SEM Series (2016) 4. Confirmatory Factor Analysis Part 1
Summary
TLDRThe video script offers a detailed tutorial on conducting a confirmatory factor analysis using Amos software. It guides viewers through the process of manually constructing a model, utilizing a plugin for efficiency, and importing data. The presenter demonstrates how to address common issues like missing data and error handling. Further, it covers model fit assessment, modification indices, and invariance testing by gender, providing practical insights into ensuring the reliability and validity of the analysis.
Takeaways
- 📕 The video script provides a step-by-step guide on conducting a confirmatory factor analysis (CFA) using the Amos program.
- 🔧 The script outlines both an easy method using a plugin and a more challenging manual approach to inputting a pattern matrix into Amos.
- 🔄 The manual method involves creating a model, adding latent factors, rotating them, and setting covariances manually within the Amos interface.
- 🔨 The video emphasizes the importance of correctly labeling variables and handling missing data to ensure the accuracy of the analysis.
- 🔇 It details the process of using plugins to simplify tasks in Amos, such as the pattern matrix model builder, and explains how to install and use these plugins.
- 🔬 The speaker discusses the process of checking model fit and validity, including looking at standardized estimates and modification indices.
- 🔅 The video highlights the significance of convergent and discriminant validity, explaining how to interpret the values and what thresholds to aim for.
- 🔍 The speaker provides insight into dealing with errors that arise during model fitting, such as missing observations and how to address them.
- 🔄 The script touches on multi-group analysis, explaining how to set up groups and conduct configural and metric invariance tests.
- 🔨 The video concludes with how to report the findings from the CFA, emphasizing the importance of providing evidence of model fit and invariance.
- 🔧 The script serves as an educational resource for users unfamiliar with Amos or those looking to refine their CFA techniques.
Q & A
What is the first step in conducting a confirmatory factor analysis according to the transcript?
-The first step is to put the pattern matrix into the Amos program.
What are the two ways to input the pattern matrix into Amos as described in the transcript?
-The two ways are using a plugin or building it by hand.
How does the plugin help in putting the pattern matrix into Amos?
-The plugin automates the process of creating the model by allowing users to paste the matrix directly into Amos.
What does the user need to do if the plugin does not work?
-If the plugin does not work, the user needs to build the model by hand, which involves opening Amos, creating a new model, and manually dragging and rotating the latent factors and observed variables.
How does the user ensure that all variables are covaried in the model?
-The user ensures all variables are covaried by manually selecting each one and applying the covariance, or by using the plugin's feature to automatically covariety them.
What does the user need to do to fix the labels in Amos?
-The user needs to go to view, interface properties, and then on the misc tab select 'don't display variable labels' to fix the labels.
Why is it important to name the latent factor variables without spaces according to the transcript?
-It is important because Amos automatically names them as 'f1' and 'f2' if not named properly, and renaming them afterward can be confusing.
What is the process of installing a plugin in Amos as described in the transcript?
-The process involves downloading the plugin, unblocking it if necessary, and then placing it into the Amos plugins folder.
What is the purpose of the Excel Stats Tools Package mentioned in the transcript?
-The Excel Stats Tools Package is used to help with the analysis and management of data in Excel, which can then be imported into Amos.
What does the user need to check for in terms of model fit when conducting the confirmatory factor analysis?
-The user needs to check the ACFI, RMSEA, and PCLOSE values to ensure the model has good fit.
What is the significance of the chi-square value in the context of the model fit?
-The chi-square value indicates how well the model fits the data. A large chi-square value can suggest a poor fit, but it can also be inflated by a complex model or large sample size.
Outlines
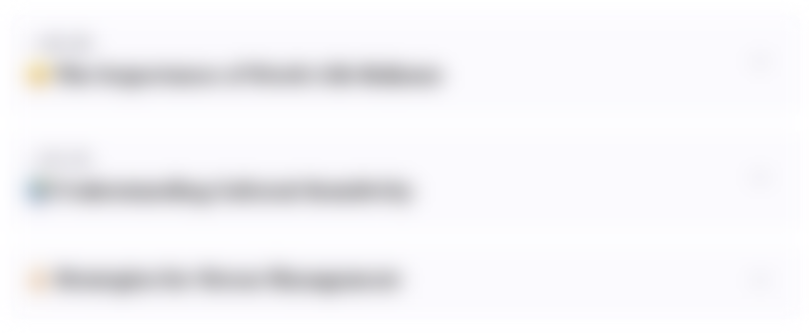
هذا القسم متوفر فقط للمشتركين. يرجى الترقية للوصول إلى هذه الميزة.
