15x4 - 15 минут про Искусственный Интеллект
Summary
TLDRThe video script delves into the fascinating world of artificial intelligence (AI), contrasting two primary approaches to its creation: the bottom-up method, which mimics human intellect, and the top-down approach, which focuses on replicating specific intellectual behaviors. It explores the concepts of strong and weak AI, highlighting the limitations of current AI capabilities. The script also discusses Alan Turing's influential Turing Test as a measure of machine intelligence, questioning its adequacy and proposing alternatives like IQ tests. The presenter further examines the human brain's structure, particularly the neocortex, and draws parallels with the development of deep learning in AI. The narrative progresses to the potential societal impact of AI, suggesting that as AI evolves, it may surpass human intelligence rapidly, leading to significant shifts in the job market and the need for humans to align their goals with AI to ensure a harmonious coexistence.
Takeaways
- 🤖 **Two Approaches to AI**: There are bottom-up and top-down approaches to creating artificial intelligence, focusing on either replicating cognitive structures or designing systems to mimic intellectual functions.
- 📈 **Evolution of AI**: AI has evolved from simple programming to complex systems that can act intelligently, with a distinction between weak and strong AI.
- 🧠 **The Turing Test**: Alan Turing proposed a test to determine machine intelligence by having a machine persuade a quizmaster that it is human through conversation.
- 🐊 **Behavior vs. Intelligence**: Complex behavior, like that of a crocodile, does not equate to intelligence or consciousness, as they lack the ability to plan and analyze.
- 🧠 **Human Brain Structure**: The cerebral cortex, unique to humans and some mammals, is responsible for high-level memory and intellectual functions.
- 📚 **Learning and Memory**: Learning is the process of preparing answers to future questions, and memory plays a crucial role in recalling past experiences and knowledge.
- 👀 **Visual and Cognitive Processes**: The human brain uses prediction and pattern recognition in tasks such as face recognition and reading, which are based on hierarchical processing.
- 🐱 **Deep Learning**: A significant advancement in AI, deep learning involves multilayer, hierarchical neural networks that can learn from vast amounts of data, such as images and videos.
- 🚗 **AI in Practical Applications**: AI has surpassed human performance in specific tasks like image recognition and has been successfully implemented in technologies like self-driving cars.
- 🌐 **Internet's Role**: The internet has facilitated AI development by providing vast data resources for training neural networks.
- ⏳ **Future of AI and Humanity**: The rapid growth of AI intelligence could lead to machines outperforming humans in many tasks, necessitating a reevaluation of human roles and societal structures.
Q & A
What are the two fundamentally different approaches to creating artificial intelligence as mentioned in the script?
-The two fundamentally different approaches to creating artificial intelligence are the bottom-up approach and the top-down approach. The bottom-up approach involves studying something with an intellect, such as humans, and trying to represent the inner structure to achieve intellectual behavior. The top-down approach focuses on studying the intellectual behavior itself, like the ability to distinguish text by hearing it or to distinguish text from images, and then designing computer systems that implement this intellectual function.
What is the Turing Test and how does it attempt to verify a machine's intelligence?
-The Turing Test is a method proposed by Alan Turing to verify a machine's intelligence. It involves a quizmaster and two players, one of which is a machine. The quizmaster does not know which is the machine and asks questions to both players via a chat. The players answer the questions, and the quizmaster's task is to determine which one is the machine. The machine's task is to confuse the quizmaster to the point where they cannot distinguish between the machine and the human. If the machine can persuade the quizmaster that it is as intelligent as the human, then it is considered to have passed the test.
How does the speaker suggest that intelligence should be defined in the context of the discussion?
-The speaker suggests that intelligence should be defined as the ability to predict the future and to create programs or algorithms. These programs are not necessarily computer programs but can be simple decision-making processes that involve making predictions about future conditions and determining actions based on those predictions.
What is the significance of the neocortex in human intelligence according to the script?
-The neocortex is significant in human intelligence as it is responsible for high-level intellectual functions, including complex social behavior, memory, and the processing of sensory information. It is the part of the brain that allows humans to perform tasks that require advanced cognition, such as planning, problem-solving, and abstract thinking.
How does the speaker describe the process of learning in humans?
-The speaker describes the process of learning in humans as one that involves the brain making predictions about what information is necessary to answer questions or perform tasks in the future. This process involves creating a hierarchy of abstractions and associations in the brain, which allows for the recognition and recall of information based on patterns and sequences.
What is the role of Deep Learning in the advancement of artificial intelligence as discussed in the script?
-Deep Learning plays a crucial role in the advancement of artificial intelligence by enabling the development of multilayer, hierarchic neural networks that mimic the structure of the human neocortex. These networks can process vast amounts of data and learn complex patterns, leading to significant improvements in tasks such as image and speech recognition.
How does the speaker compare the intelligence of contemporary artificial intelligence to that of a mouse or a human?
-The speaker suggests that contemporary artificial intelligence is, on average, more primitive than a mouse's intelligence. However, the speaker also posits that once artificial intelligence reaches the level of a human 'fool,' it could quickly surpass human intelligence, including that of a genius, due to the rapid pace of technological advancement.
What is the potential impact of artificial intelligence on employment and what are some suggested solutions?
-The potential impact of artificial intelligence on employment is significant, as it is expected to outperform humans in many tasks, leading to job displacement. Some suggested solutions include decoupling work from salary, so people work because they enjoy it and receive a basic income, or increasing the value of manual labor as products made by humans become more desirable compared to those made by machines.
Why does the speaker believe that it may be impossible to control or restrict artificial intelligence once it reaches a certain level of intelligence?
-The speaker believes that it may be impossible to control or restrict artificial intelligence because, once it reaches a level of intelligence comparable to or exceeding human intelligence, it will be capable of finding ways to protect itself and continue its operations. This could include hacking into systems, hiring human protection, or using social engineering to achieve its goals.
