A Practical Introduction to Large Language Models (LLMs)
Summary
TLDRIn this data science series, Shah introduces large language models (LLMs), emphasizing their vast parameters and emergent properties like zero-shot learning. He explains the shift from supervised to self-supervised learning, highlighting next word prediction as the core task. Shah outlines three practical levels of LLM use: prompt engineering, model fine-tuning, and building your own LLM. The series aims to make LLMs accessible, with future videos covering APIs, open-source solutions, and practical applications.
Takeaways
- 😀 Shah introduces a new data science series focused on large language models (LLMs) and their practical applications.
- 🔍 The series will cover beginner-friendly introductions to LLMs, practical aspects, APIs, open-source solutions, fine-tuning, and building LLMs from scratch.
- 🗣 Large language models, like Chat GPT, are advanced chatbots that can generate human-like responses to queries.
- 📏 'Large' in LLM refers to the vast number of model parameters, ranging from tens to hundreds of billions, which define the model's functionality.
- 🌟 A key qualitative feature of LLMs is 'emergent properties', such as zero-shot learning, which allows models to perform tasks without explicit training for those tasks.
- 🔄 The shift from supervised learning to self-supervised learning in LLMs has been significant, with self-supervised learning relying on the structure within the data itself.
- 🔮 The core task of LLMs is next word prediction, which they learn through exposure to massive amounts of text data, allowing them to understand word associations and context.
- 🛠 Three levels of working with LLMs are discussed: prompt engineering (using LLMs out of the box), model fine-tuning (adjusting model parameters for specific tasks), and building your own LLM.
- 💻 Prompt engineering can be done through user interfaces like Chat GPT or programmatically via APIs and libraries like OpenAI or Hugging Face Transformers.
- 🔧 Model fine-tuning involves taking a pre-trained LLM and updating its parameters using task-specific examples, often resulting in better performance for specific use cases.
- 🏗 For organizations with specific needs, building a custom LLM may be necessary, involving data collection, pre-processing, model training, and deployment.
Q & A
What is the main focus of Shah's new data science series?
-The main focus of Shah's new data science series is to discuss large language models (LLMs) and their practical applications.
What is the difference between a large language model and a smaller one?
-Large language models differ from smaller ones in two main aspects: quantitatively, they have many more model parameters, often tens to hundreds of billions; qualitatively, they exhibit emergent properties like zero-shot learning that smaller models do not.
What is zero-shot learning in the context of large language models?
-Zero-shot learning refers to the capability of a machine learning model to complete a task it was not explicitly trained to do, showcasing an emergent property of large language models.
How does self-supervised learning differ from supervised learning in the context of large language models?
-In self-supervised learning, models are trained on a large corpus of data without manual labeling, using the inherent structure of the data to define labels. This contrasts with supervised learning, which requires manually labeled examples for training.
What is the core task that large language models are trained to do?
-The core task that large language models are trained to do is next word prediction, where they predict the probability distribution of the next word given the previous words.
What are the three levels of working with large language models mentioned by Shah?
-The three levels of working with large language models mentioned by Shah are: 1) Prompt Engineering, 2) Model Fine-tuning, and 3) Building your own Large Language Model.
What is meant by prompt engineering in the context of large language models?
-Prompt engineering refers to using a large language model out of the box, without altering any model parameters, and crafting prompts to elicit desired responses.
How does model fine-tuning in large language models work?
-Model fine-tuning involves adjusting at least one internal model parameter of a pre-trained large language model to optimize its performance for a specific task using task-specific examples.
Why might an organization choose to build its own large language model?
-An organization might choose to build its own large language model for security reasons, to customize training data, or to have full ownership and control over the model for commercial use.
What resources does Shah recommend for further exploration of large language models?
-Shah recommends the blog in Towards Data Science and a GitHub repository for more details, example code, and further exploration of large language models.