قم بالترقية الآنMindmap
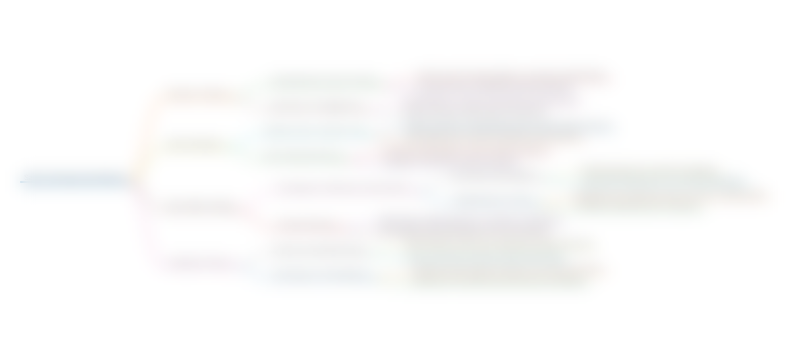
هذا القسم متوفر فقط للمشتركين. يرجى الترقية للوصول إلى هذه الميزة.
قم بالترقية الآنKeywords
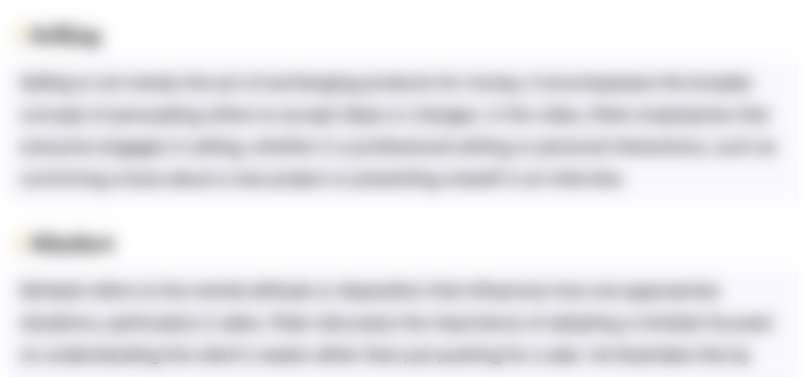
هذا القسم متوفر فقط للمشتركين. يرجى الترقية للوصول إلى هذه الميزة.
قم بالترقية الآنHighlights
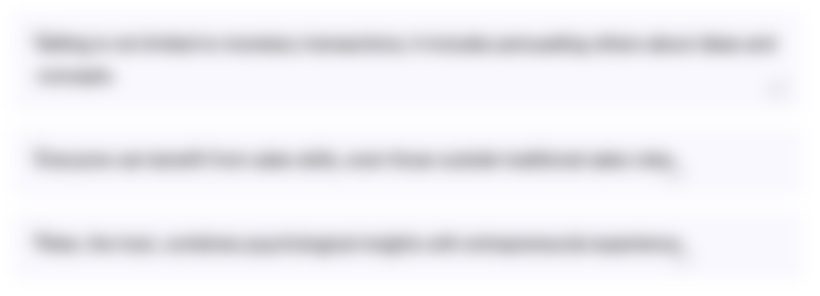
هذا القسم متوفر فقط للمشتركين. يرجى الترقية للوصول إلى هذه الميزة.
قم بالترقية الآنTranscripts
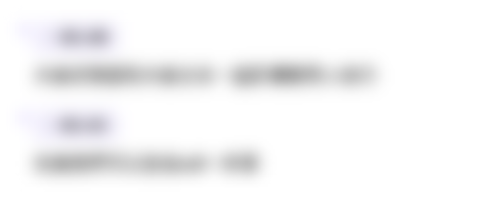
هذا القسم متوفر فقط للمشتركين. يرجى الترقية للوصول إلى هذه الميزة.
قم بالترقية الآن5.0 / 5 (0 votes)