Responsible Data Management – Julia Stoyanovich
Summary
TLDRIn this engaging talk, the speaker discusses the complexities of responsible data management and AI, particularly in hiring practices. They define AI as systems that assist in decision-making and highlight the potential for biases—pre-existing, technical, and emerging—that can exacerbate societal inequalities. By illustrating the consequences of AI errors, from trivial to catastrophic, the speaker emphasizes the importance of rigorous validation of AI tools. The necessity for human oversight in addressing bias and ensuring fairness is stressed, along with an invitation to explore resources aimed at demystifying these critical issues in AI.
Takeaways
- 😀 AI is defined as a system where algorithms utilize data to aid decision-making for humans.
- 🤖 There is a significant promise in AI applications, but mistakes made by AI can lead to varying consequences.
- 📦 Simple AI mistakes, like shipping the wrong shoes, have minor consequences, while mistakes in autonomous vehicles can be catastrophic.
- 💼 AI is increasingly involved in hiring processes, yet it often replicates existing biases in recruitment.
- 🚫 Despite AI's potential, it can exacerbate biases based on race, gender, and disability during hiring.
- 🔍 Researchers must assess whether AI tools genuinely help achieve diversity and fairness in hiring.
- 📊 A recent study found that AI personality profiling tools may yield inconsistent results based on factors like file format.
- ⚖️ Three types of bias in AI are identified: pre-existing, technical, and emerging bias, each affecting AI's effectiveness.
- 📉 Data serves as a reflection of reality; thus, biases in data often mirror societal issues rather than providing an unbiased view.
- 👥 It is up to people, not algorithms, to determine the ideal state of the world and to work towards change.
Q & A
What is the speaker's definition of AI?
-The speaker defines AI as a system in which algorithms use data to make decisions on our behalf or assist humans in making decisions.
What are some examples of AI mentioned in the talk?
-Examples of AI mentioned include a smart vacuum (Roomba), chess-playing AI, automated hiring systems, and autonomous cars.
What is the significance of the mistakes made by AI systems?
-Mistakes made by AI systems can range from minor issues, like shipping the wrong shoes, to catastrophic failures, such as accidents involving autonomous cars, which can lead to severe consequences including loss of life.
How does AI impact hiring practices according to the speaker?
-AI can exacerbate bias in hiring practices, as it often reflects existing societal biases. The speaker mentions that humans tend to favor candidates who resemble themselves, which AI can reinforce.
What does the speaker suggest about the stability of AI predictions?
-The speaker highlights that the stability of AI predictions can vary significantly based on factors such as file format, suggesting that if an AI tool cannot consistently provide the same output for the same input, its reliability is questionable.
What types of biases are discussed in the talk?
-The speaker discusses three types of biases: pre-existing bias (originating from society), technical bias (introduced by the technical properties of a system), and emerging bias (arising from the context of use).
How does the speaker view the relationship between data and the real world?
-The speaker argues that data acts as a mirror reflecting the world. However, this reflection can be distorted, and it is crucial to understand whether it accurately represents reality or perpetuates existing biases.
What are the implications of bias in AI systems for society?
-Bias in AI systems can lead to systemic issues that negatively impact individuals and groups, particularly in crucial areas like hiring, where unfair algorithms can perpetuate discrimination and inequality.
What role does regulation play in responsible AI according to the speaker?
-The speaker notes that there is a growing push for regulations to oversee AI use, particularly in hiring practices, to ensure fairness and accountability in AI systems.
What did the speaker's recent research involve regarding AI and personality prediction?
-The speaker's research involved testing commercial AI tools that claim to predict personality profiles based on resumes. They found that the outputs varied significantly based on simple changes, like file format, raising concerns about the tools' validity.
