Jennifer Golbeck: The curly fry conundrum: Why social media "likes" say more than you might think
Summary
TLDRThe speaker explores how social media transformed the web from a static space to an interactive platform driven by user-generated content. They discuss the vast amount of personal data shared online, often unknowingly, and how predictive models can reveal hidden traits. Using examples like Target's pregnancy score and Facebook 'likes,' the speaker highlights the power of data analysis and the lack of user control. They propose future solutions, including science-driven tools for user empowerment, while acknowledging the challenges of balancing privacy with data usage for online interactions.
Takeaways
- 🌐 The early web was static, with most content created by organizations or tech-savvy individuals.
- 📱 The rise of social media has transformed the web into an interactive platform where average users generate most of the content.
- 👥 Social media has enabled users to create online personas easily, leading to the widespread sharing of personal data.
- 🔍 Large companies, like Target, use behavioral patterns from users' data to predict personal attributes and events, such as pregnancy.
- 📊 Machine learning models can predict hidden user traits, like political preferences, intelligence, and even personality, based on subtle patterns in behavior and online actions.
- 🤖 Likes on platforms like Facebook, such as the example of 'curly fries,' can predict personal attributes due to patterns in social networks.
- 🛑 Users often lack control or understanding of how their data is used and what it reveals about them.
- ⚖️ Solutions to regain user control include policy and law changes, but these are unlikely due to the business models of social media companies and the slow political process.
- 🔐 Technological solutions, such as data encryption and transparent risk warnings, could give users more control over their data sharing decisions.
- 🔬 Scientists aim to empower users with tools that help them make informed choices about their data, focusing on improving online interactions rather than exploiting personal information.
Q & A
How did the web evolve from the first decade to the era of social media?
-In the first decade, the web was mostly static, with content created by organizations or tech-savvy individuals. The rise of social media in the early 2000s transformed the web into an interactive space where average users contribute content through platforms like YouTube, blogs, and social media posts.
What is homophily, and how does it relate to predicting traits online?
-Homophily is a sociological theory that suggests people are friends with others who are like them. In the context of online behavior, it helps explain how people with similar attributes, like intelligence, cluster together, allowing models to predict traits based on patterns of behavior.
What example is given to illustrate how companies predict personal traits based on seemingly irrelevant data?
-An example is Target predicting a teenage girl's pregnancy before she informed her parents. By analyzing her purchase history, including buying more vitamins or a large handbag, Target computed a 'pregnancy score' and used it to predict her due date.
How does liking something like curly fries on Facebook relate to predicting intelligence?
-Liking the curly fries page is indicative of high intelligence, not because of the content itself, but due to homophily. A smart person may have initially liked the page, and through their network of similarly smart friends, this action propagated, making it a signal of intelligence.
Why do companies like Facebook hold so much data about users, and what are the concerns around this?
-Social media companies rely on user data as their primary asset for generating revenue. The concern is that users often don't understand how their data is used or shared, and they have little control over it. This leads to privacy risks and potential misuse of personal information.
What are the potential dangers of using predictive models on social media data?
-These models can predict sensitive personal attributes, such as political preferences, sexual orientation, and drug use, without users' knowledge or consent. Such information could be exploited for non-altruistic purposes, like by employers making hiring decisions based on these predictions.
What challenges exist in addressing user control over their personal data?
-There are two primary challenges: the slow political process in enacting laws to protect data rights and the reliance of social media companies on user data for revenue. This makes it unlikely for companies to voluntarily give users full control over their data.
How can science help users regain control over their online data?
-Research can develop tools that inform users about the risks of their actions, such as liking a page or sharing information. This could help users make informed decisions about what data they share. Scientists could also work on encrypting data to protect user privacy.
What is the speaker’s perspective on balancing data prediction models and user control?
-The speaker believes that users should have control over how their data is used. Although predictive models are useful for enhancing online interactions, users should have the option to prevent data collection if they wish, even if that diminishes the models' effectiveness.
Why does the speaker think law reform on user data control is unlikely in the near future?
-The speaker observes that the political process is slow and unlikely to prioritize or effectively address the complexities of data privacy laws. Additionally, social media companies have a vested interest in maintaining control over user data for profit.
