OpenAI's 'AGI Robot' Develops SHOCKING NEW ABILITIES | Sam Altman Gives Figure 01 Get a Brain
Summary
TLDRフィギュアAIとOpenAIが協力して開発したロボット「フィギュア01」が注目されています。このロボットは、視覚と言語を理解する高水準のニューラルネットワークを搭載し、人とフルコンバーサーションを行うことができます。ロボットは、テーブル上の食べ物を認識し、ゴミを片付けたり、食器を乾燥架に移動させたりする動揺をとることができます。全ての動作はニューラルネットワークによって学習され、人間の指示に従って自然に動くことができます。
Takeaways
- 🤖 Figure AIとOpenAIが協力し、ロボット工学とニューラルネットワークの専門知識を組み合わせた。
- 🔧 ロボットは金属とニューラルネットワークの統合体であり、視覚と言語の高度な機能を備えている。
- 🍎 Figure Oneは、与えられたシーンに基づいて、食べ物を選定し、清理を行うことができる。
- 🗑️ ロボットは、テーブル上の汚れ物を適切な場所に移動させることができ、 multitaskingも可能だ。
- 🌐 すべての動作はニューラルネットワークによって学習され、事前にプログラミングされていない。
- 📚 Figure AIは独自に開発された技術を用いていて、詳細は公開されていない可能性がある。
- 🤔 ロボットは、Google DeepMindのRT2ロボットと比較されるが、学習方法が異なる可能性がある。
- 💬 Figure Oneは、会話の履歴を処理し、短期記憶を持つことで、一般的な要求に応答することができる。
- 🔄 ロボットは、視覚情報と言語モデルを組み合わせ、高いレベルの計画を立て、実行する。
- 📈 Figure AIは、embodied AIを拡大するために最適な場所であり、現在積極的に人材を募集している。
Q & A
Figure AIとはどのような企業ですか?
-Figure AIはロボティクスに特化した企業であり、OpenAIと協力してAIとロボット技術を組み合わせています。
Figure AIとOpenAIのパートナーシップの目的は何ですか?
-このパートナーシップの目的は、OpenAIの高度な視覚と言語知能とFigure AIの迅速で緻密なロボット動作を組み合わせることです。
Figure AIのロボットはどのように人間と対話できますか?
-Figure AIのロボットは視覚的な情報を理解し、音声を通じて会話を行い、質問に応答することができます。
Figure AIのロボットはどのようにしてタスクを実行しますか?
-ロボットは環境を視覚的に認識し、その情報を基にして行動を決定し、物体を操作することでタスクを実行します。
Google DeepMindのRT2ロボットとFigure AIのロボットの違いは何ですか?
-Google DeepMindのRT2ロボットは独自の「ビジョン言語アクションモデル」を使用し、Figure AIのロボットはOpenAIの技術と統合されています。
ロボットが動作を学習するプロセスについてどのような情報がありますか?
-ロボットはウェブやロボティクスのデータから学習し、視覚的情報と動作を組み合わせて状況に応じた行動を実行します。
Figure AIのロボットはどのようにしてマルチタスクを処理しますか?
-ロボットは複数のタスクを同時に処理する能力を持ち、会話をしながら物理的なタスクを実行できます。
Figure AIとOpenAIの技術統合の特徴は何ですか?
-技術統合により、ロボットは視覚的情報と言語を理解し、複雑なタスクを独立して実行できるようになります。
ロボットによるテレオペレーションとはどういう意味ですか?
-テレオペレーションは、人間が遠隔でロボットを操作し、その動作を制御するプロセスを指します。
Figure AIのロボットの将来性についての見解は?
