How AIs, like ChatGPT, Learn
Summary
TLDRThis video script delves into the pervasive yet mysterious world of algorithms that govern our digital experiences. It explains how these complex bots, initially built through a process of trial and error by simpler bots, evolve to perform tasks beyond human comprehension. Despite their creators' inability to fully understand their inner workings, these algorithms shape our online interactions, from video recommendations to financial transactions, often driven by the relentless pursuit of data to improve their accuracy. The script concludes with a humorous nod to the irony of being governed by bots while also asking viewers to engage with the content to influence algorithmic decisions.
Takeaways
- 🌐 Algorithms are pervasive on the internet, influencing what we see, buy, and how we interact with various platforms.
- 👀 Companies are reticent to disclose how their algorithms work due to the competitive advantage and trade secret nature of their technology.
- 🤖 Modern algorithms are often too complex for humans to fully understand, even the developers who created them.
- 🧠 The process of creating algorithms involves 'builder bots' that construct other bots and 'teacher bots' that test them, using a trial-and-error methodology.
- 🔄 The iterative process of testing, building, and refining bots leads to increasingly effective algorithms over time.
- 📈 The effectiveness of algorithms is measured by how well they perform in specific tasks, such as recognizing images or recommending content.
- 📊 More data leads to longer and more comprehensive tests, which in turn results in the development of better algorithms.
- 🔍 Algorithms are not just tools; they are also used to test and manipulate human behavior, as seen in the way content is recommended to keep users engaged.
- 🔑 The success of an algorithm is often determined by its performance on tests designed by humans, highlighting the ongoing interplay between human intention and machine learning.
- 🌟 Despite the complexity and sometimes mysterious inner workings of algorithms, they are an integral part of our digital lives and are here to stay.
Q & A
How do algorithms influence our online experiences?
-Algorithms shape our online experiences by determining what content we see, set prices, monitor transactions for fraud, and even decide which videos to recommend on platforms like NetMeTube.
Why is it difficult for humans to provide explicit instructions for complex algorithmic tasks?
-Many problems are too large and complex for humans to write simple instructions for. For instance, identifying fraudulent transactions among billions of financial transactions per second or selecting videos from an almost infinite library for recommendation.
How do companies protect the secrets of their algorithmic bots?
-Companies treat their algorithmic bots as valuable employees and guard the details of their functioning as fiercely protected trade secrets.
What is the process of creating an algorithmic bot that can recognize images?
-A builder bot creates student bots with random connections, which are then tested by a teacher bot using a set of labeled images. The best-performing bots are selected, replicated with variations, and the process repeats until a bot can recognize images effectively.
Why do algorithms sometimes fail to understand complex or nuanced tasks?
-Algorithmic bots are often good at specific tasks they have been trained for but may fail at others, such as understanding videos, recognizing upside-down images, or distinguishing between unrelated objects.
How does the process of building and testing algorithmic bots relate to the concept of evolution?
-The process resembles evolution, where the builder bot selects the 'fittest' bots (those that perform best in tests), replicates them with variations, and discards the rest, leading to gradual improvement over many iterations.
What role does data play in improving the performance of algorithmic bots?
-Data is crucial for creating longer and more comprehensive tests, which in turn lead to better-performing bots. More data allows for more extensive testing and fine-tuning of the bots' abilities.
How do algorithmic bots determine what content to show users on platforms like NetMeTube?
-Bots are tested on their ability to keep users engaged on the platform. They analyze user behavior and select content that maximizes the time users spend on the site.
Why do companies collect vast amounts of user data?
-Companies collect data to create more extensive tests for their algorithmic bots, which helps improve the bots' performance and accuracy in tasks such as content recommendation or user engagement.
What is the significance of the 'Are you human?' tests on websites?
-These tests serve a dual purpose: they verify that the respondent is a human, and they also contribute to the training data used to improve bots' ability to recognize and respond to human-like inputs.
How do algorithmic bots impact the way content is selected and presented on social media platforms?
-Bots are used to analyze user preferences and interactions to select and prioritize content that is most likely to engage users, such as posts, articles, or videos, based on their past behavior and preferences.
