Regresi Ordinal dan Multinomial Logistik Pada Data Crosssection dengan Minitab
Summary
TLDRThis video provides an in-depth explanation of ordinal logistic regression on cross-sectional data using Minitab software. The presenter discusses how this regression model is applied when the dependent variable has more than two ordered categories, such as low, medium, and high scores. The tutorial covers model fitting, interpreting results, and evaluating significance of variables like social scores and gender. Additionally, the video compares ordinal regression with multinomial logistic regression, explaining the difference in handling nominal variables. It's an informative guide for understanding regression techniques in statistical analysis.
Takeaways
- 📊 The video discusses ordinal logistic regression for cross-sectional data analysis using Minitab.
- 📝 Ordinal logistic regression is used when the dependent variable consists of more than two ordered categories.
- 📈 The example used examines the impact of social score and gender on different levels of a dependent variable.
- 🧑💻 Minitab is used to input the dataset and run the regression, with 'Ordinal Regression' selected for analysis.
- 💡 The dependent variable in the example is ordered in three categories: low, medium, and high.
- ✔️ The model is tested for significance, showing whether it is a good fit or not using p-values.
- ♀️ Gender is found to have a significant impact, where females are more likely to fall into the first category compared to males.
- 👨🏫 Social score has a positive influence, meaning a higher score is associated with higher categories.
- 🔄 The script contrasts ordinal regression with multinomial logistic regression, which deals with nominal variables (unordered).
- 🔍 The multinomial regression example looks at the influence of math and science scores on students' program selection (e.g., vocational, general, academic).
Q & A
What is ordinal logistic regression?
-Ordinal logistic regression is a statistical method used when the dependent variable is categorical and ordered, with more than two categories. It models the relationship between predictor variables and an ordinal outcome, where the categories have a meaningful order but no consistent distance between them.
In what type of scenarios is ordinal logistic regression used?
-Ordinal logistic regression is used when you want to analyze the relationship between predictors and an ordinal outcome variable. For example, you might use it to assess how factors like social score and gender influence the ranking of satisfaction levels (low, medium, high).
What kind of data is suitable for ordinal logistic regression?
-The dependent variable in ordinal logistic regression should be qualitative and ordinal, meaning it has more than two ordered categories (e.g., low, medium, high). The independent variables can be continuous or categorical.
How does Minitab help in performing ordinal logistic regression?
-In Minitab, ordinal logistic regression can be executed by selecting the 'Regression' menu and choosing 'Ordinal Logistic Regression.' You specify the dependent variable (response) and independent variables (predictors), then choose options like link functions (logit, probit, etc.) to complete the analysis.
What does a significant result in an ordinal logistic regression imply?
-A significant result in ordinal logistic regression means that at least one of the predictor variables has a statistically significant impact on the ordinal outcome variable, indicating that the predictors are associated with changes in the category levels of the dependent variable.
What is multinomial logistic regression and how is it different from ordinal logistic regression?
-Multinomial logistic regression is used when the dependent variable is categorical with more than two categories, but these categories are not ordered. It differs from ordinal logistic regression, where the outcome variable has an inherent order (e.g., low, medium, high).
When would you use multinomial logistic regression?
-You would use multinomial logistic regression when your dependent variable is nominal and cannot be ordered, such as when evaluating students' preferences for different study programs (e.g., general, vocational, academic) without assuming any ranking among the options.
What statistical tests are used to evaluate the fit of the ordinal logistic regression model?
-Two key tests are used: the model deviance test, which checks the overall fit of the model (whether it is statistically significant), and the likelihood ratio test, which evaluates whether at least one of the predictor variables has a significant effect on the outcome variable.
What does it mean when the variable 'female' has a positive coefficient in the model?
-A positive coefficient for the variable 'female' in the model indicates that females are more likely to be in a higher category of the ordinal dependent variable compared to males. For example, if the dependent variable is satisfaction level, females are more likely to have a higher satisfaction level.
How does ordinal logistic regression handle multiple categories in the dependent variable?