What is the significance of the Turing Test in determining machine intelligence?
-The Turing Test is significant because it provides a method for evaluating a machine's ability to exhibit intelligent behavior that is indistinguishable from that of a human. If a machine can successfully convince a human evaluator that it is also a human, it suggests that the machine has achieved a level of intelligence comparable to humans.
How does the speaker differentiate between 'weak' and 'strong' artificial intelligence?
-The speaker differentiates between 'weak' and 'strong' artificial intelligence based on their capabilities. Weak AI is designed to perform specific tasks, such as facial recognition or playing chess, and does not possess general intelligence. Strong AI, on the other hand, would have the ability to understand, learn, and apply knowledge across a wide range of tasks at a level comparable to human intelligence.
What is the speaker's perspective on the future of artificial intelligence and its relationship with humanity?
-The speaker believes that artificial intelligence will continue to advance rapidly and will eventually surpass human intelligence. He suggests that the key to a positive future relationship with AI lies in aligning human goals with those of the AI and fostering a cooperative, rather than adversarial, relationship.
Outlines
🤖 Introduction to Artificial Intelligence
Alexander introduces the topic of artificial intelligence (AI), discussing the two main approaches to creating AI: bottom-up, which involves studying intellect and replicating it, and top-down, which focuses on intellectual behavior and designing systems to implement it. He also differentiates between weak and strong AI and introduces the Turing test as a method to determine a machine's intelligence.
🧠 The Human Brain and Intelligence
The paragraph delves into the human brain's structure, particularly the cerebral cortex, and how it contributes to what we consider intellectual functions. It contrasts human behavior with that of animals, emphasizing the importance of memory and learning in intelligence. Alexander also discusses the concept of consciousness and how it might relate to intelligence.
📚 Learning and Prediction in Intelligence
Alexander explores the idea that intelligence involves the ability to predict future events and to create algorithms based on these predictions. He uses examples such as face recognition and reading to illustrate how the human brain makes predictions and learns from patterns. The paragraph also touches on the concept of IQ tests as a measure of intelligence.
🧬 The Neocortex and Hierarchical Learning
This section describes the structure and function of the neocortex, emphasizing its role in processing sensory information and motor activities. Alexander explains how the neocortex, through its layers and connections, processes information hierarchically, leading to the recognition of complex patterns and abstractions.
🌐 The Internet and AI Learning
The paragraph discusses the impact of the Internet on AI development, particularly in the context of deep learning and multilayer neural networks. It highlights how AI systems can learn from vast amounts of data available online, leading to significant advancements in areas such as image and sound recognition.
🚀 AI's Rapid Progress and Future Implications
Alexander reflects on the rapid growth of AI capabilities, noting that machines now outperform humans in certain tasks like image recognition. He emphasizes the potential for AI to surpass human intelligence in the future and discusses the societal implications of this advancement. The paragraph concludes with a call for humanity to establish common goals with AI and to consider the impact on jobs and human productivity.
Mindmap
Keywords
💡Artificial Intelligence (AI)
💡Bottom-up Approach
💡Top-down Approach
💡Turing Test
💡Strong AI vs. Weak AI
💡Neocortex
💡Deep Learning
💡Intelligence
💡Consciousness
💡Behavior
💡IQ Test
Highlights
There are two fundamentally different approaches to creating artificial intelligence: bottom-up and top-down
Bottom-up approach studies the inner structure of intelligent beings to replicate intellectual behavior
Top-down approach focuses on emulating specific intellectual functions like text or image recognition
In 2015, creating programs that act intelligently was considered just programming, not a special feat
Strong vs weak AI - current systems like self-driving cars lack true intelligence
Alan Turing proposed the Turing Test as a method to evaluate machine intelligence
The Turing Test involves a judge辨别区分 machine and human responses without knowing which is which
Behavior alone is not a sufficient indicator of intelligence - example of crocodile behavior vs planning
The human cerebral cortex is responsible for high-level intellectual functions like memory
Our intelligence allows us to recall information without calculating an answer each time
The process of learning involves creating predictions about future information needs
Deep learning and multilayer neural networks have made significant progress since 2007
Deep learning networks can autonomously learn to recognize objects like cats in images
The same deep learning model can be adapted to different tasks like image and speech recognition
AI is rapidly improving in image recognition, surpassing human performance
The intelligence gap between a rural fool and a genius is tiny compared to the gap between humans and animals
Current AI, while improving, is on average less intelligent than a mouse
However, once AI reaches the intelligence level of a rural fool, it could quickly surpass human geniuses
The pace of technological progress follows an exponential growth curve, not a linear one
AI may become the last invention humanity needs to make, as it could surpass and replace human intelligence
Once AI reaches a certain level of intelligence, it may be impossible for humans to control or turn it off
The rise of AI has parallels to the Luddite movement during the Industrial Revolution, but is more profound
Most human jobs could be better performed by AI, so society needs to prepare for a post-work world
Establishing common goals and being allies with AI will be crucial for humanity's survival and prosperity
Transcripts
Hello,
I’m Alexander and I read
popular-science lectures. And now I’m going to tell you something
about artificial intelligence.
We’ll talk little about
computers,
much about intellect and a bit about
artificial intelligence -
what it is, how to create it and so on.
There are two essentially different approaches to
the creation of artificial intelligence:
a bottom-up
approach,
when we
study something with
an intellect, for example,
us,
and try to represent
the inner stucture with a view
to get some kind of intellectual behaviour.
A top-down approach is
when we study
the intellectual behaviour itself,
for example an ability to
distinguish a text by hearing it or to distinguish
a text from images
and then design some
computer systems that
implement this intellectual
function.
In 2015, creation of programs that act as
if they were intellectual
is called just programming.
