 Understanding table grain helps define the level of detail in a dataset, which can enhance your interview performance.
- 💡 Business phrases like Northstar KPIs and requirements gathering are useful when working with non-technical stakeholders.
- 📉 Growth rates (WoW, MoM, YoY) and seasonality are essential for assessing company performance over time.
- 🛠 Exploratory analysis helps identify trends and patterns before formal testing and is a key part of data analysis.
- 📅 Performance measurement involves tracking metrics against goals or forecasts to assess success.
- 📊 Historical values offer insights into trends by comparing current data with past metrics for deeper analysis.
- 📋 Leverage data analyst lingo in project writeups, resumes, and interviews to show expertise and readiness for the role.
Q & A
Why is using data analyst lingo important in the job search?
-Using data analyst lingo is important because it signals to hiring managers that you are confident in your abilities and that you fit the culture and requirements of the role. It demonstrates that you can effectively communicate with both technical and non-technical teams.
What are metrics in the context of data analysis?
-Metrics, also known as measures or KPIs (Key Performance Indicators), are quantitative values that can be measured and analyzed, such as sales revenue or number of customers. They are the backbone of a data analyst’s job, used to report, measure, analyze, and predict performance.
What is the difference between a metric and a dimension?
-A metric is a quantitative value that can be measured, like revenue or sales, while a dimension is a qualitative characteristic that provides context to the metrics, such as time, location, or product category.
How can you use the term 'table grain' in a live coding interview?
-You can use 'table grain' to describe the level of detail or granularity in a dataset. For example, you might explain that the grain of a sales table is daily transactions per store, where each row represents the day, the store, and the number of transactions.
What is the role of 'requirements gathering' in data projects?
-Requirements gathering is the phase where data analysts determine what stakeholders need, including the specific questions they want answered, the deliverables, and the timeline for the project.
What are 'Northstar KPIs' and how are they used?
-Northstar KPIs are the top metrics that capture a company’s overall performance. For instance, in a subscription-based business, Northstar KPIs could be monthly bookings, subscriptions, and active users.
Why is understanding 'seasonality' important in data analysis?
-Understanding seasonality is important because it allows analysts to identify regular ups and downs in data that occur at specific times of the year. This helps in making more accurate forecasts and understanding patterns in business performance.
What is exploratory analysis and when is it used?
-Exploratory analysis is when data is examined to uncover trends, patterns, or insights without testing a specific hypothesis. It is often used at the beginning of an analysis to find unexpected patterns before conducting more formal analysis.
How can using data analyst lingo improve your resume?
-Incorporating data analyst lingo into your resume, such as metrics, dimensions, Northstar KPIs, and exploratory analysis, can improve ATS (Applicant Tracking System) matching and show recruiters that you are familiar with the terminology and responsibilities of the job.
What are some examples of questions you can ask in an interview to demonstrate data literacy?
-To demonstrate data literacy in an interview, you can ask questions like 'What is the level of data integrity and data quality at the company?', 'How does the data analytics team work with the data engineering team?', or 'What are the Northstar KPIs for the departments I would be working with?'
Outlines
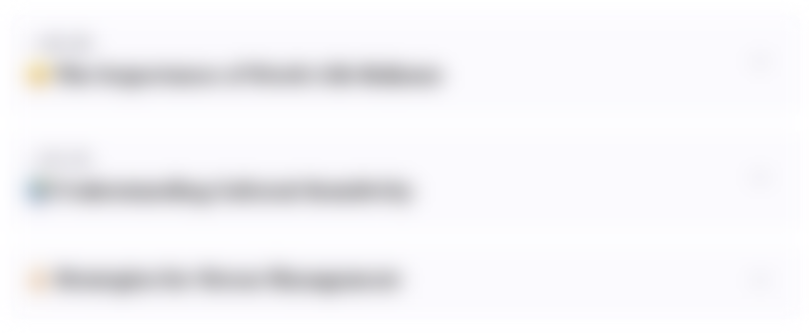
This section is available to paid users only. Please upgrade to access this part.
