Introdução - Cadeias de Markov (Markov Chains) - Outspoken Market
Summary
TLDRThe video script introduces the concept of Markov Chains, a stochastic process where the next state depends only on the current state. The presenter, Leandro Guerra, aims to demystify the topic by explaining it with the help of a simple weather prediction example. He discusses the concept of states, transitions, and probabilities, and demonstrates how to calculate the likelihood of future states based on current conditions. The example uses two states (sun and rain) and their transitions to illustrate the calculations involved in predicting weather. The presenter emphasizes the practical application of Markov Chains and encourages the audience to explore the topic further.
Takeaways
- 📚 The concept of Markov Chains is introduced as a beautiful topic in the field of probability and stochastic processes.
- 🎓 Named after Russian mathematician Andrey Markov, these chains are characterized by the dependence of future states solely on the current state, not on the history of previous states.
- 🔢 Markov Chains are a type of stochastic process, meaning they involve a sequence of events where the probability of each event occurring is calculated based on the current state.
- 🌩️ An example used in the script is weather prediction, where two states (sun and rain) are used to demonstrate how probabilities can be calculated for future weather conditions.
- 🔄 The script emphasizes the importance of understanding the concept of 'state' in Markov Chains, including terms like 'previous state', 'current state', and 'next state'.
- 🚀 The concept of 'transition' is introduced as the probability of moving from one state to another, which is a fundamental aspect of Markov Chains.
- 🧠 The script aims to demystify Markov Chains, encouraging learners not to be intimidated by the complexity but to grasp the basic intuition behind the concept.
- 📈 The use of matrices and matrix multiplication is explained as a method for calculating probabilities across multiple transitions in a Markov Chain.
- 🔍 The script provides a practical example of calculating the probability of rain on Monday, given that it is raining on Saturday, using the transition matrix.
- 📚 The importance of algebra and matrix calculus is highlighted for handling complex Markov Chains with many states and transitions.
- 📈 The script concludes by encouraging further study and application of Markov Chains, emphasizing their utility in various fields and problems.
Q & A
What is the main topic of the video?
-The main topic of the video is an introduction to Markov Chains, explaining their concept, applications, and how they work in a simple and approachable way.
Who is the mathematician that Markov Chains are named after?
-Markov Chains are named after the Russian mathematician Andrey Andreyevich Markov.
What is the defining characteristic of a Markov Chain?
-The defining characteristic of a Markov Chain is that the next state of the system depends only on the current state and not on the previous states.
What does the term 'stochastic process' refer to?
-A stochastic process refers to a process that involves random variables and describes a sequence of events where the outcomes are determined by probability distributions.
How does the concept of probability relate to Markov Chains?
-In Markov Chains, the concept of probability is used to quantify the likelihood of transitioning from one state to another, based on the current state of the system.
What is an example of two states in the context of the video?
-In the video, the example given is weather prediction where state A represents a sunny day and state B represents a rainy day.
How does the video demonstrate the transition probabilities between states?
-The video demonstrates transition probabilities by showing how likely it is to move from one weather state to another, using percentages and a simple matrix to illustrate the transitions.
What is the purpose of the transition matrix in a Markov Chain?
-The transition matrix in a Markov Chain is used to calculate the probabilities of moving from one state to another over a certain number of transitions or steps in the chain.
How does the video simplify the calculation of Markov Chains?
-The video simplifies the calculation of Markov Chains by using a small number of states and explaining the process with a step-by-step example, making it easier to understand the underlying concepts.
What is the relevance of linear algebra in calculating Markov Chains?
-Linear algebra is relevant in calculating Markov Chains because it allows for the efficient manipulation and multiplication of transition matrices, which simplifies the process of determining probabilities over multiple steps.
What is the practical application of Markov Chains demonstrated in the video?
-The practical application demonstrated in the video is weather prediction, where Markov Chains can be used to estimate the probability of different weather conditions based on current conditions and historical data.