-Ordinal logistic regression models the probability of the outcome being in one category versus all lower categories. For instance, if the dependent variable has three categories, the model would compute the probability of being in category 2 or 3 versus category 1, and separately the probability of being in category 3 versus categories 1 and 2.
Outlines
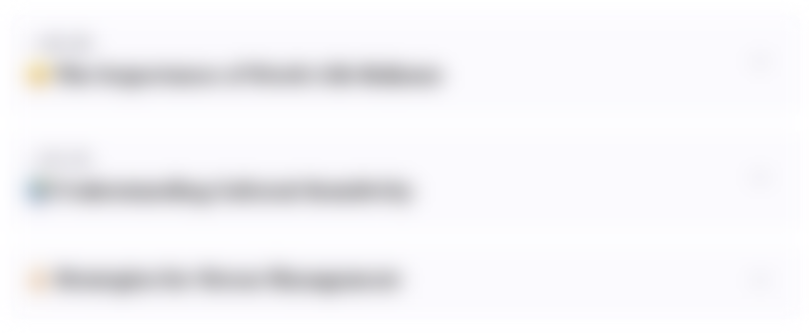
このセクションは有料ユーザー限定です。 アクセスするには、アップグレードをお願いします。
今すぐアップグレードMindmap
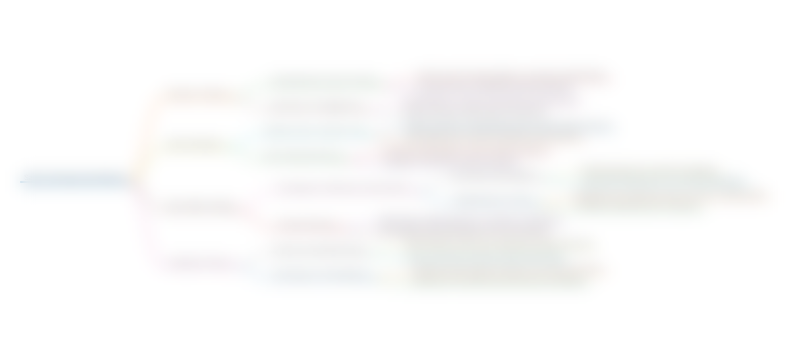
このセクションは有料ユーザー限定です。 アクセスするには、アップグレードをお願いします。
今すぐアップグレードKeywords
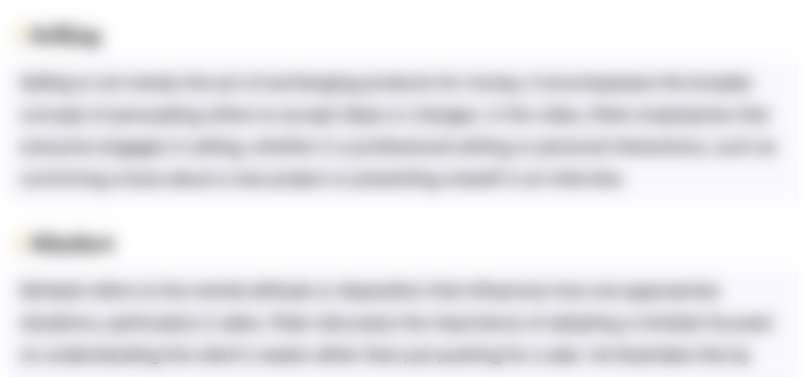
