Statistics 101: Linear Regression, The Very Basics 📈
Summary
TLDRIn this instructional video, Brandon introduces the concept of simple linear regression, emphasizing its foundational role in statistics. He motivates viewers with encouragement and offers to connect on social media. The video explains that without an independent variable, the best prediction for a dependent variable is its mean. Brandon uses a restaurant tipping scenario to illustrate this, showing how to predict tip amounts based on their average. He also introduces the idea of residuals and the sum of squared residuals (SSE), which is key for assessing the fit of a regression model. The video sets the stage for upcoming discussions on more complex regression analyses.
Takeaways
- 😀 Stay positive and believe in your ability to overcome challenges in learning statistics.
- 📢 Follow the presenter on social media platforms to stay updated with new video releases.
- 👍 Engage with the content by liking, sharing, and providing constructive feedback to help improve future videos.
- 📊 Simple linear regression involves modeling the relationship between two variables: an independent variable and a dependent variable.
- 📈 The 'goodness' of a regression model is determined by how well it compares to a model that assumes no relationship between variables.
- 🔢 In the absence of an independent variable, the mean of the dependent variable is used as the best predictor.
- 📋 The term 'regression' specifically refers to simple linear regression unless mentioned otherwise.
- 📉 Residuals, or the differences between observed values and the mean prediction, are a key concept in understanding regression.
- 🔴 The sum of squared residuals (SSE) is a measure used to evaluate how well the regression line fits the data.
- 📉 The goal of simple linear regression is to find a line that minimizes the SSE, indicating a better fit to the data.
- 📖 The upcoming videos will delve deeper into the concepts and calculations involved in simple linear regression.
Q & A
What is the main theme of the video series?
-The main theme of the video series is basic statistics, with a focus on simple linear regression in this particular video.
What is the first piece of advice given by Brandon to viewers who are struggling in their class?
-The first piece of advice is to stay positive and keep their head up, acknowledging that they have already accomplished a lot and that hard work, practice, and patience will help them through their struggles.
How does Brandon encourage viewers to stay connected with his content?
-Brandon encourages viewers to follow him on various social media platforms like YouTube, Twitter, Google Plus, and LinkedIn to be notified when new videos are uploaded.
What is the significance of liking and sharing the video as mentioned in the script?
-Liking and sharing the video is a way to encourage Brandon to continue making educational content, and it helps to spread the knowledge to classmates, colleagues, or through playlists.
What is the purpose of the 'tips for service' example used in the video?
-The 'tips for service' example is used to illustrate how regression can be used to predict the amount of tip one might expect based on the total bill amount at a restaurant.
What is the dependent variable in the 'tips for service' example?
-In the 'tips for service' example, the dependent variable is the tip amount.
Why does Brandon emphasize the importance of understanding the underlying meaning behind good regression models?
-Brandon emphasizes understanding the underlying meaning behind good regression models to ensure viewers not only know what is happening but also why and how to apply it.
What does Brandon mean when he says 'regression allows us to model mathematically the relationship between two or more variables'?
-This means that regression analysis helps in establishing a mathematical model that describes how one variable (dependent) is related to one or more other variables (independent).
What is the best prediction for the next tip amount if only the tip data is available?
-If only the tip data is available, the best prediction for the next tip amount is the mean of the existing tip amounts.
What is a residual in the context of this video?
-A residual is the difference between the observed value (actual tip amount) and the predicted value (mean of tips or value on the best fit line).
Why are residuals squared in the calculation of the sum of squared residuals?
-Residuals are squared to ensure all values are positive and to emphasize larger deviations from the mean, which helps in calculating the sum of squared residuals (SSE).
What is the goal of simple linear regression as described in the video?
-The goal of simple linear regression is to create a linear model that minimizes the sum of squares of the residuals, which is another way of saying it minimizes the sum of squares of the error.
Outlines
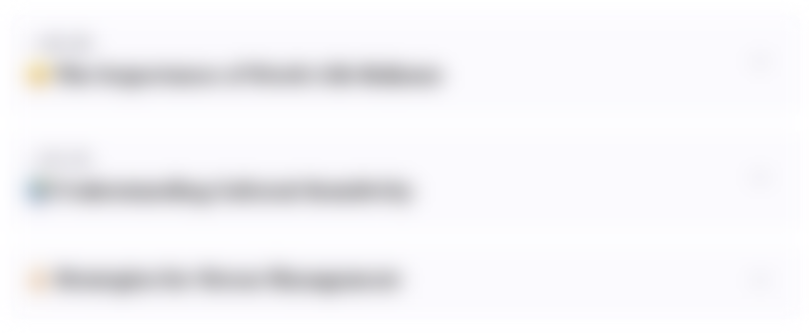
Этот раздел доступен только подписчикам платных тарифов. Пожалуйста, перейдите на платный тариф для доступа.
