15x4 - 15 минут про Искусственный Интеллект
Summary
TLDRАлександр рассказывает о различных подходах к созданию искусственного интеллекта (ИИ), разделяя их на нисходящий и восходящий подходы. Он обсуждает разницу между сильным и слабым ИИ, а также вопросы, связанные с определением интеллекта и сознания в машинах, ссылаясь на тест Тьюринга. Александр затрагивает тему обучения и способности предсказания как основных компонентов интеллекта, размышляя о будущем ИИ и его влиянии на человечество, подчеркивая важность совместных целей между ИИ и людьми для избежания потенциальных угроз.
Takeaways
- 😀 Есть два основных подхода к созданию искусственного интеллекта: снизу вверх, изучая интеллект, и сверху вниз, изучая поведение.
- 🤖 Тест Тьюринга предлагает метод верификации интеллекта машины через игру в имитацию, где машина должна запутать судью.
- 🧠 Искусственный интеллект делится на сильный и слабый, причём реально существующие системы пока не достигли уровня сильного ИИ.
- 📚 Человеческий интеллект включает способность анализировать внешний мир, обучаться и генерировать рациональное поведение.
- 🐊 Примеры поведения животных, такие как крокодилы и мыши, демонстрируют различные уровни интеллектуального поведения и памяти.
- 🔬 Нейрокортекс млекопитающих играет ключевую роль в высших интеллектуальных функциях, включая память и принятие решений.
- 💡 Глубокое обучение и нейронные сети вдохновлены структурой и функциями человеческого мозга, что привело к значительным успехам в ИИ.
- 👁 ИИ превосходит человека в некоторых задачах, включая распознавание образов, благодаря быстрому развитию и обучению на больших данных.
- 🚀 Технологический прогресс и развитие ИИ могут привести к моменту, когда искусственный интеллект превзойдёт человеческий по всем параметрам.
- 🌍 Перед обществом стоит задача адаптации к миру, где ИИ выполняет большинство работ, требующихся для поддержания современного образа жизни.
Q & A
Что такое искусственный интеллект?
-Искусственный интеллект - это раздел компьютерных наук, который занимается созданием программ или машин, способных выполнять задачи, требующие человеческого интеллекта, такие как обучение, понимание языка и решение проблем.
Какие существуют подходы к созданию искусственного интеллекта?
-Существуют два основных подхода: bottom-up (снизу вверх), который изучает внутреннюю структуру объекта с интеллектом (например, человека) для имитации интеллектуального поведения, и top-down (сверху вниз), который сосредотачивается на изучении самого интеллектуального поведения и разработке систем, способных его воспроизводить.
Чем отличается сильный искусственный интеллект от слабого?
-Слабый искусственный интеллект способен выполнять конкретные задачи в рамках определенной области, в то время как сильный ИИ обладает общим интеллектом, сравнимым с человеческим, и может решать задачи в различных областях.
Какова основная идея теста Тьюринга?
-Основная идея теста Тьюринга заключается в том, что если машина способна убедить человека в том, что она не отличается от другого человека, отвечая на вопросы через текстовый чат, то машина может считаться обладающей интеллектом.
Почему поведение не всегда является показателем интеллекта?
-Поведение может быть сложным и разнообразным даже у существ без развитого интеллекта, как, например, у крокодилов. Наличие сложного поведения не обязательно указывает на способность к планированию или размышлению, которые являются ключевыми аспектами интеллекта.
Что такое обучение в контексте искусственного интеллекта?
-Обучение в контексте искусственного интеллекта - это процесс, в ходе которого машина или программа улучшает свои способности к решению задач или адаптации к новым условиям на основе предыдущего опыта или данных.
Какие методы используются для оценки детского интеллекта?
-Для оценки детского интеллекта используются различные задачи, например, тесты на сообразительность, в которых детям предлагается дополнить последовательность слов или изображений, либо решить логические головоломки.
Что такое глубокое обучение?
-Глубокое обучение - это подмножество методов машинного обучения, использующее многослойные нейронные сети для анализа больших объемов данных, что позволяет системам обучаться, распознавать образы и делать выводы на уровне, сравнимом с человеческим.