このセクションは有料ユーザー限定です。 アクセスするには、アップグレードをお願いします。
今すぐアップグレードHighlights
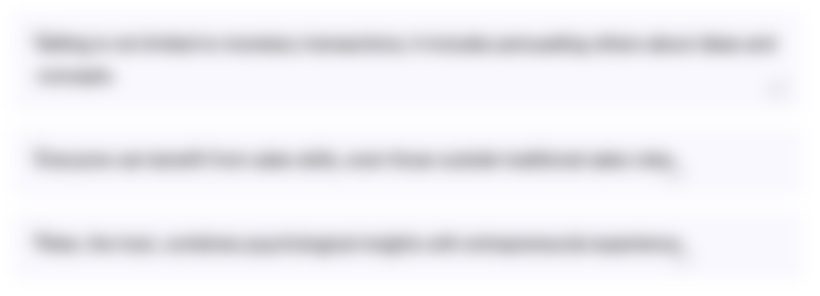
このセクションは有料ユーザー限定です。 アクセスするには、アップグレードをお願いします。
今すぐアップグレードTranscripts
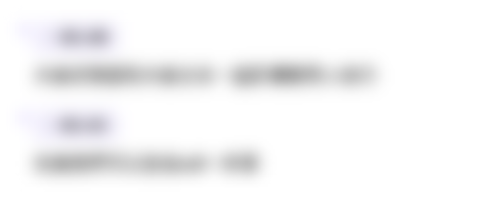
このセクションは有料ユーザー限定です。 アクセスするには、アップグレードをお願いします。
今すぐアップグレード関連動画をさらに表示