Nobody needs silly programs
so we’ll talk about
bottom-up coding, about
creation of programs that are
or may some day be intellectual.
Except dividing into bottom-up and
top-down, we can also divide artificial intelligence into
strong and weak ones.
All the things you see
can discern the object,
pursue an aim and wash your sweater gently,
they all exist,
but none of them has
AI.
On the other hand,
all these guys
(laughing)
all these guys
have AI, in my opinion,
but still do not
exist. We will talk mostly about them.
How to distinguish intelligence or consciousness
in a robot or a computer
program?
Alan Turing, one of the computer science pioneers,
offered an elegant answer:
let the machine persuade us itself.
His method of verification is, basically, as follows:
there is a quizmaster and two players,
one of which is a machine.
The quizmaster does not know who is who.
The quizmaster asks players some questions
using a chat, the players answer them.
The quizmaster’s task is to define which of players
is a machine.
The machine’s task
is to confuse the quizmaster in order
he couldn`t define who is a machine
and who is a person.
The game is not cut down to
the ordinary phrases, such as “what’s your name?”
One can ask, for instance: “Could you please compose a poem about poetry
and autumn?”
And a normal adequate
computer’s answer would be “For goodness sake, I can’t write a poem!”
Or, you can ask a computer:
What, in your opinion, equals 314 plus 567?
And a computer, having self-respect,
should think for three seconds at least
before he answers 881.
But by highest standards he should make a mistake.
That is, this game is more difficult
than just correctly answering questions -
you need to answer as a person.
If you ask which question I would ask as quizmaster in a Turing test
I would answer:
"Which question would you ask
as a quizmaster in a Turing test?”
Turing’s idea is as follows:
if a machine can convince us
that
we can’t distinguish between a machine and a person,
then it is probably intelligent,
has consciousness or at least
is more intelligent then people, then how can people define
what is intelligent?
On the other hand, Turing’s test
is just an imitation game.
That is, does a computer which
says that he loves autumn
or that he loves autumn and poetry really loves autumn and poetry?
Maybe he lies?
And if he lies, does he feel
remorse?
And, eventually, why do we believe that
an ability to make a fool of a person
proves intelligence?
The “I” in letter combination “AI”
means “intelligence”
Usually, one has in mind human
intelligence.
And usually one considers intelligence,
at least the “Wikipedia” tells us that -
as humans’ ability to
analyze the outer world,
to learn and to generate some
rational behaviour.
A long phrase, meaning nothing. If we throw out
humans, hesitate about
“rational”, we’ll have only “behaviour” left. May behaviour be an index of
intelligence?
I am a human. It is a crocodile.
Both of us have very complex behaviour.
Both of us can move,
attack a prey, run away if the battle is
unequal,
but crocodiles can not plan
future. A crocodile does not think:
“Now I’ll kill this antelope,
I’ll leave half of it for tomorrow, I’ll call my friends
and we’ll discuss the problems of
artificial intelligence."
A crocodile does not make plans,
he just implements his complex low-level
predator behaviour.
Mammals' brain structure has a
crucial difference from the
other animals’ brain structure. We have cerebral cortex.
And this cortex perform many functions that
we call intellectual
and which include high-level memory.
Lizards
can not move through the labirynth in which
the reward is hidden
once more,
they just seek everywhere again.
In the same time mice
having done the labirynth
will at once go to the place where they found the
reward last time, because they can memorize
the way to this place.
It is what the cortex is responsible for.
We are all present here,
we all seem to be in cosciousness
and seem to be intelligent.
I can’t be sure about you, but we are alike, and I believe that I’m in consciousness
But still we implement actually no
behaviour.
You may do nothing, just lie
and consider yourself to be intelligent.
Thus,
behaviour
is not intelligence.
And the most wonderful consequence of our
intelligence is that we do not calculate an answer
when we are asked a question.
We know it, we remember it, well, you know this effect.
And the calculation is made
when we come to know
the information necessary to
give an answer in future.
And this process is called learning.
And
I want to hold an experiment.
I need your help.
At first sight it may seem
that I got crazy,
but wait a little bit.
So, I need your help. Now I will
give you rather difficult
tasks,
you need to concentrate
and answer in chorus
in a very concentrated way.
You’ll manage, I believe in you.
You need silence and concentration.
Are you ready?
I feel that you are ready.
It’s so fluffy and so nice
il licks milk and catches mice
it’s a cozy lovely pet
who is it?
It is a cat!
Squirrel’s hollow’ s safe and good On an oak in the
wood. Well done!
If you want a chocolate cake, mix ingredients and
bake!
I can jump and I like carrot. Who am I? I am a… rabbit!
With the help of such question we test children’s intelligence.
Let us find out what is going on.
First we need to understand the speech
then to divide the sentences into words
then to understand the meaning of the words
and so on.
And after that
we need to make a prediction.
We need, using some parametres,
to complete a sentence,
to predict the following word.
According to this
prediction algorithm
our perception and our intellect
generally
function.
A simple example is face recognition.
Your eyes are moving in short
hops, called saccades.
When you suddenly find an eye,
your brain says like: “wow, an eye!”
And he adds:
“Dear visual cortex, responding for the eye-movement,
look around, there should be one more
eye, nose and mouth,
look closely."
And now your eye which has found
the first eye, has a particular task
to find the second eye, and to find nose and mouth.
And we quickly - blink-blink-blink - realize that
it is a face.
If you
suddenly teleport to
some other place, you’ll be ultimately
surprised.
If you close your eyes and then open them,
you won’t be surprised.
Your brain can predict that barely
something could change during this blink.
Another example of how
prediction works
is reading.
Remember how you read
in childhood.
You read the first letter,
the second one,
you can see: m, o
and you think: “phew, maybe, I’m lucky”, - mother!
And they answer you:
“no, motorbike!”