Upgrade NowMindmap
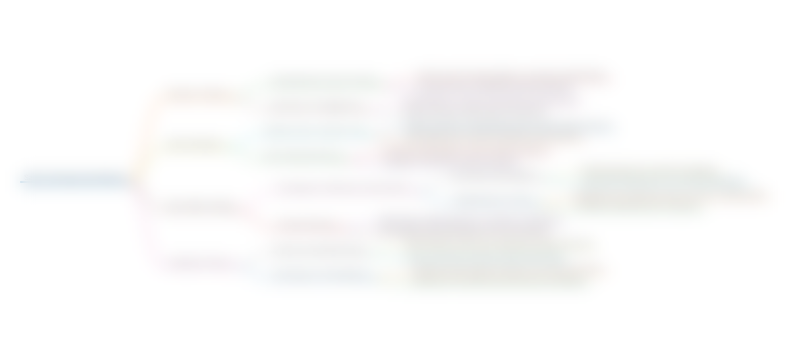
This section is available to paid users only. Please upgrade to access this part.
Upgrade NowKeywords
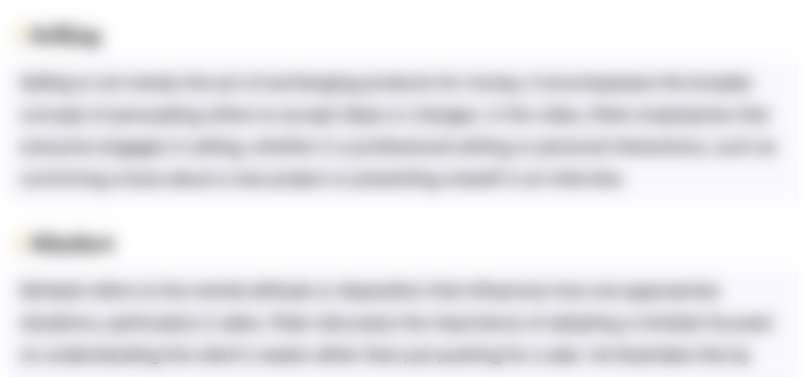
This section is available to paid users only. Please upgrade to access this part.
Upgrade NowHighlights
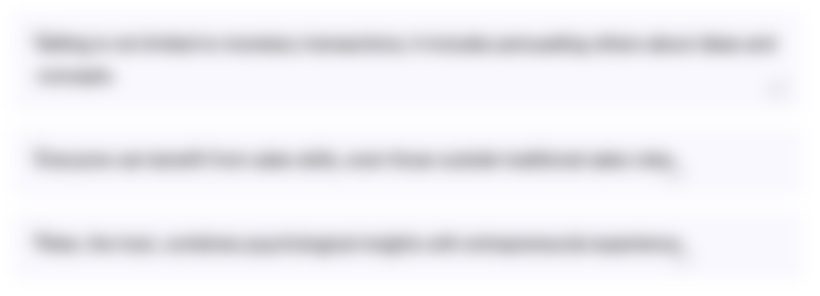
This section is available to paid users only. Please upgrade to access this part.
Upgrade NowTranscripts
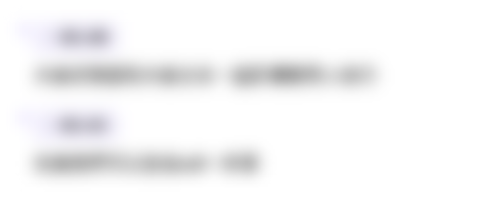
This section is available to paid users only. Please upgrade to access this part.