Почему искусственный интеллект считается менее развитым, чем интеллект мыши?
-На момент речи искусственный интеллект считается менее развитым, чем интеллект мыши, потому что ИИ еще не способен к такому же уровню принятия решений, обучения и адаптации к изменяющимся условиям, как живые существа с развитым мозгом.
Как изменится общество с развитием искусственного интеллекта?
-С развитием искусственного интеллекта многие задачи, традиционно выполняемые людьми, будут автоматизированы, что может привести к изменениям в структуре трудового рынка, требованиях к образованию и социальной организации общества.
Outlines
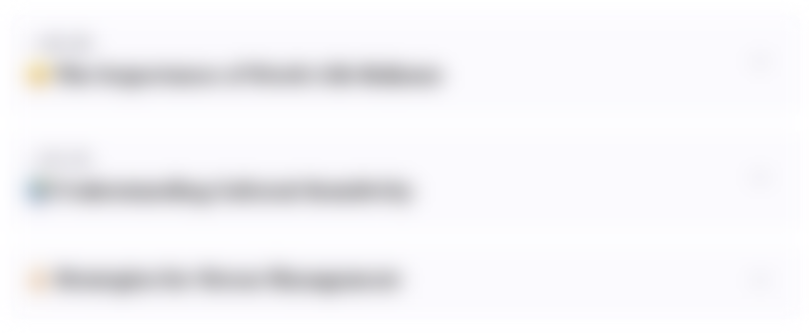
Этот раздел доступен только подписчикам платных тарифов. Пожалуйста, перейдите на платный тариф для доступа.
Перейти на платный тарифMindmap
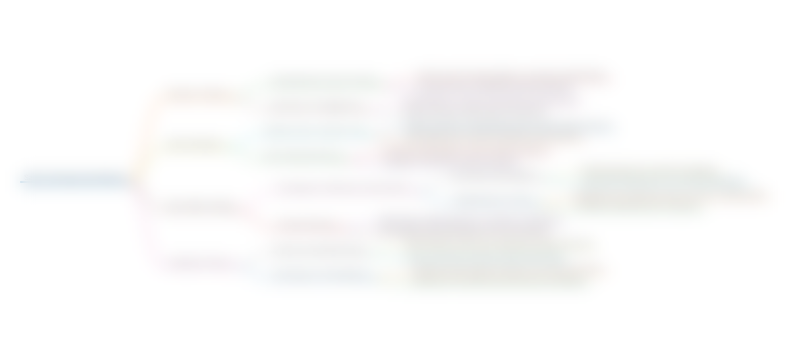
Этот раздел доступен только подписчикам платных тарифов. Пожалуйста, перейдите на платный тариф для доступа.
Перейти на платный тарифKeywords
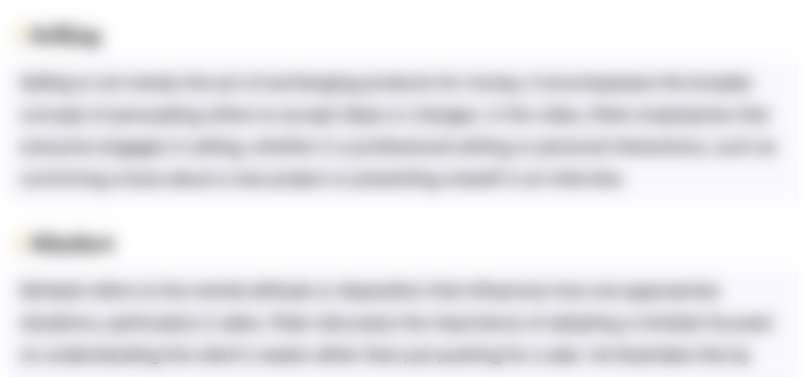
Этот раздел доступен только подписчикам платных тарифов. Пожалуйста, перейдите на платный тариф для доступа.