Outlines
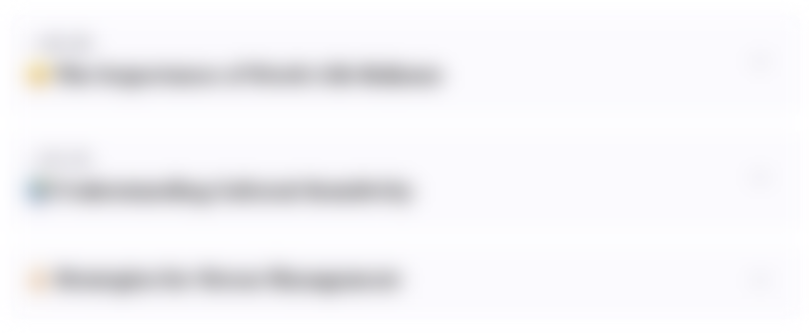
This section is available to paid users only. Please upgrade to access this part.
Upgrade NowMindmap
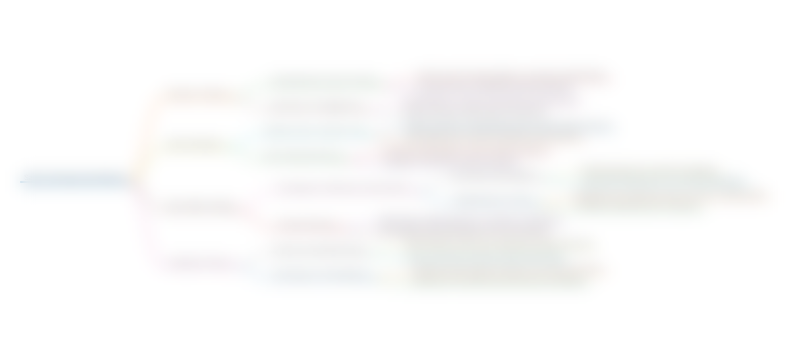
This section is available to paid users only. Please upgrade to access this part.
Upgrade NowKeywords
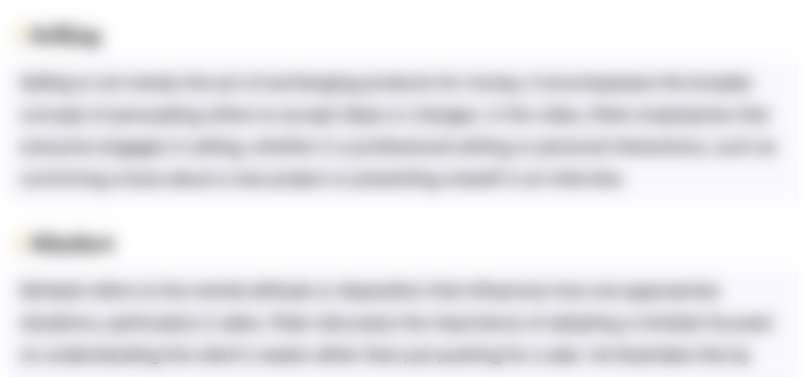
This section is available to paid users only. Please upgrade to access this part.
Upgrade NowHighlights
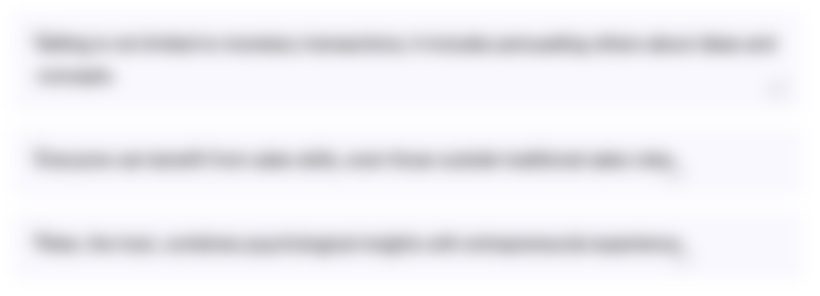
This section is available to paid users only. Please upgrade to access this part.
Upgrade NowTranscripts
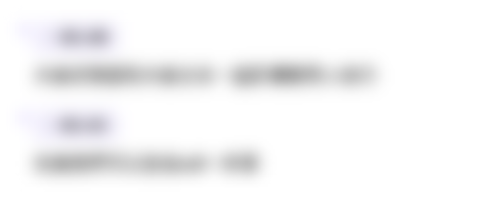
This section is available to paid users only. Please upgrade to access this part.