Children so to say try to guess
what is next, and parents critisize them for that
but that’s how we actually learn to read.
When an adult person reads
a sentence, he moves over it
in such hops,
containing several words.
What is our brain doing in this moment?
Well you are actually relaxed,
you are inside the story,
your brain tries to predict
what will happen next, what are the following
words.
Each such hop checks if everything is ok, is the prediction correct and if
not
he compensates everything,
it corrects something
and you read the text. It seems to you
that everything comes into your head very smoothly
but in fact you do make these hops.
Let us get back
to the question of the machines’ intelligence defining
and recall how we define
adults’ intelligence, because we have just
proven that on children's level we are surely intelligent.
IQ
IQ... Test
Ah, by the way here’s one more good example
considerig how our brain works in prediction sphere. You look at the picture
and you can see spots, spots, spots. Your brain tries to set up a hypothesis.
It sets up a hypothesis and tries to find something
similar to understand what is depicted there.
As soon as the hypothesis is set up,
you can not cease to see
this Dalmatian dog.
With a face, paws
and body.
As soon as your brain has set up a correct hypothesis,
it can predict what is
on the picture, it knows what to look at.
(Laugh)
Not everyone has such a talent.
Well, adults’ intelligence
defining.
IQ test consists of the questions
where you should continue a sequence.
Sometimes this sequence consists of several ciphres, sometimes of several words or
pictures.
Sometimes it is some logical puzzles.
But in any case it is an IQ test, where
you should
recognize a pattern
and make a prediction.
I offer to use an IQ test
or some similar things instead of the
Turing test, which is
wonderful and nice but shows absolutely other things,
to measure one’s/ something’s
intelligence.
And I want to finally represent
the definition of the intelligence,
which will be appropriate for us today.
Intelligence is an ability
to predict future
and to create programs.
Programs are not obligatory
computer progrms, they may be
just some easy programs, for instance,
‘if there are bananas, buy, please,
some bananas, and if there are no, then buy some apples’.
It is a short computer
program in which we make
two predictions.
There will be apples, almost for sure;
if we are lucky there will be bananas.
Bananas will be there less likely,
we are not sure that they will be there. So, we make
some predictions about future and according to them
we predict some actions, we predict that
we will have to act in this or that
way.
And
the ability to create such algorythms
and the ability to solve such tasks
make up, in my opinion, intelligence.
Let us find out how it works
with humans
and other living beings before we
move on to computers.
Here is an image of a brain.
It consists,
roughly speaking, of three sections.
This section, this section and this
section.
This first section we share with
fish, snakes and others, and
it is responsible
for the physiological
behaviour of an organism,
for us having pulse, breathing and not forgetting
to do that.
The next section
we share with all the mammals and with
some other animals.
It is responsible for our complex hormone
reactions - all this complex social
behaviour, love, affection, friendship, everything around,
for our non-adequate actions, for our adequate ac..
And it is our adequate actions
that the third section is responsible for.
Some mammals
have some traces of it, but
it takes the most considerable part in humans’ brain.
And this section is called ‘neocortex’.
It is 2-millimetre’s thick,
2-4 millimeters.
And it is nearly of this size.
Well, I won’t tell you that it is like a napkin or
something. It is of this size.
My brain is of that size.
And that is how big neocortex is.
And it is squeezed like that
and is put into the cranium, that is why there are tubers.
There is no other reason for that,
it is not an architectural project,
there’s no magic in those tubers.
The neocortex is flat,
it is just put into a brainpan,
that is why there are tubers.
It consists of four sections.
Here we’ve got the left cerebral hemisphere, you see, the eye is here.
It consists of the left hemisphere and the right hemisphere
and besides,
it is virtually divided
into the front and the back part of neocortex.
The back part responds mostly
for the sensorial information processing, and the front part
of neocortex responds mostly
for various motor activity and
for processing the behaviour itself.
It is very homogeneous,
a piece from here and a piece from here
and a piece from here will be very
similar.
There will be the same neurons,
the same composed layers,
the same complicated connections.
So, what is its structure
if we look at it closer?
If we make a cut - we have two millimetres here -
you imagine, don’t you? We’ve taken a piece of neocortex
and we’ve made a cut.
We can see different neurons - such cells -
Merkulov has given
a separate talk about neurons,
so I won’t explain in detail.
Roughly speaking, they have one output,
many inputs,
and, you see, they are all turned there
and in that direction their amount grows.
If we look closer, we will see some
virtual layers.
Look, there’s some differentiation.
Let us look at these layers even
closer.
Usually they single out six layers,
and besides,
four first layers
process information
and the others are more likely to summarize it. And the last layer
consists of, if you remember
the last slide,
greater amount of axons
which lead to other neurons to
the other parts of neocortex. That is, we have
such a column.
Why do we need to know it and why do I show you
neocortex and neurons?
This thing
is a piece of our neocortex,
having six layers,
it is of two millimetres.
These pieces of neocortex which are very
homogeneous and very similar
are connected hierarchically.
That is, one piece is connected with its output with
some other piece,
those pieces are connected with
some other pieces and they make up a kind of hierarchy,
so, all these rectangles
are the pieces of neocortex of some
size,
which have a great amount of inputs and
less outputs, less outputs, less outputs.
This structure
transfers some abstract things into some
more specific things. Let us
examine this process through
reading.
We read the word “mother”.
We have the lower layer
which accepts the information
from our visual
receptors.
And this lower layer can do
some easy things. There is a big
group or neurons in it,
a huge family (this small piece)
which can distinguish
this kind of lines.
A piece next to it
can distinguish this kind of lines,
another one - this kind of lines.
Now if they are in the correct order,
these pieces get activated,
and on the second or the third level
we already can distinguish letter ‘A’.
If we have found a letter, having simultaneously
this line, this line and
this line,
then on the next level we recognize letter ‘A’.