Перейти на платный тарифHighlights
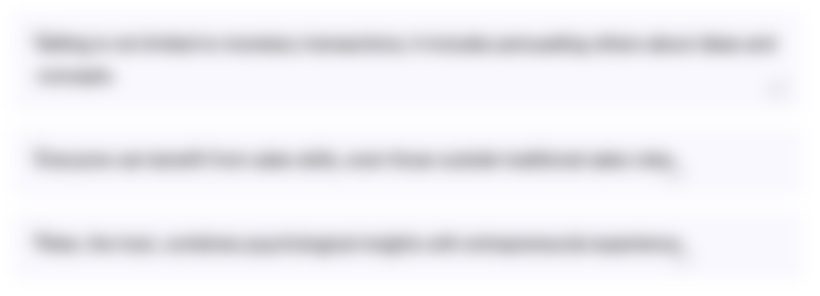
Этот раздел доступен только подписчикам платных тарифов. Пожалуйста, перейдите на платный тариф для доступа.
Перейти на платный тарифTranscripts
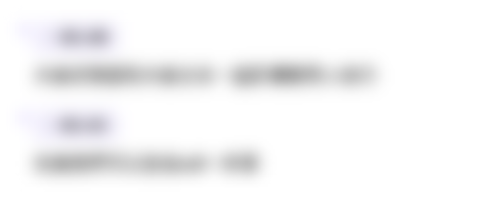
Этот раздел доступен только подписчикам платных тарифов. Пожалуйста, перейдите на платный тариф для доступа.
Перейти на платный тарифПосмотреть больше похожих видео
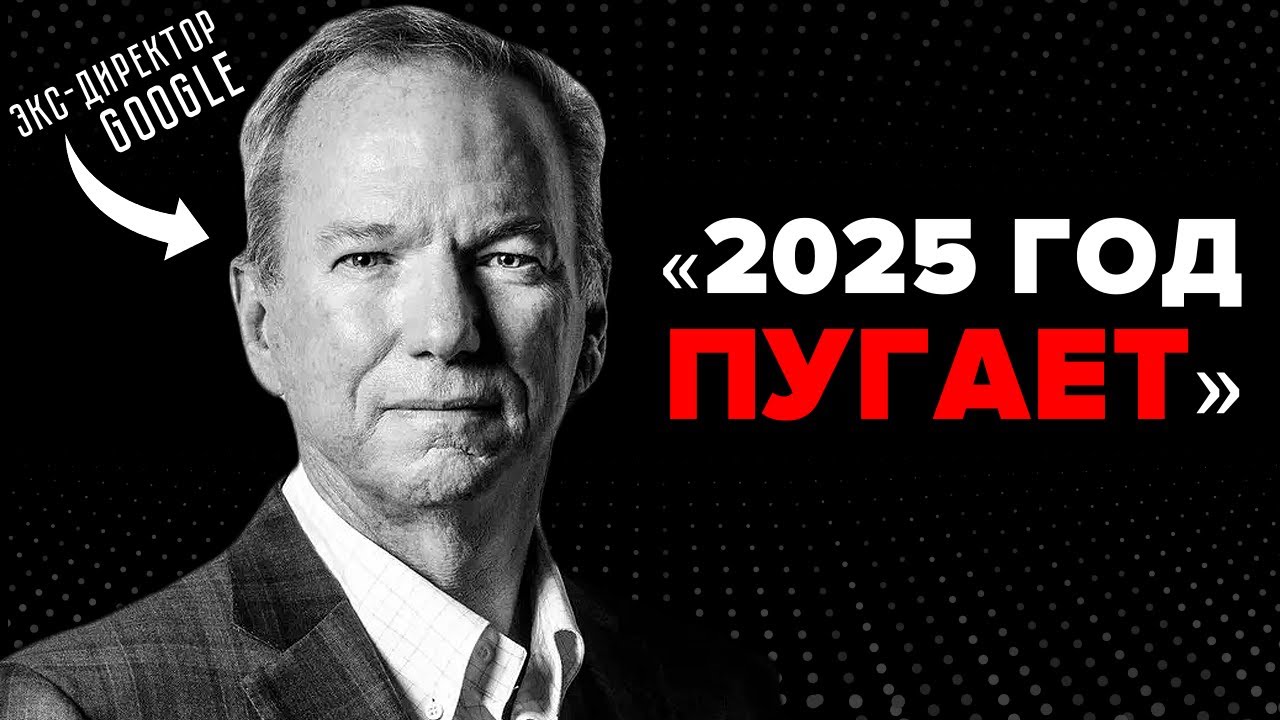