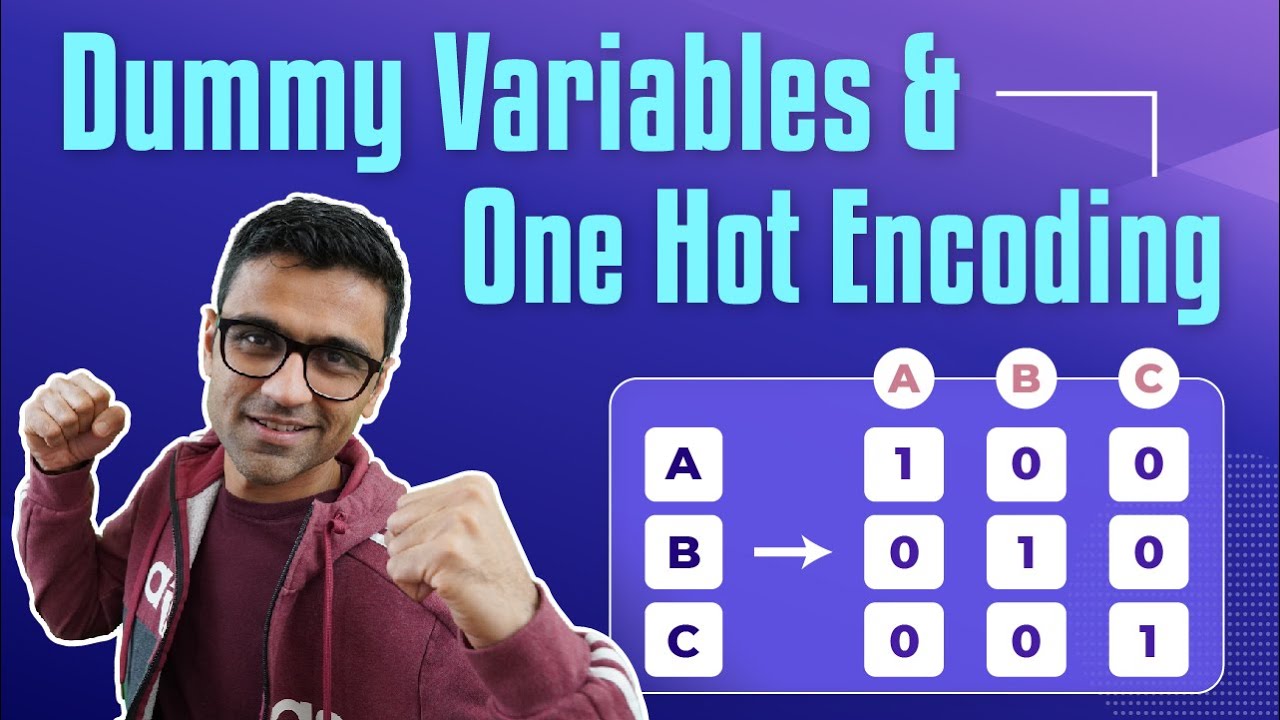
Machine Learning Tutorial Python - 6: Dummy Variables & One Hot Encoding
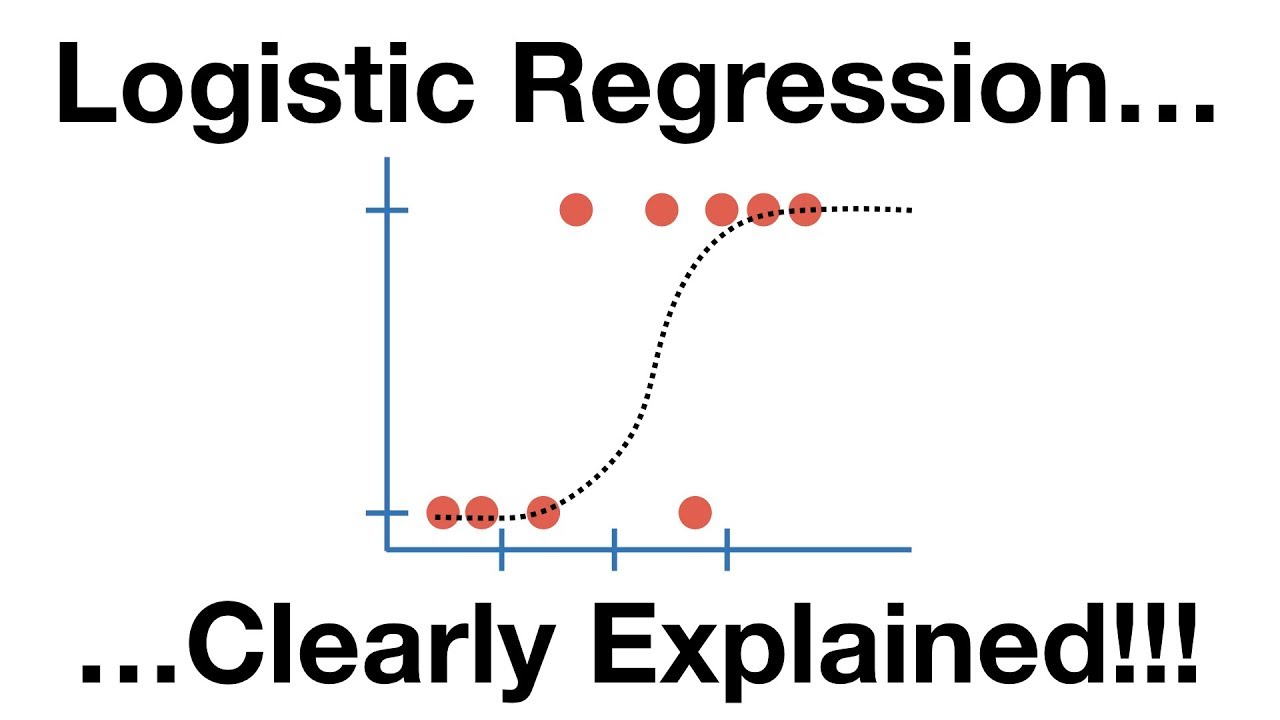
StatQuest: Logistic Regression
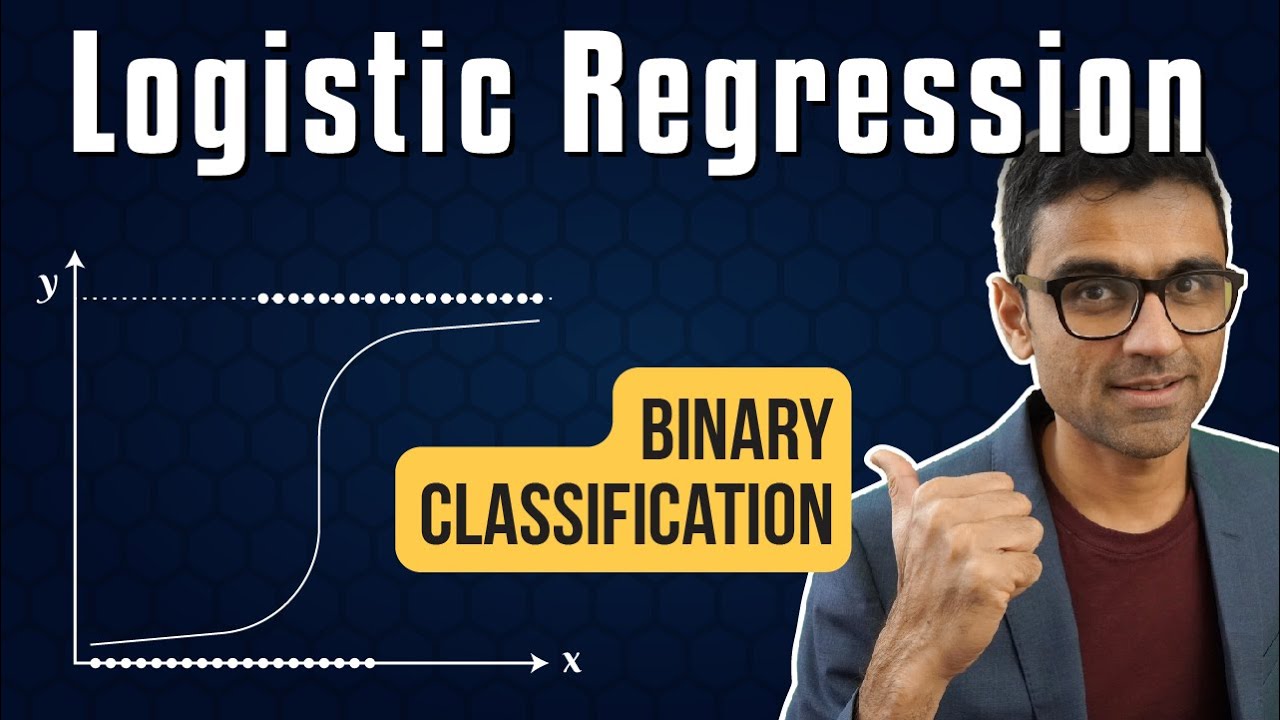
Machine Learning Tutorial Python - 8: Logistic Regression (Binary Classification)