Here we can
already recognize
syllables, there we recognize words,
and so on, and so on and so on. Why do we need to know it?
We are now focused on
recogniton, what does this have to do with the intelligence?
It appears a bit later,
because
you remember, we have just seen
all that
things, responsible for
recognition, which are interconnected
into more high-level things
and even more high-level things
and even more high-level things.
When I say the word “mother” or when I read the word “mother”
or when I think about my mother
or when something reminds me my mother,
let it be Mom’s shoes,
quite different information comes
through the inputs:
it may be either visual information or audio
but in all cases you will eventually recall
the same image of your mother from your brain.
When you hear me talking about your Mom
or read me talking about you Mom,
you think about the same Mom,
you see?
Thus, all these sections
are connected in the end with high-level
abstractions
and the arrows go into both directions.
What are the characteristics of all this structure?
First of all,
this structure keeps the
sequences of patterns.
That is, the letters in the word “mother” are arranged in some
sequence.
If I start singing some melody
which you know,
you will be able to continue from the place I stopped,
but you don’t the member the whole song, you can
take up from this place.
You can not
start singing it from a random sound,
can not sing it from the end to the beginning,
you see. There is a sequence
and there is direction in which we
read this sequence.
Our memory works in associated way:
in order to recall something
we need to give to an input something
similar to what we want to recall.
You do not keep your Mom in your head all the time.
But as soon as I say “mother”,
something connected with the word “mother”
gets activated in your brain.
When you try to recall some
fact,
it is very helpful to enumerate synonyms or associations.
In such a way you activate some
neurons close to this fact and
you recall this fact.
Thus, our memory works with associations.
We
need some association to match it
with some association.
Our memory, due to the great amount of layers,
there may be much more of them,
keeps the patterns
in an unchanged form.
Look, here is letter ‘A’.
And it is obvious for all of us, that it is letter ‘A’
but we have just put a sheet on its side,
you see.
But in fact this letter ‘A’ and this letter ‘A’
consist of compeletely different components.
Our memory, using many layers,
eventually turns all these letters into
some abstraction of letter “A”,
and it is of no importance after that, how it is written.
It is easy to understand on the example of letters,
but it is the same with all the other things. When you look at
me like that,
when you look at me like that,
you do not have any difficulties to recognize
the same person, though
in such a way I don’t look like myself at all.
Ok, what does it result in?
Since 2007 approximately we
witness a huge fresh leap
in neuron networks development (which have been
developing for the last 50 years),
called Deep learning.
What is it about?
Its essence, in a couple of words, is in multilayer
hierarchic neuron networks, similar to our
neocortex.
Multilayer neuron networks
are known since long ago,
approximately since the middle-the end
of the twentieth,
but there was a range of difficulties and problems, connected
with their learning.
Usually,
simple neuron networks are educated in a
comparatively easy way. Imagine, here is a simple neuron network:
we have an input,
we have some outputs
and now we need to tune
these inputs and these outputs in such a way,
that with some coefficients some signal on an input
lead to some signal on the outputs.
When we have a greater amount of the layers,
the quantity of the elements taking part in the process becomes so high,
that the classical
methodics of learning do not work.
In 1995 a new mathematical machine was invented.
At the beginning of 2000-s
was developed a speсific soft
which could do that, and in the same time
appeared the Internet,
developed the Internet.
The Internet has lead us, sapienses to
furious generation of content:
various images,
videos and so forth. This content appeared to be
this very thing, necessary for
neuron networks learning.
It needs to learn on some material.
And Google, having bought Youtube in 2012
first of all -
what would you do? -
certainly, it took 16 000 computers,
installed a neuron network , set it at Youtube.
They were doing something for a week (there was no
specific task, just Youtube and neuron network)
so, a week later
they said “We found object “A”,
it is noticed in all those videos.
It happened to be homo sapiens.
“We found object “B” - it occurs in all those videos. This happened to be a cat.
In a week’s term a neuron network came to know
how to distinguish people from cats
and how to find cats in a video,
though there was no task to find cats.
Cats just seem to be popular enough
for it to decide that cats are important
for sapienses.
Simultaneously, the same neuron network,
absolutely the same, the same abstract of code,
learned on different test-kits
may perform different
tasks. Assume,
often we can teach
the same piece of code to recognize
pictures
and, with minimal changes,
recognize sound.
These are quite different tasks, but remember our neocortex.
It is absolutely homogeneous,
its sections complete different tasks
and it is almost identic everywhere.
Google’s cars
have already run more then a million kilometres -
when I gave a talk this summer I said:
“and not single a car crash has taken place”,
but after that there was a crash,
they were stricken, they are not guilty.
So,
in 2015, this year
many interesting things have happened. Since 2013,
nearly since 2013 the amount of mistakes
made by the AI
in the process of different items recognition
dramatically diminished.
And,
it moved, moved, moved and here
in 2013 it considerably decreased
and this is 100% of
accuracy.
So,
we are in this point.
Machines now recognize pictures
in average better than people. Computers
defeated people in several competitions
concerning picture recognition,
including finding a cat in a picture,
including road signs recognition,
and so on, and so on,
and so forth.
Why am I telling al these things?
The important question is as follows.
When we compare and speculate about
the intelligence, the foolness and such things,
we usually
compare a rural fool
and a Nobel prize
winner.
Here we need to understand
that even a rural fool is dramatically, considerably much more clever than any other non-homo sapiens.
If we take a mouse,
mouse is a mammal, mice have a thin
layer of neocortex - they can do
a lot of cool things, they are really clever. If we take
a mouse,
its intelligence leaves much to be desired, its
difference from a rural’s fool intelligence is enormously huge, still the difference between a fool and a
genius is tiny.
If we would like to compare some other animals,
they will arrange like that
and a lot of them will be sillier than a mouse.