Outlines
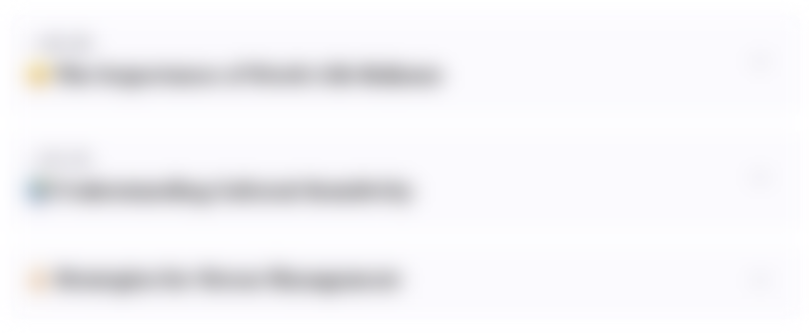
このセクションは有料ユーザー限定です。 アクセスするには、アップグレードをお願いします。
今すぐアップグレードMindmap
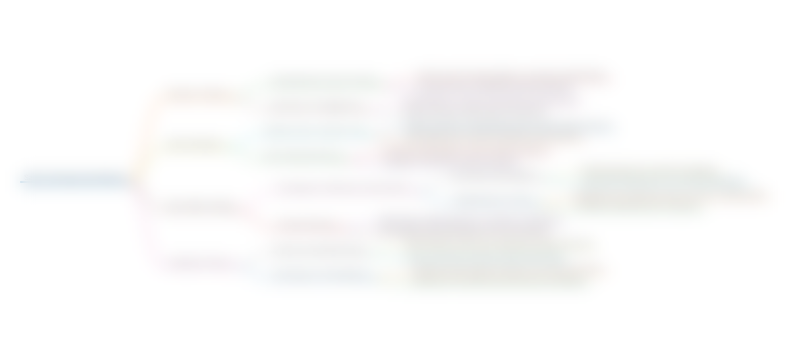
このセクションは有料ユーザー限定です。 アクセスするには、アップグレードをお願いします。
今すぐアップグレードKeywords
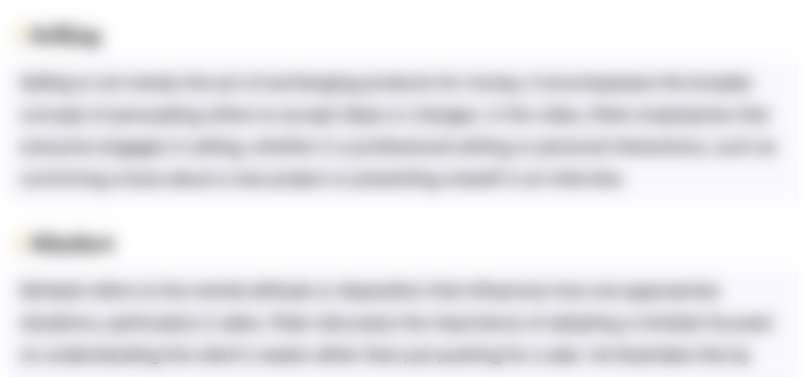
このセクションは有料ユーザー限定です。 アクセスするには、アップグレードをお願いします。
今すぐアップグレードHighlights
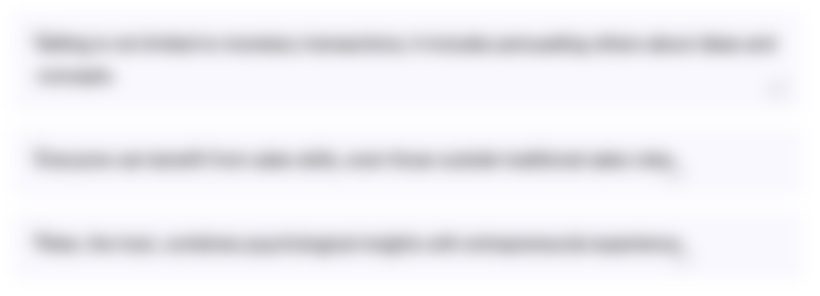
このセクションは有料ユーザー限定です。 アクセスするには、アップグレードをお願いします。
今すぐアップグレードTranscripts
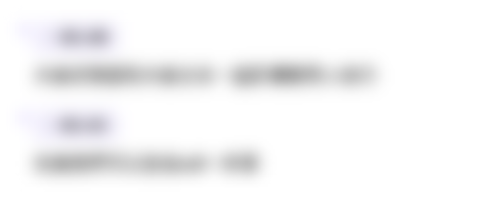
このセクションは有料ユーザー限定です。 アクセスするには、アップグレードをお願いします。
今すぐアップグレード5.0 / 5 (0 votes)