Upgrade NowBrowse More Related Video
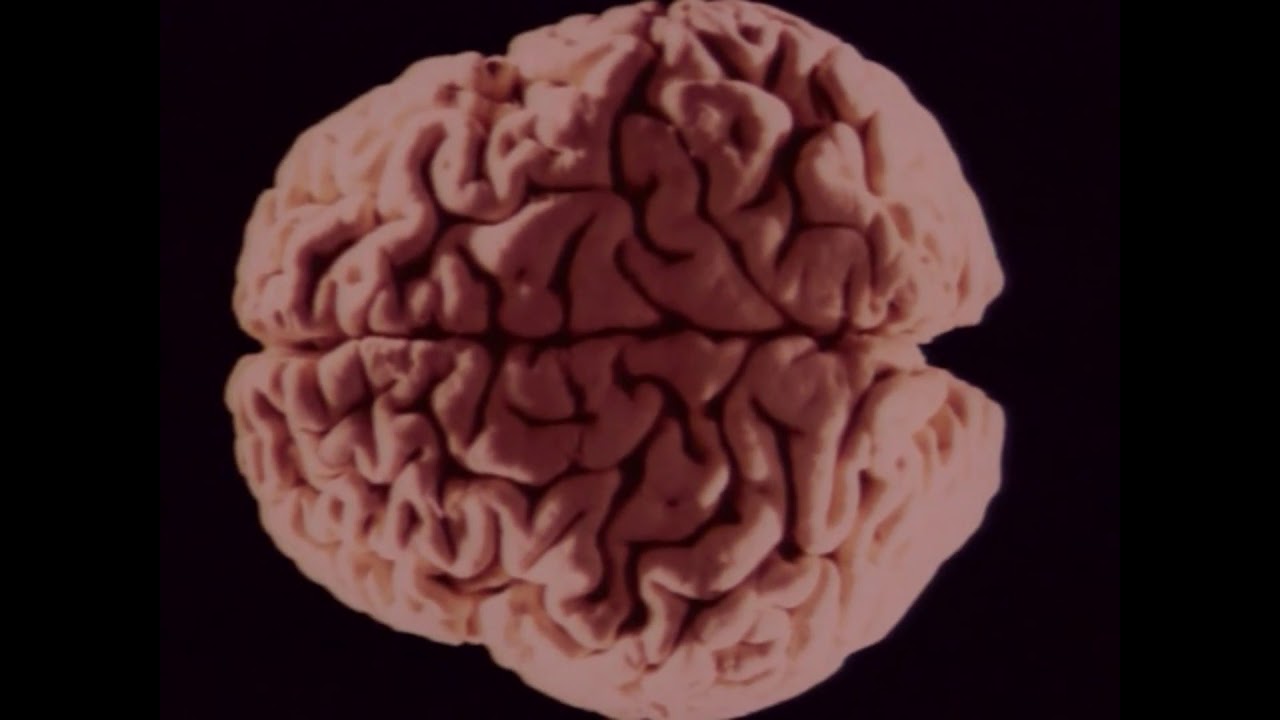
The Brain | Part 13 | Discovery Channel Body Atlas

How algorithms shape our world - Kevin Slavin
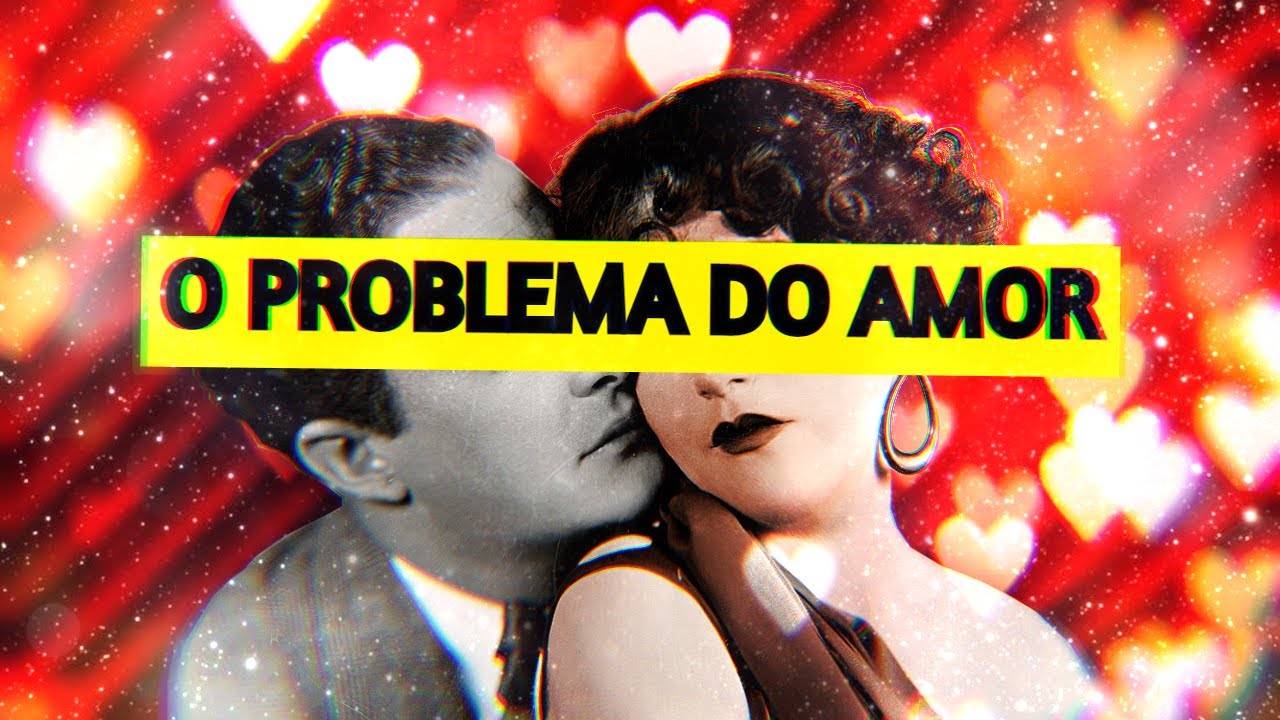
Apenas mentirosos podem falar sobre o amor.