-Figure AIのロボットは、AIとロボティクスの進歩を代表し、将来的にはさらに多様なタスクを実行可能になると考えられます。
Outlines
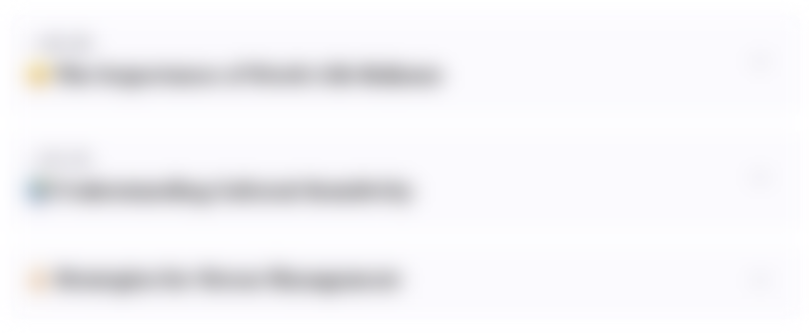
このセクションは有料ユーザー限定です。 アクセスするには、アップグレードをお願いします。
今すぐアップグレードMindmap
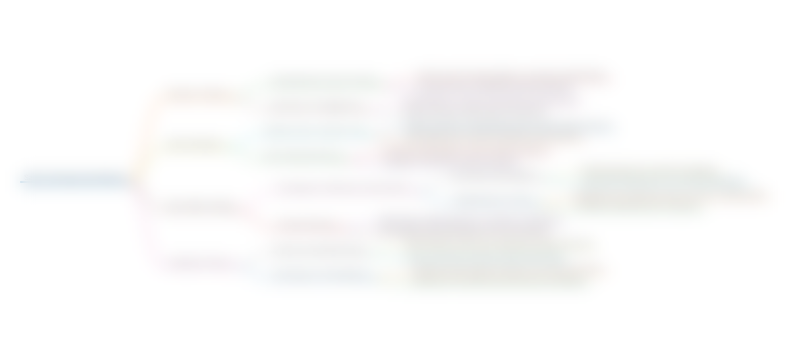
このセクションは有料ユーザー限定です。 アクセスするには、アップグレードをお願いします。
今すぐアップグレードKeywords
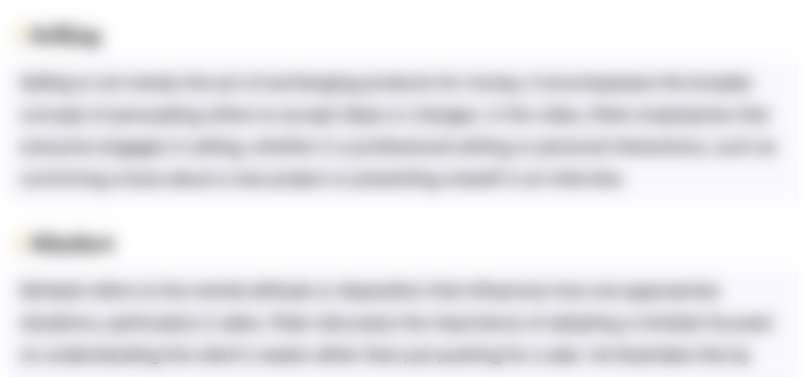
このセクションは有料ユーザー限定です。 アクセスするには、アップグレードをお願いします。
今すぐアップグレードHighlights
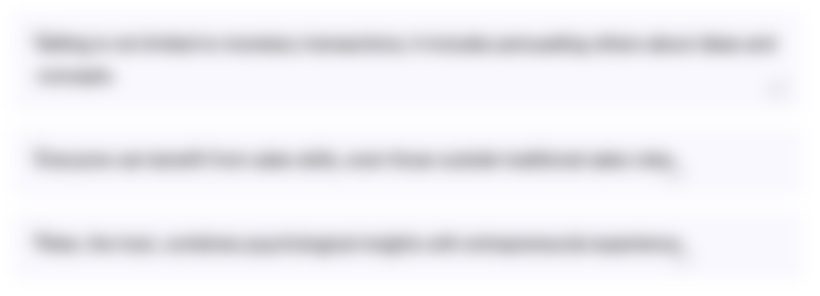
このセクションは有料ユーザー限定です。 アクセスするには、アップグレードをお願いします。
今すぐアップグレードTranscripts
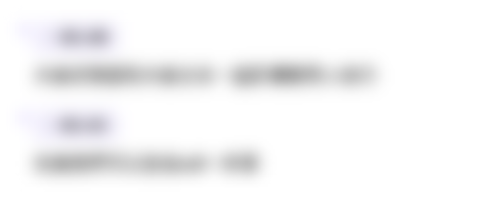
このセクションは有料ユーザー限定です。 アクセスするには、アップグレードをお願いします。
今すぐアップグレード5.0 / 5 (0 votes)