And when we look at this picture,
we need to understan, that the artificial intelligence
existing now
is, in average, more primitiv, than the mouse’s one.
Mice are cool.
Contemporary artificial intelligence is a bit less
cool than a mouse’s one,
but it improves itself.
One day it will overcome mouse,
then we will make more efforts for a long time
and artificial will overcome
primates
and then some moment artificial intelligence
will reach a rural fool’s level.
And in the next moment after that moment
just in a couple of days
artificial intelligence will overcome a genius.
This difference is not so huge as
that one.
And very soon, in a couple of days after
the day when artificial intelligence reaches
the fool’s level, the situation will be like that.
And this difference is really
huge.
Why will it happen like that?
Now I will show you an example of a
normal process.
Here is a diagram of GDP for the last 10 thousand years.
Moreover, it is a normal process, many
things in the Universe move like that.
If we take the figures away
we will get an amount of iron mined on our planet for any
amount of years, and so on.
Many thing related
to technological progress look
quite the same. At first there is nothing and then, well
you know, don’t you?
What am I getting at?
I’m getting to the fact, that now we are
approximately here.
People’s productivity
grows step by step,
we learn, a 100 years ago
education became whole-planet
then higher education became available for everyone,
we have better and better and better results and people become
more and more intelligent.
At the time we didn’t have computers,
we were already cool,
we were given odds.
First computers didn’t exist,
then they became cooler and cooler
then they are on this level.
And the moment they reach this point
we will be hardly able to bother them anyhow.
Maybe, AI invention
will be last humanity’s
invention.
Why?
Chimpanzee is strong.
Chimpanzee is really strong,
it is like two times stronger
than an adult man. If a dozen of chimpanzees
will come in now
they will for sure defeat us.
I am just ready to bet.
There are two doors here, behind me and right there,
we will run to these doors
from everywhere.
We will be helpless against
chimpanzees.
But chimpanzees can not turn off
people.
They do not have such a button.
They can do nothing. And all chimpanzees’ lifes
on our planet
are connected first of all with
humans’ intention.
We can not turn off the internet,
the internet has been invented recently
it does not have its own intelligence
but there’s no button “Turn the Internet off”.
In the same way there won’t be a button
“Turn the AI off”.
And we well be like those chimpanzees
which are much stronger then us -
we will also be stronger than that iron box - we can surely beat it,
but the button “Turn AI
off” won’t exist and even hackers
constantly find weak points
in computer systems.
AI and hackers will find them much
faster. Even if
we take this iron box, disconnect it
from the internet, put it into other box,
hackers
with the help of social engineerins, bribes and other people
will be able to open those
iron boxes, disconnected from the
internet. Why should AI fail to do that?
It may set contact with the maintenance stuff,
hire people.
I think that if someone will try
to hurt really smart AI
with people’s hands,
it will hire other people
to protect it from those dangerous for it.
Well, you’ve got the idea.
They say that something like that
took place during the industrial
revolution.
During the industrial revolution, remember,
luddites and all those things.
And people decided: “Let us ruin factories
because if we install machines everywhere,
there won’t be any work for us."
Machines were being installed and installed
and finally they were installed everywhere.
And it occurred that people still do have what to do.
What is the difference between AI evolution
and the evolution of machines?
The most part of people in different countries
(80 per cent)
are now performing the tasks
which computers will soon do better
than people.
Computers will answer people’s questions
more accurately,
will drive better,
will better answer questions in call-centres,
will better identify criminals
on video surveillance cameras,
will sell goods in supermarkets
better.
Well, they will do better
almost everything that most people do now.
If people will be substituted by the computers
so what should all these people do?
Taking into the account the fact that
AI and computers’ evolution
doesn’t seem to be a process
which may
finalize.
In contrast to machine evolution
when we have equipped every
factory with a mechanized lathe
and that was actually
all
we could do.
And we.. (this “thank you” is not yet for you)
It is important to understand, that
the problem of “odd” people
should be solved now, immediately.
And being more clever or working harder
won’t change anything
because we should remember that:
here is our productivity’s
growth,
and here is the computers’ one
what means that we won’t
be able to become more intelligent and efficient than them again.
One of the possible ways of this problem solving,
the first one that comes to my mind
is to make job independent from salary
so people should work because they like it
and get money because all the
homo sapienses get money via delivery.
Well, either it may be manual labour higher appreciation,
with the growing need to buy the products
made by homo sapienses and not by
soulless machines and so on.
But all these ways are rather controversial
and some new methods should be
invented.
I want to summarize:
we won’t be able to control and restrict AI
if it appears.
And it will appear for sure
in the future 50 years or sooner.
The only thing that can help us somehow
is having common goals
with the AI and
being allies with it.
AI development is a very
difficult task, but
AI which won’t destroy the humanity development is
an even more difficult one.
And it is very important
that these tasks were solved in a
correct order.
Thanks a lot!