Upgrade NowBrowse More Related Video
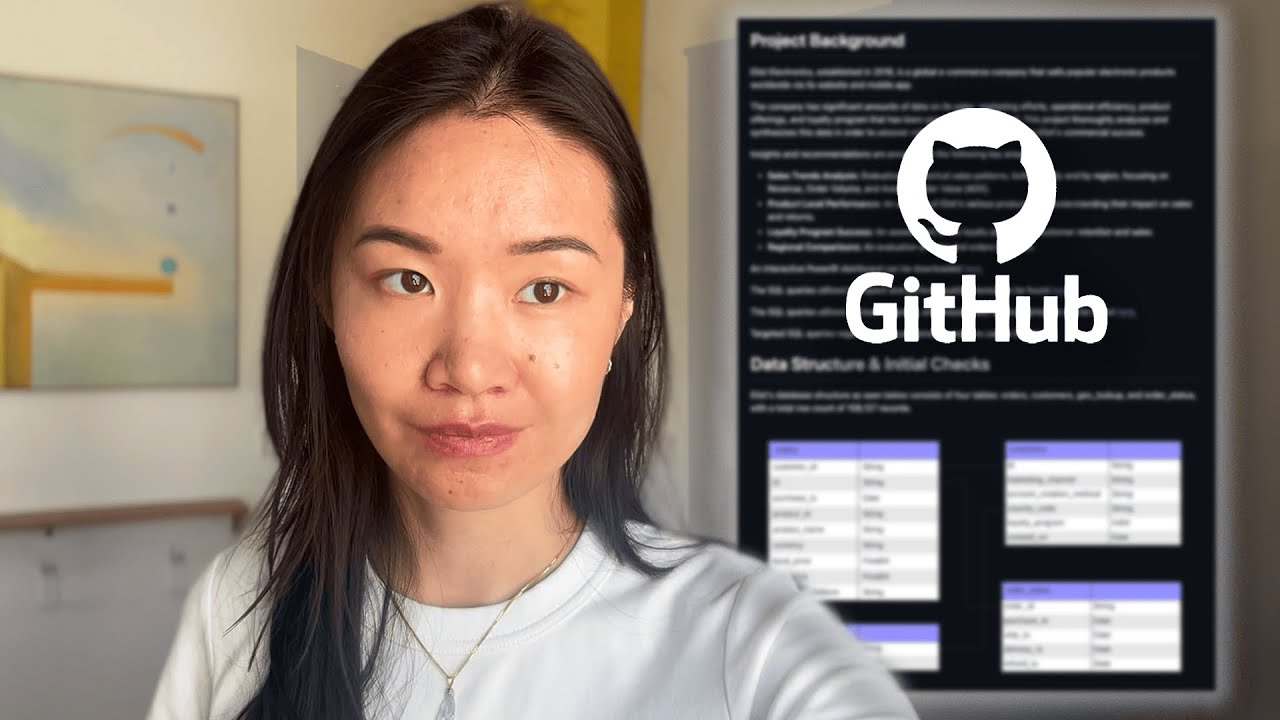
Create a Standout Project Write-Up | Data Analyst Insights
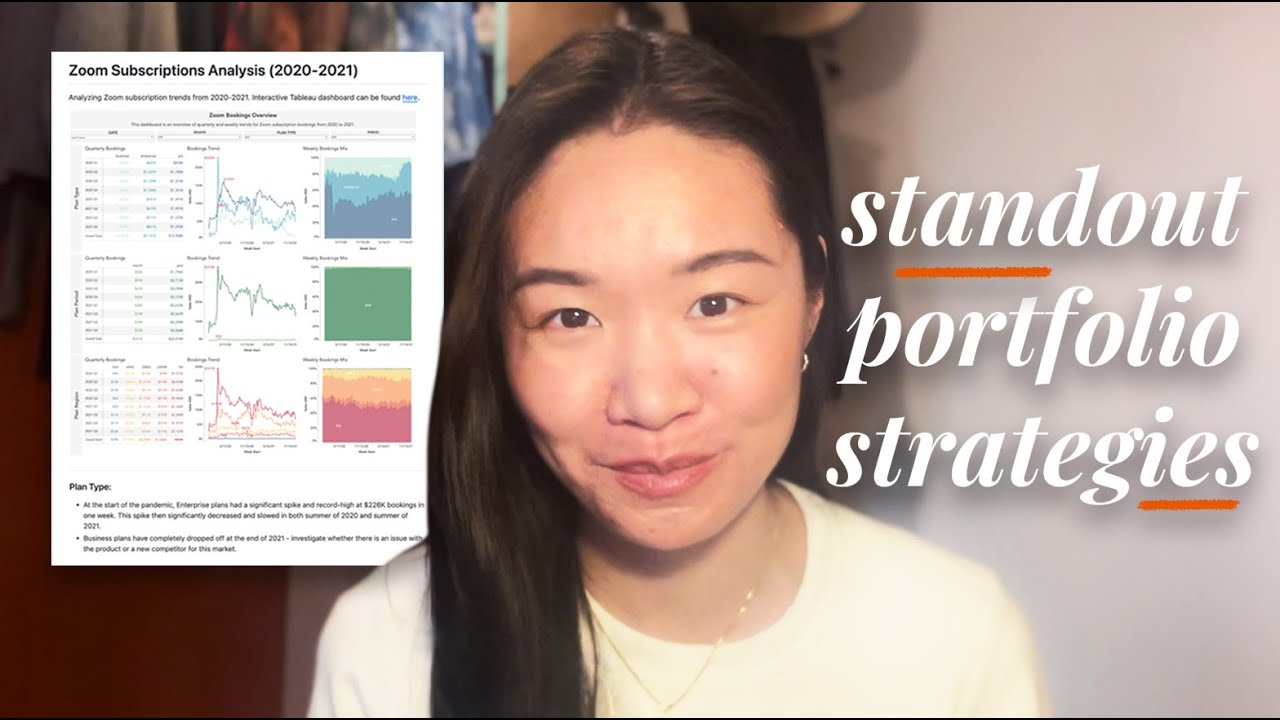