Перейти на платный тарифMindmap
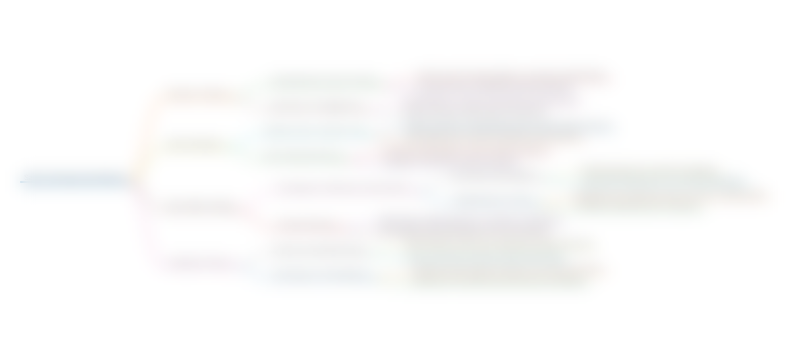
Этот раздел доступен только подписчикам платных тарифов. Пожалуйста, перейдите на платный тариф для доступа.
Перейти на платный тарифKeywords
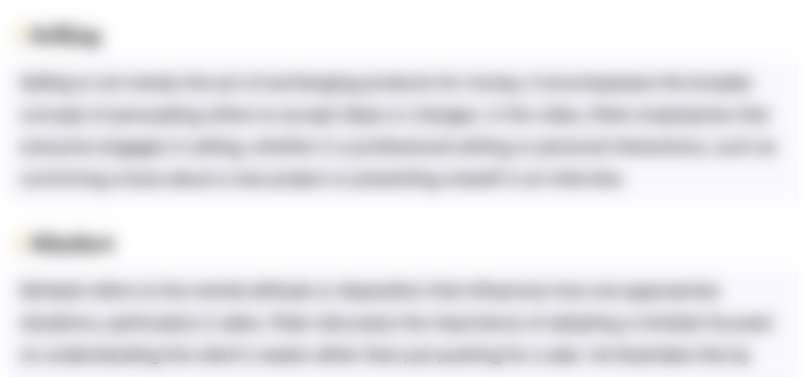
Этот раздел доступен только подписчикам платных тарифов. Пожалуйста, перейдите на платный тариф для доступа.
Перейти на платный тарифHighlights
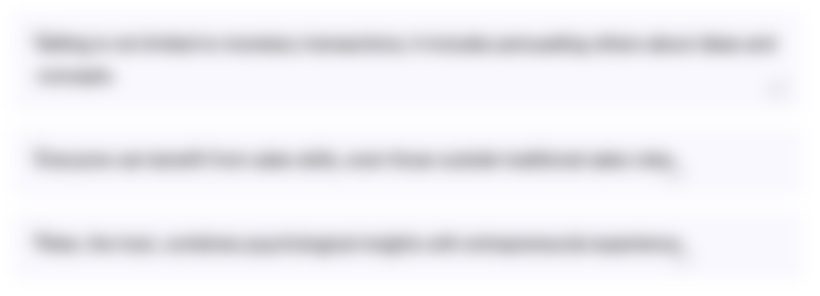
Этот раздел доступен только подписчикам платных тарифов. Пожалуйста, перейдите на платный тариф для доступа.
Перейти на платный тарифTranscripts
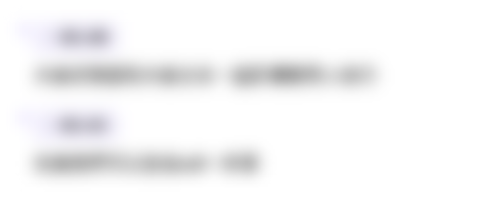
Этот раздел доступен только подписчикам платных тарифов. Пожалуйста, перейдите на платный тариф для доступа.
Перейти на платный тариф5.0 / 5 (0 votes)