Outlines
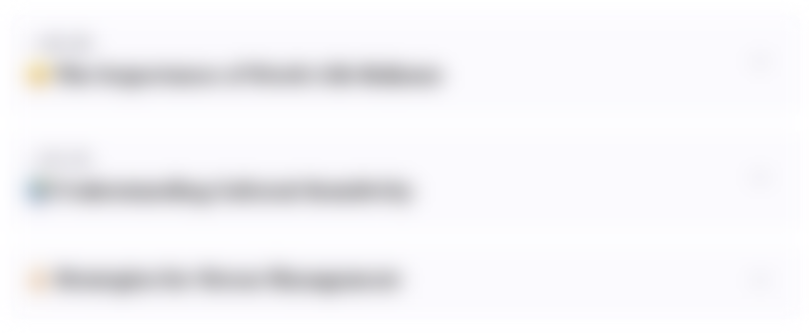
Этот раздел доступен только подписчикам платных тарифов. Пожалуйста, перейдите на платный тариф для доступа.
Перейти на платный тарифMindmap
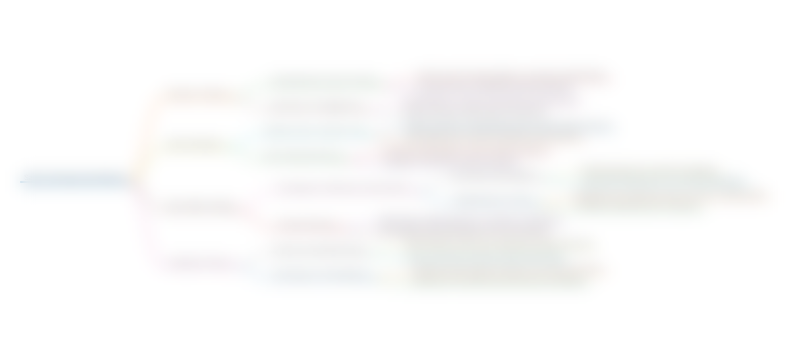
Этот раздел доступен только подписчикам платных тарифов. Пожалуйста, перейдите на платный тариф для доступа.
Перейти на платный тарифKeywords
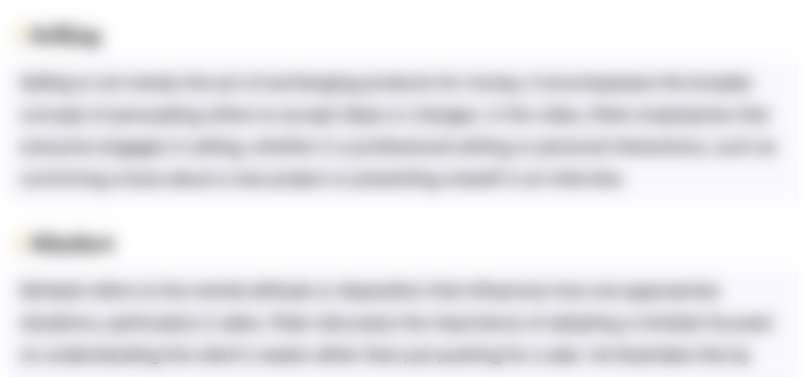
Этот раздел доступен только подписчикам платных тарифов. Пожалуйста, перейдите на платный тариф для доступа.
Перейти на платный тарифHighlights
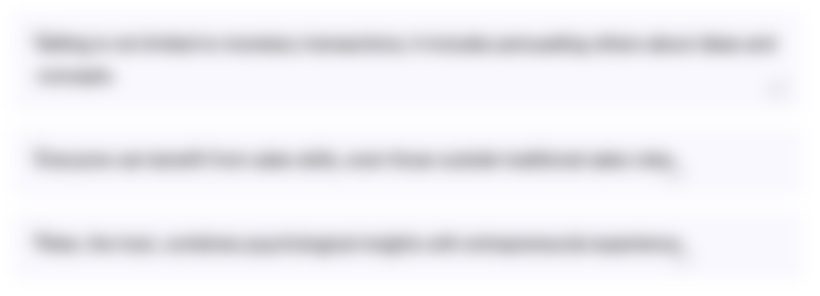
Этот раздел доступен только подписчикам платных тарифов. Пожалуйста, перейдите на платный тариф для доступа.
Перейти на платный тарифTranscripts
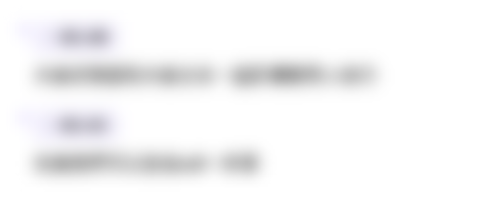
Этот раздел доступен только подписчикам платных тарифов. Пожалуйста, перейдите на платный тариф для доступа.
Перейти на платный тариф5.0 / 5 (0 votes)