Outlines
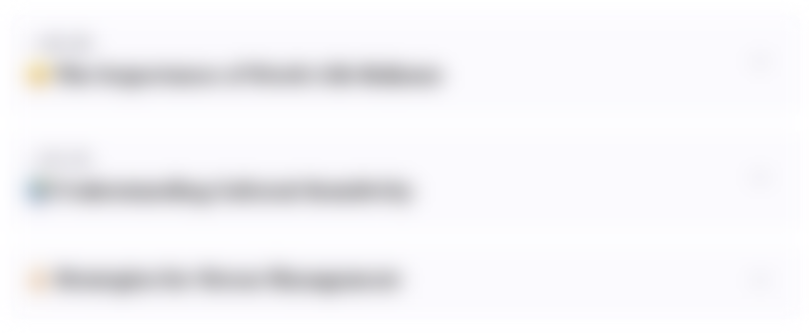
このセクションは有料ユーザー限定です。 アクセスするには、アップグレードをお願いします。
今すぐアップグレードMindmap
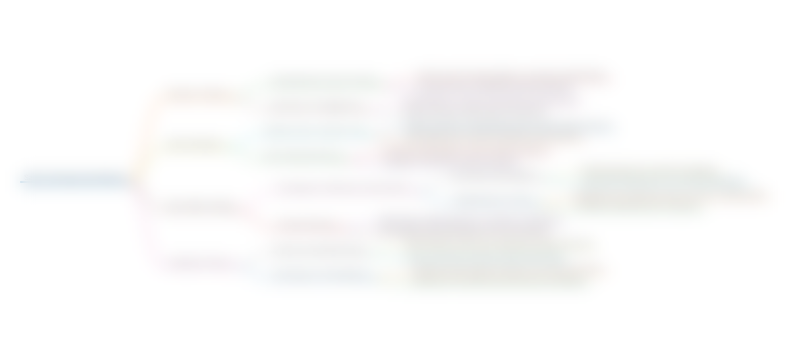
このセクションは有料ユーザー限定です。 アクセスするには、アップグレードをお願いします。
今すぐアップグレードKeywords
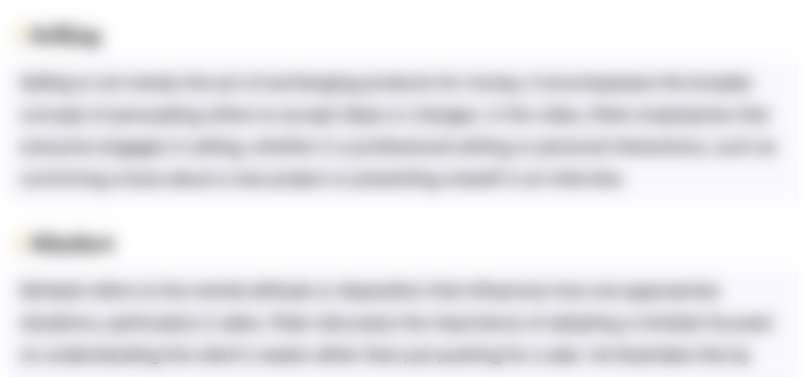
このセクションは有料ユーザー限定です。 アクセスするには、アップグレードをお願いします。
今すぐアップグレードHighlights
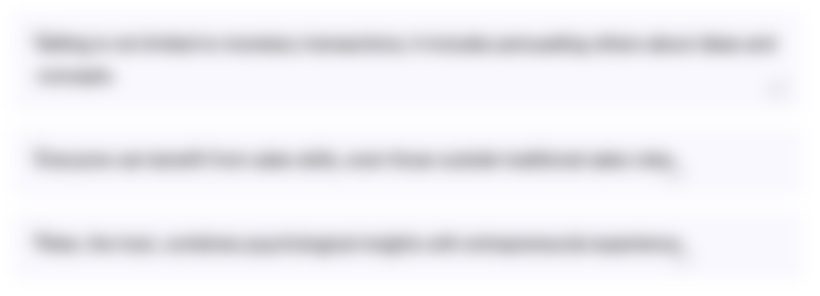
このセクションは有料ユーザー限定です。 アクセスするには、アップグレードをお願いします。
今すぐアップグレードTranscripts
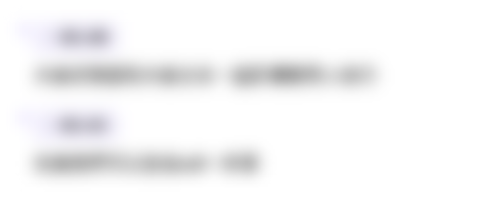
このセクションは有料ユーザー限定です。 アクセスするには、アップグレードをお願いします。
今すぐアップグレード5.0 / 5 (0 votes)