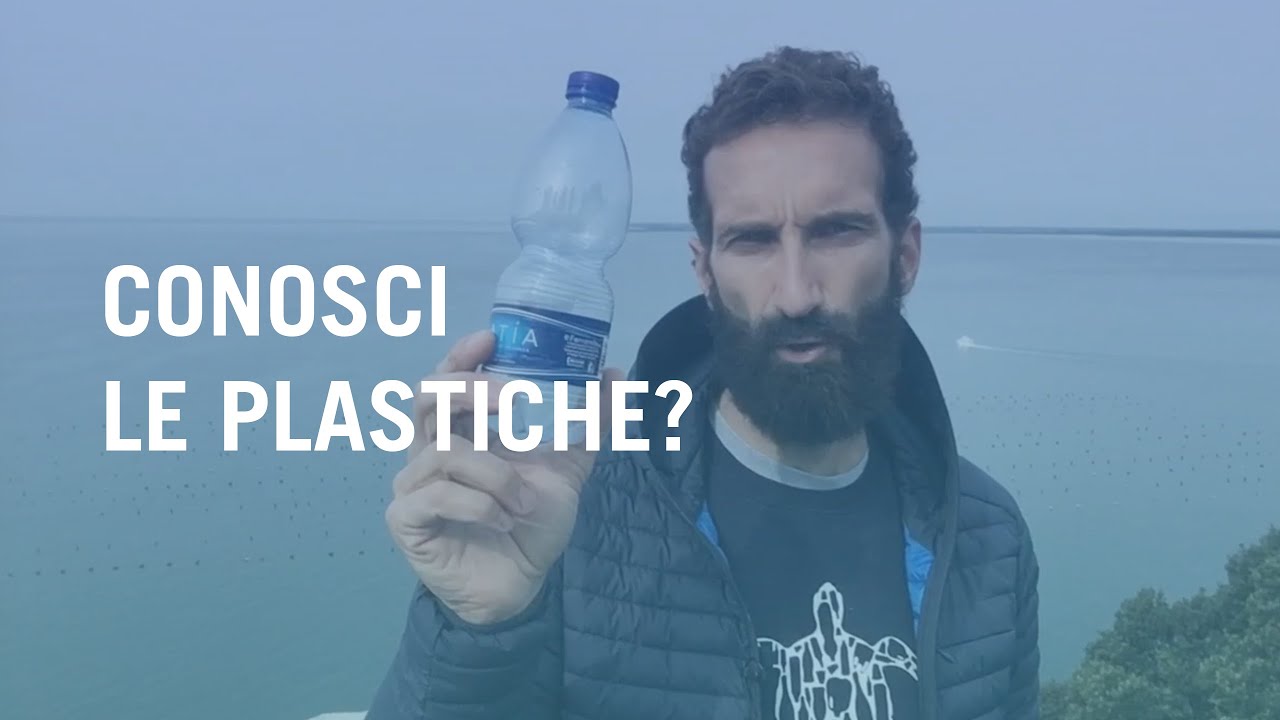
Breve guida ai diversi tipi di plastiche utilizzate // Alex Bellini

Nature's Numbers By: Ian Stewart (Chapter 2: WHAT MATHEMATICS IS FOR?)

Painted with numbers: mathematical patterns in nature
5.0 / 5 (0 votes)