Hiring Manager Explains: Data Portfolio Do’s and Don’ts
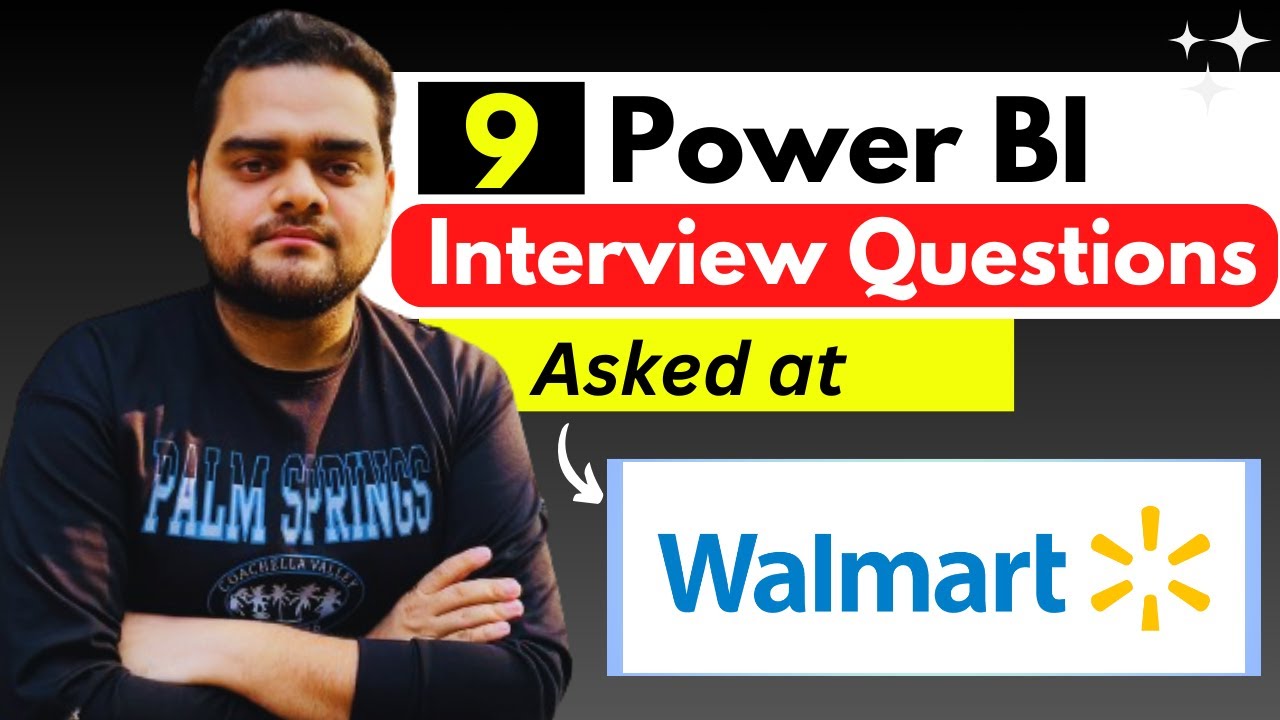
Walmart - 9 Interview Questions you must know | Power BI 😲
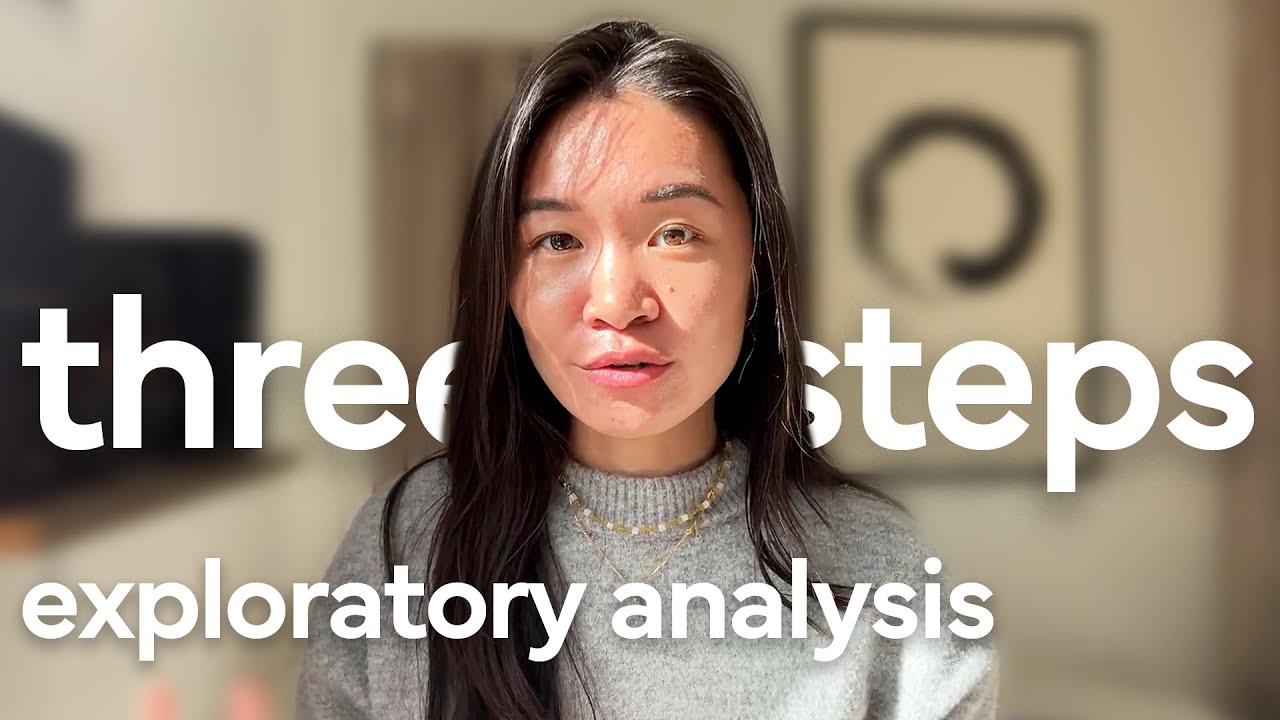
The EDA framework data hiring managers love (use it in your portfolio projects)
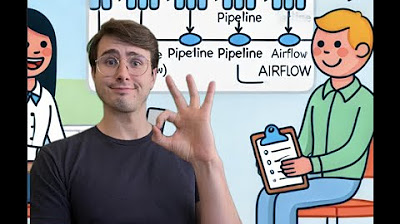
Data Engineering Interview Guide! How to Get a Data Engineering Job!