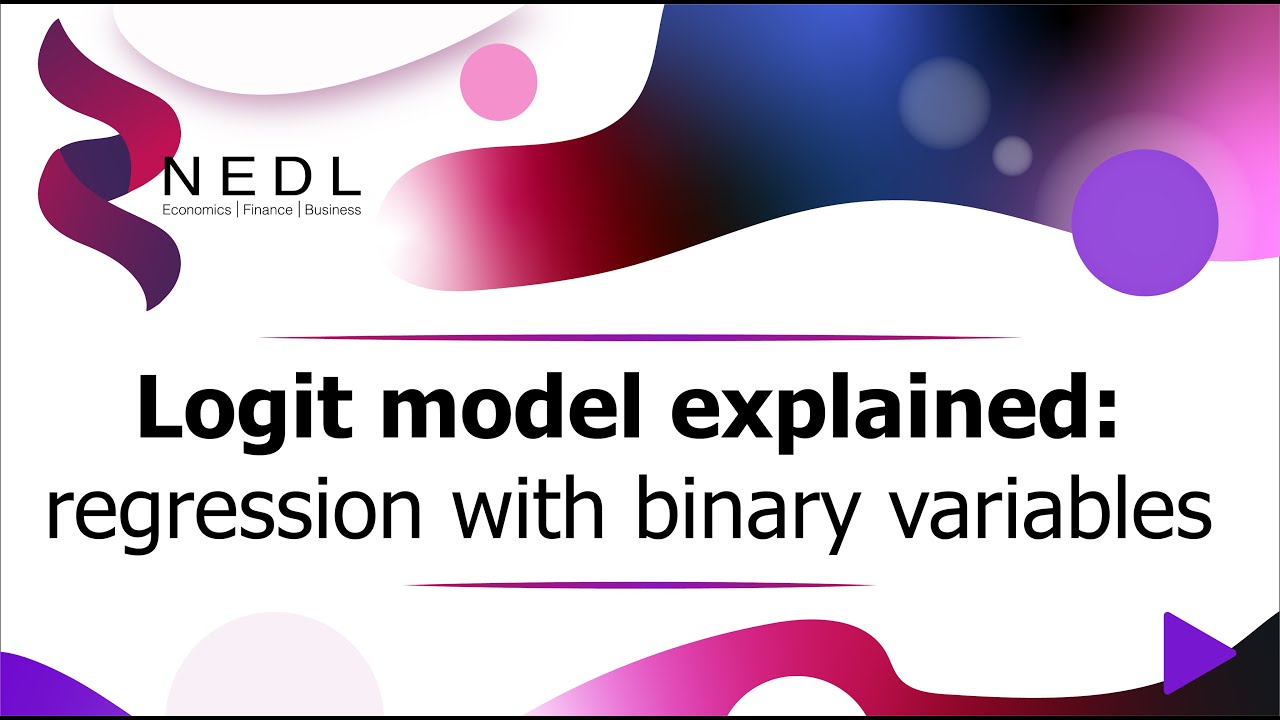
Logit model explained: regression with binary variables (Excel)

Using Multiple Regression in Excel for Predictive Analysis
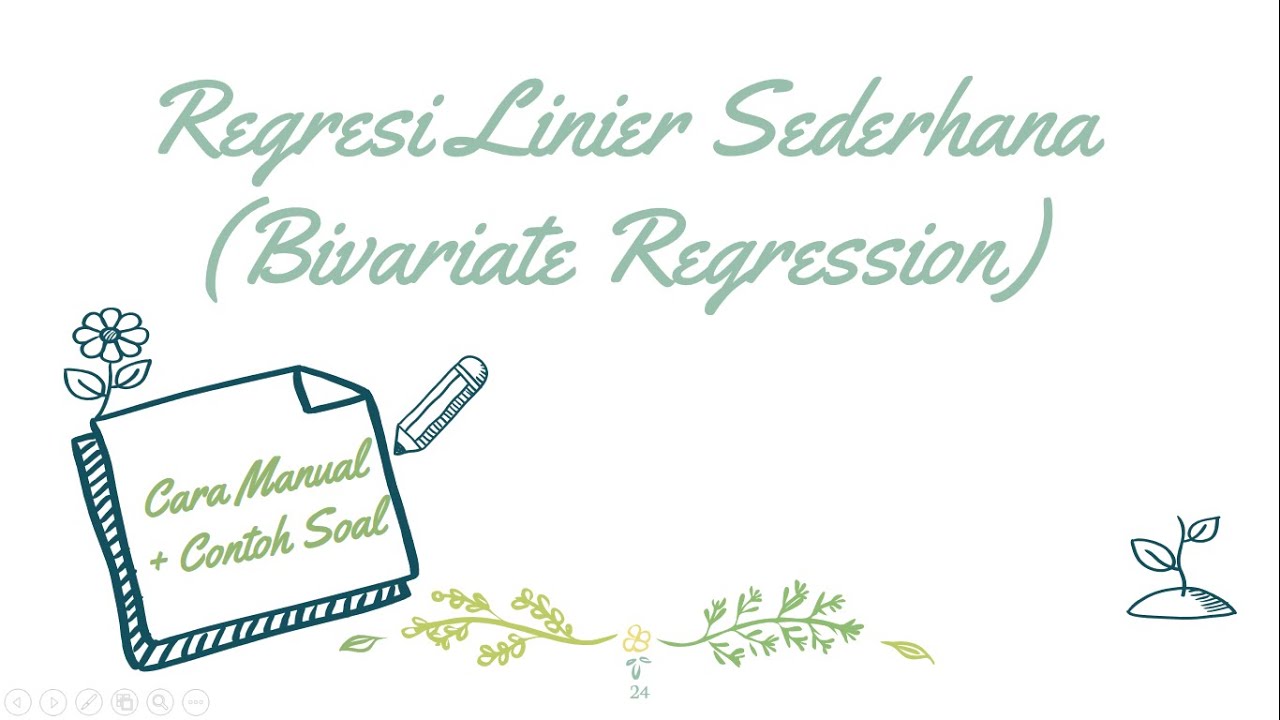
STATISTIKA - Regresi Linier Sederhana Cara Manual + Contoh Soal
5.0 / 5 (0 votes)