Outlines
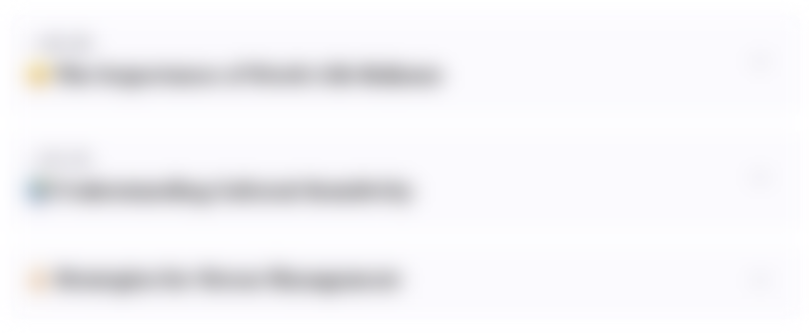
هذا القسم متوفر فقط للمشتركين. يرجى الترقية للوصول إلى هذه الميزة.
قم بالترقية الآنMindmap
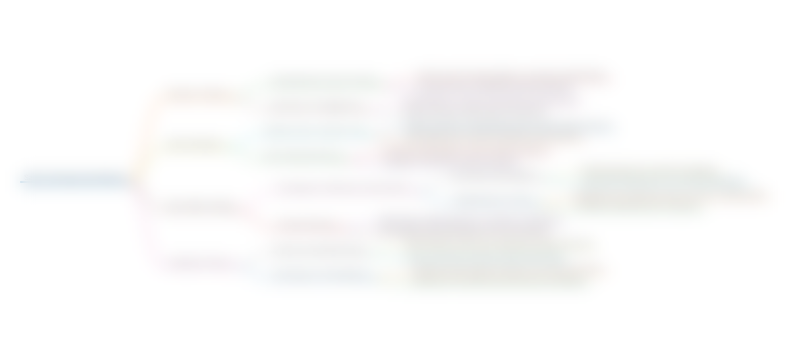
هذا القسم متوفر فقط للمشتركين. يرجى الترقية للوصول إلى هذه الميزة.
قم بالترقية الآنKeywords
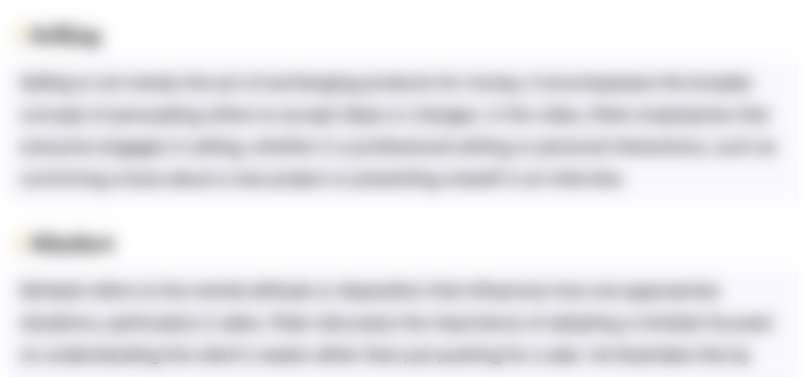
هذا القسم متوفر فقط للمشتركين. يرجى الترقية للوصول إلى هذه الميزة.
قم بالترقية الآنHighlights
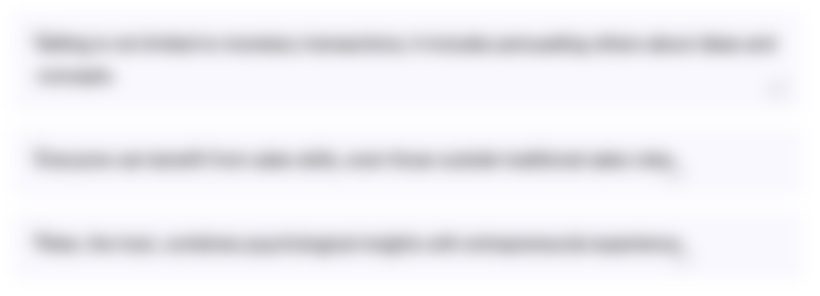
هذا القسم متوفر فقط للمشتركين. يرجى الترقية للوصول إلى هذه الميزة.
قم بالترقية الآنTranscripts
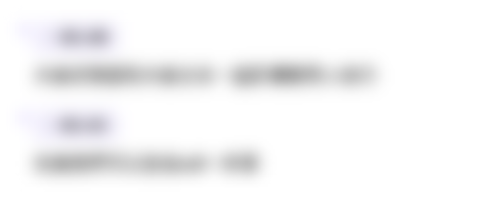
هذا القسم متوفر فقط للمشتركين. يرجى الترقية للوصول إلى هذه الميزة.
قم بالترقية الآن5.0 / 5 (0 votes)