Coming soon- robots that drive
Summary
TLDRThe script delves into the evolution of self-driving car technology, starting from the 1960s with the Stanford Cart and Shakey, which utilized vision systems to navigate. Fast forward to the DARPA Urban Challenge in 2007, where 'Boss' from Carnegie Mellon University excelled. By 2014, Google's autonomous vehicle demonstrated advanced sensors and point cloud imaging for a more sophisticated understanding of the environment. The narrative highlights the progression from rudimentary robotic navigation to the sophisticated, sensor-rich systems capable of making complex driving decisions.
Takeaways
- 🤖 The concept of self-driving cars has been a staple in science fiction for a long time, but their technological origins can be traced back to the 1960s.
- 👓 The Stanford Cart, developed by Hans Moravec, was an early attempt at creating a self-driving machine using stereo vision to navigate and avoid obstacles.
- 📈 The limited computing power of the 1960s greatly affected the speed and capabilities of early self-driving technology like the Stanford Cart.
- 🔍 Shakey, developed at SRI International, was another pioneering robot that used vision to map its environment, contributing to the evolution of autonomous vehicles.
- 🏁 The DARPA Urban Challenge in 2007 was a significant milestone, showcasing the progress of self-driving cars and their ability to perform tasks comparable to human drivers.
- 🏆 Carnegie Mellon University's 'Boss' won the DARPA Urban Challenge, highlighting the importance of advanced sensors and computing equipment in autonomous vehicles.
- 🚗 By 2014, Google's self-driving cars had become more streamlined, with the Velodyne scanning laser range finder being the most prominent sensor.
- 🌐 The point cloud image, generated by the Velodyne scanner, provides a 3D geometric model that allows the car's software to make driving decisions.
- 🛑 The software in self-driving cars processes rich sensory information to create a driving plan, including adjusting the steering, throttle, and brakes.
- 🚦 Modern self-driving cars can identify and respond to various objects and road signs, demonstrating an advanced level of environmental awareness and decision-making.
- 📊 The evolution of self-driving car technology from the 1960s to the present shows rapid advancements in sensors, computing power, and software capabilities.
Q & A
What is the significance of the Stanford cart in the history of self-driving car technology?
-The Stanford cart is significant because it was one of the earliest research robots to use a stereo vision system to perceive its environment and plan a path to avoid obstacles, marking an early step towards self-driving car technology.
What was the primary limitation of the Stanford cart?
-The primary limitation of the Stanford cart was its excruciatingly slow speed, which was mainly due to the limited computing power available in 1964.
What was Shakey, and how did it contribute to the development of self-driving cars?
-Shakey was a famous robot developed at SRI International in the late 60s and 70s. It used vision to build a map of its environment and navigate, contributing to the advancement of self-driving car technology.
What was the DARPA Urban Challenge and why was it important?
-The DARPA Urban Challenge was a competition in 2007 where teams built robot cars to perform tasks like human drivers, such as parking and intersection management. It was important because it significantly advanced the technology and capabilities of self-driving cars.
Which team won the DARPA Urban Challenge and what was their robot car called?
-The team from Carnegie Mellon University won the DARPA Urban Challenge, and their robot car was called 'Boss'.
How did the appearance of self-driving cars change from the DARPA Urban Challenge to 2014?
-By 2014, self-driving cars like Google's became much sleeker, with only one obvious sensor, a Velodyne scanning laser range finder, making them look more like ordinary cars.
What is a point cloud image and how is it used in self-driving cars?
-A point cloud image is a three-dimensional representation of the world surrounding the car, created by sensors like the Velodyne scanner. It helps the car's software make decisions about navigation by identifying objects, humans, and road signs.
How do self-driving cars process the sensory information they collect?
-Self-driving cars process sensory information by creating a three-dimensional geometric model of the environment, which the onboard software uses to make decisions and send commands to control the vehicle.
What are the key components of a self-driving car's sensory system?
-Key components of a self-driving car's sensory system include cameras, LiDAR sensors like the Velodyne scanner, and other sensors that help in perceiving the environment and planning the vehicle's movements.
How do the colors in a point cloud image represent different features of the environment?
-In a point cloud image, cool colors like blue represent the ground plane, green indicates points above the ground plane, and red is used for points that are very high above the ground plane.
What role does high-performance computing play in self-driving cars?
-High-performance computing is crucial in self-driving cars as it processes and analyzes the vast amounts of sensory data in real-time, enabling the car to make quick decisions and navigate safely.