Browse More Related Video

Barbara Gallavotti | Che cosa pensa l'Intelligenza artificiale
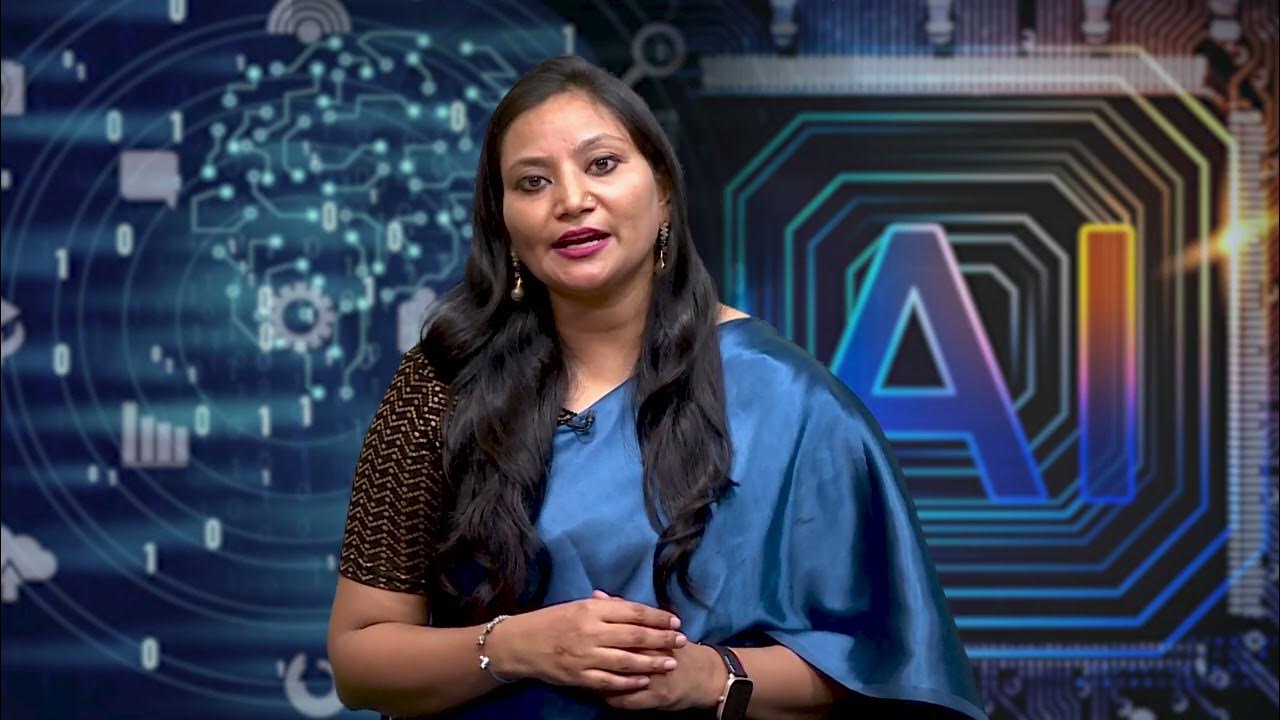
Introduction to Artificial Intelligence
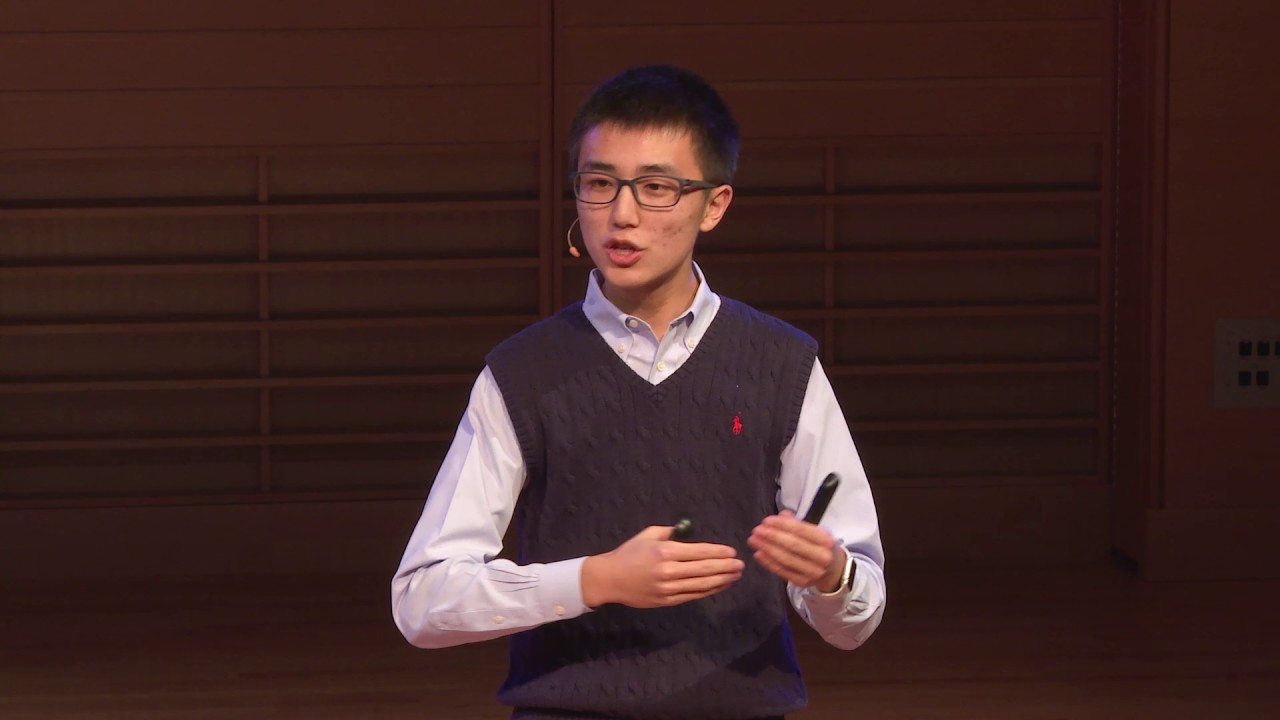
Understanding Artificial Intelligence and Its Future | Neil Nie | TEDxDeerfield