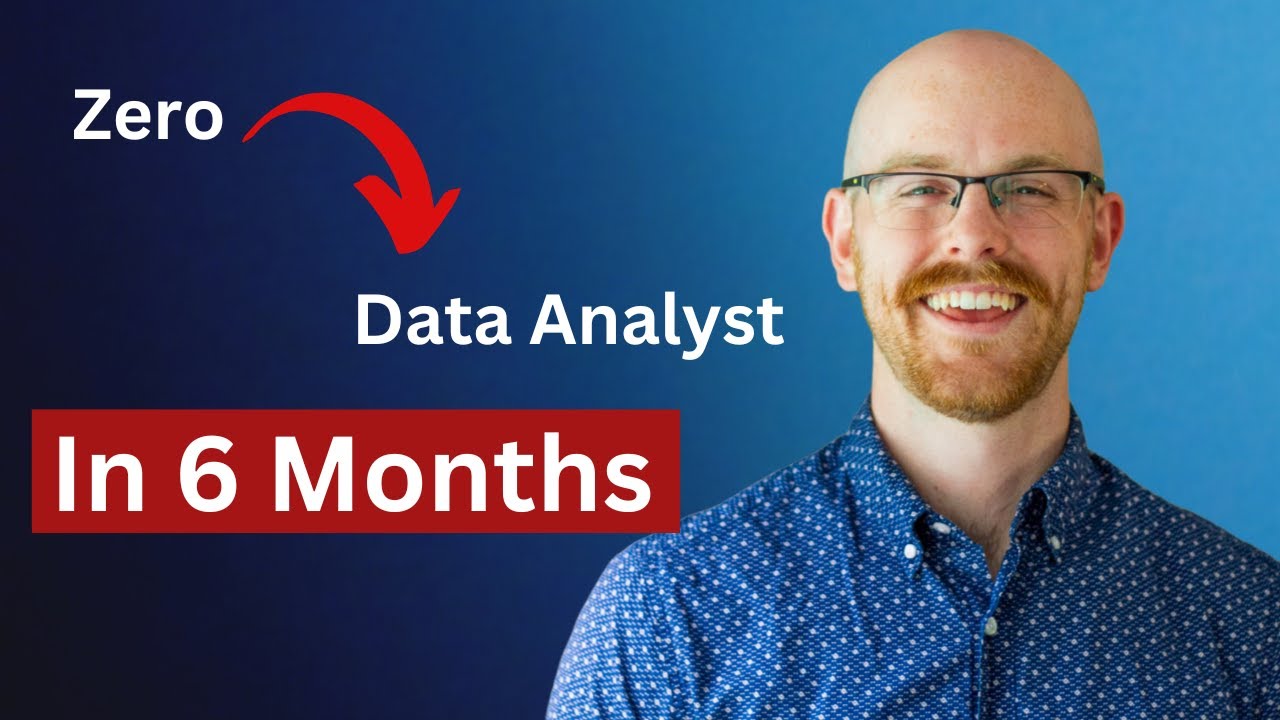
How I Would Become a Data Analyst if I had to Start Over in 2024 | 6 Month Plan
5.0 / 5 (0 votes)