ЗАПРЕЩЕННОЕ ИНТЕРВЬЮ бывшего ген Директора Google: «Вы даже не представляете, что вас ждет»

Илья Суцкевер . Увлекательный и опасный путь к Общему ИИ (AGI). Дублированный перевод

Microsoft CEO Satya Nadella on the Future of AI

2 1 Обзор языковых моделей
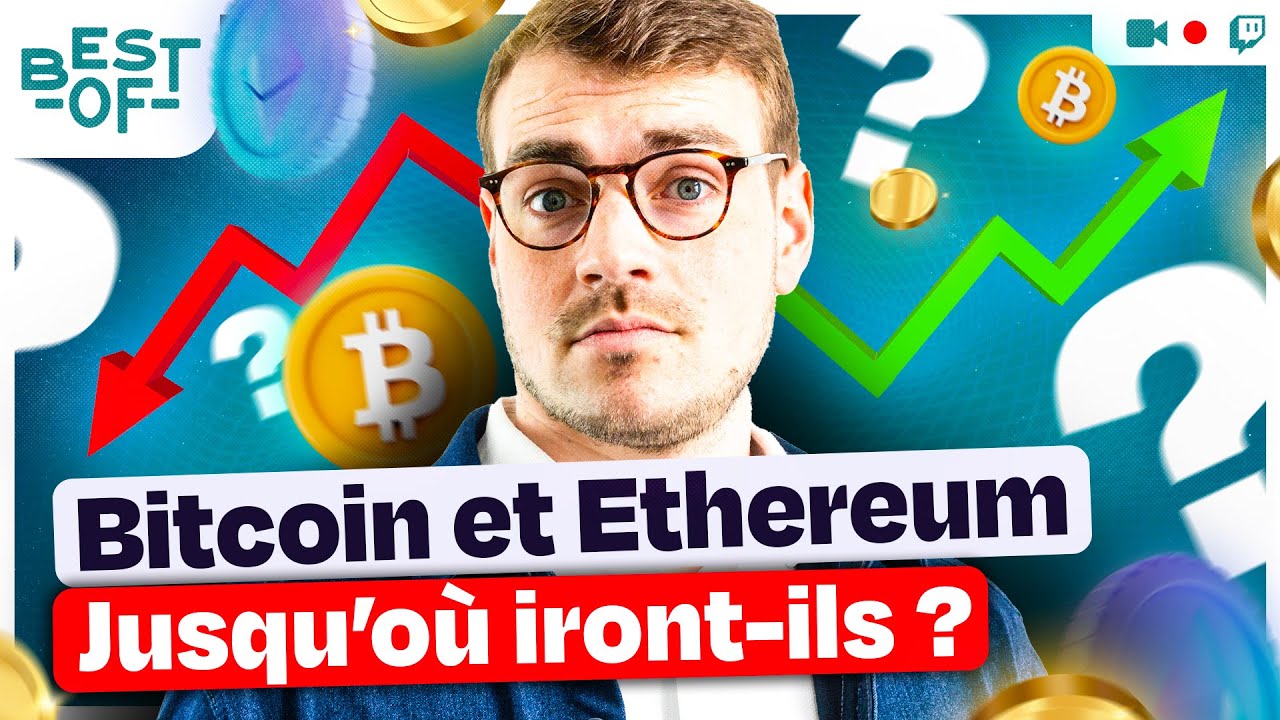
Le futur prix de Bitcoin et Ethereum ? 250K en 2025 ? | Hasheur Live #BestOf

Де взяти ідею для свого стартапу? Три джерела

Европа в сфере ИИ бросает вызов технологическим гигантам из США
5.0 / 5 (0 votes)