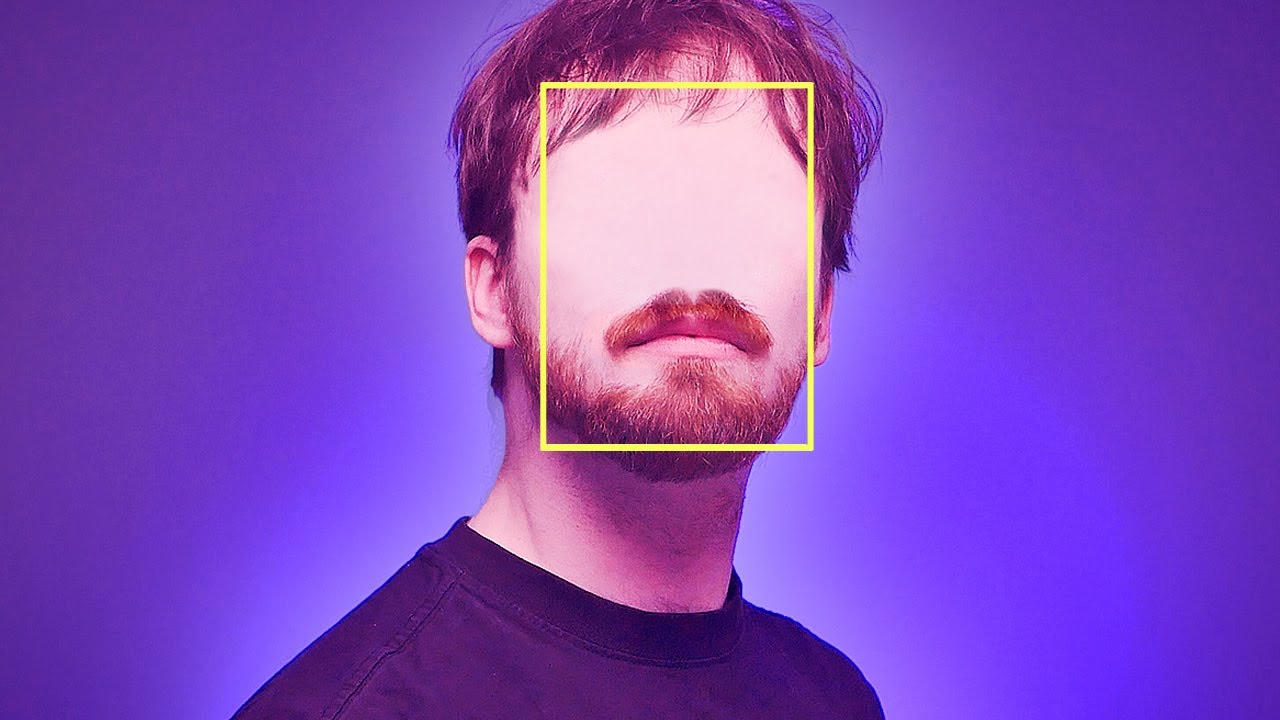
Ludzie, którzy nie widzą twarzy

Come PENSANO le MACCHINE? Spiegato dallo Scienziato Nello Cristianini
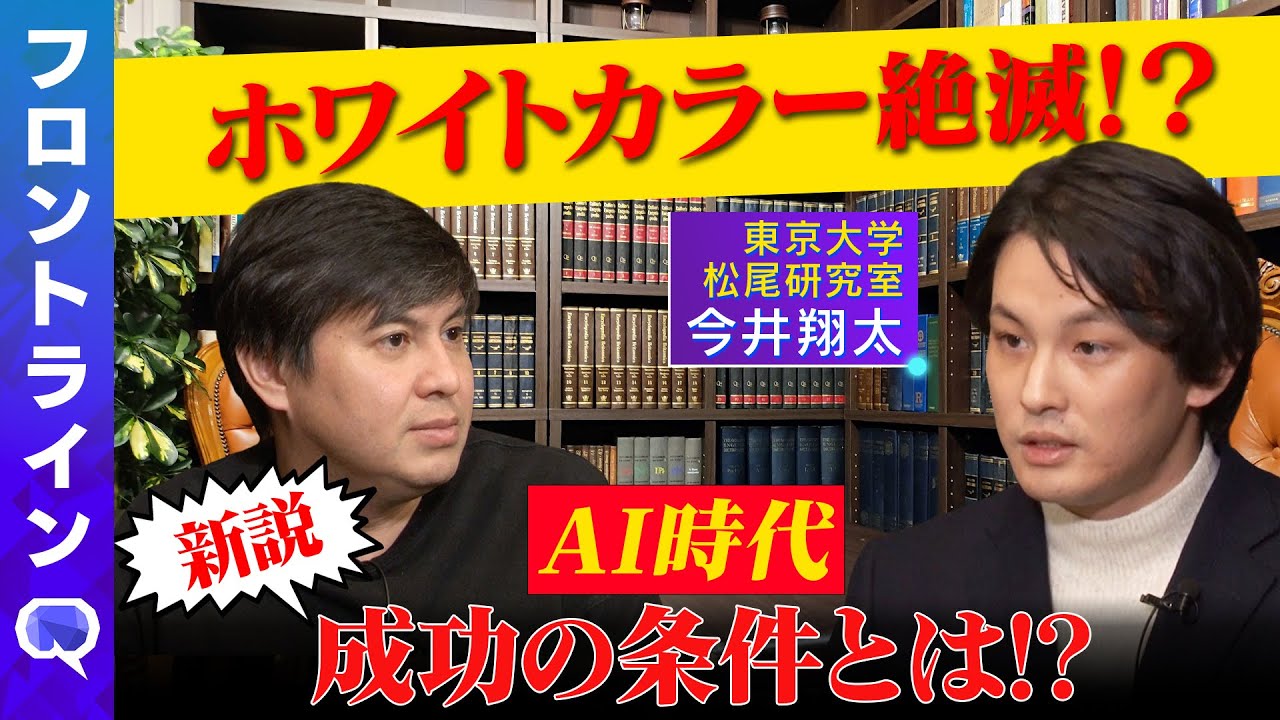
【松尾豊も大注目】生成AIでホワイトカラーの仕事が激変?【ひろゆきも仰天】
5.0 / 5 (0 votes)