Outlines
🤖 Early Self-Driving Car Technology
The script begins by discussing the historical roots of self-driving car technology, dating back to the 1960s with two notable research robots. The Stanford cart, developed by Hans Moravec, utilized a stereo vision system to navigate its environment and avoid obstacles, albeit at a slow pace due to limited computing power in 1964. Shakey, developed at SRI International, also relied on visual input to map its surroundings, marking significant advancements in autonomous vehicle technology.
Mindmap
Keywords
💡Self-driving cars
💡Stereo vision
💡Computing power
💡Shakey
💡DARPA Urban Challenge
💡Boss
💡Google cars
💡Velodyne scanner
💡Point cloud
💡High-performance computing
💡Onboard software
Highlights
Interest in self-driving cars has deep roots in both real-world development and science fiction.
The Stanford Cart from the 1960s represents an early milestone in autonomous vehicle technology.
Hans Moravec's Stanford Cart utilized stereo vision to navigate and avoid obstacles.
Early self-driving technology was constrained by the limited computing power of the time.
Shakey, developed at SRI International, was another pioneering robot that used vision to map its environment.
The DARPA Urban Challenge in 2007 was a significant event that spurred advancements in autonomous vehicle technology.
Boss, developed by Carnegie Mellon University, won the DARPA Urban Challenge, showcasing advanced capabilities in autonomous driving.
Modern self-driving cars, like Google's, have evolved to have more streamlined and less obtrusive sensor systems.
The Velodyne scanner is a key sensor used in modern autonomous vehicles for creating a 3D model of the environment.
Point cloud technology allows self-driving cars to perceive their surroundings in three dimensions.
Color coding in point cloud images helps differentiate between the ground plane and objects above it.
Autonomous vehicle software processes sensory data to make driving decisions and control the vehicle.
Self-driving cars can detect and respond to various objects, including humans, other cars, and road signs.
Onboard software creates a driving plan and sends commands to adjust vehicle components like the steering wheel, throttle, and brake.
The evolution of self-driving car technology reflects significant improvements in sensor integration and computational efficiency.
The DARPA Urban Challenge was a pivotal event that accelerated the development of autonomous driving capabilities.
The Boss robot car's victory in the DARPA Challenge demonstrated the potential for autonomous vehicles to perform complex driving tasks.
Google's autonomous vehicles represent a leap forward in the practical application of self-driving technology.
Transcripts
There is a lot of interest today in robots that drive, otherwise known as self driving
cars and such technology has been depicted in fiction for a very long time. The origin
of self driving car technology can probably be traced back to these two research robots
from the 1960s.
On the left we have a machine known as the Stanford cart. This one here was built by
Hans Moravec. What this robot is doing is using a vision system. In fact, a stereo vision
system to reconstruct the three dimensional nature of the world in which it’s driving.
It uses that information to plan a path so that it can avoid hitting any of these obstacles.
This machine was excruciatingly slow. Mostly dictated by the limited computing power that
was available for the problem back in 1964.
The robot on the right is also pretty famous. It’s known as Shakey and developed at SRI
International in the late 60s and its career went on through the 70s. This robot also used
sense of vision to build a map of the environment in which it was navigating. A major step forward
in self driving car technology was the DARPA urban challenge in 2007. A number of teams competed
to build robot cars that could perform as well as human drivers. They had to perform
tasks like moving into parking bays. They had to do the right things at the intersections.
They had to demonstrate that they could do overtaking and all of this safely with skill
levels comparable to human drivers.
The winner of that competition was this robot car called, ‘Boss’ developed by Carnegie
Mellon University and we can see that it doesn't look anything like an ordinary car. It’s
bristling with all sorts of sensory devices and a large part of the car is filled with
high performance computing equipment.
Now technology has evolved pretty rapidly so by the year 2014, Google cars look much
more sleek. There is really in fact only one sensor that’s obvious when you look at the
car and that is the device known as a Velodyne scanning laser range finder on the roof of
the car. The way the robot car sees its world is shown here in what we call a point cloud
image and this is generated by that Velodyne scanner that we saw on top of the Google car.
The point cloud is a number of points in three dimensional space and they are typically color
coded. So the colors blue, the cool colors indicate the ground plane on which the robot
is driving and points above the ground plane where it might be imprudent to drive are colored
green or red for those points that are very high above the ground plane. So from this
fairly simple three dimensional geometric model of the world surrounding the car, the
software on board the car is able to make a number of decisions about which direction
it should drive.
It’s able to see other objects perhaps human beings, perhaps other cars, perhaps road signs.
The software on board the vehicle has to take all of this rich sensory information and create
a plan and then send commands to the car to adjust the steering wheel or to adjust the
throttle or to adjust the brake.
تصفح المزيد من مقاطع الفيديو ذات الصلة

Dieser KI Durchbruch wird Fahrzeugen das autonome Fahren beibringen
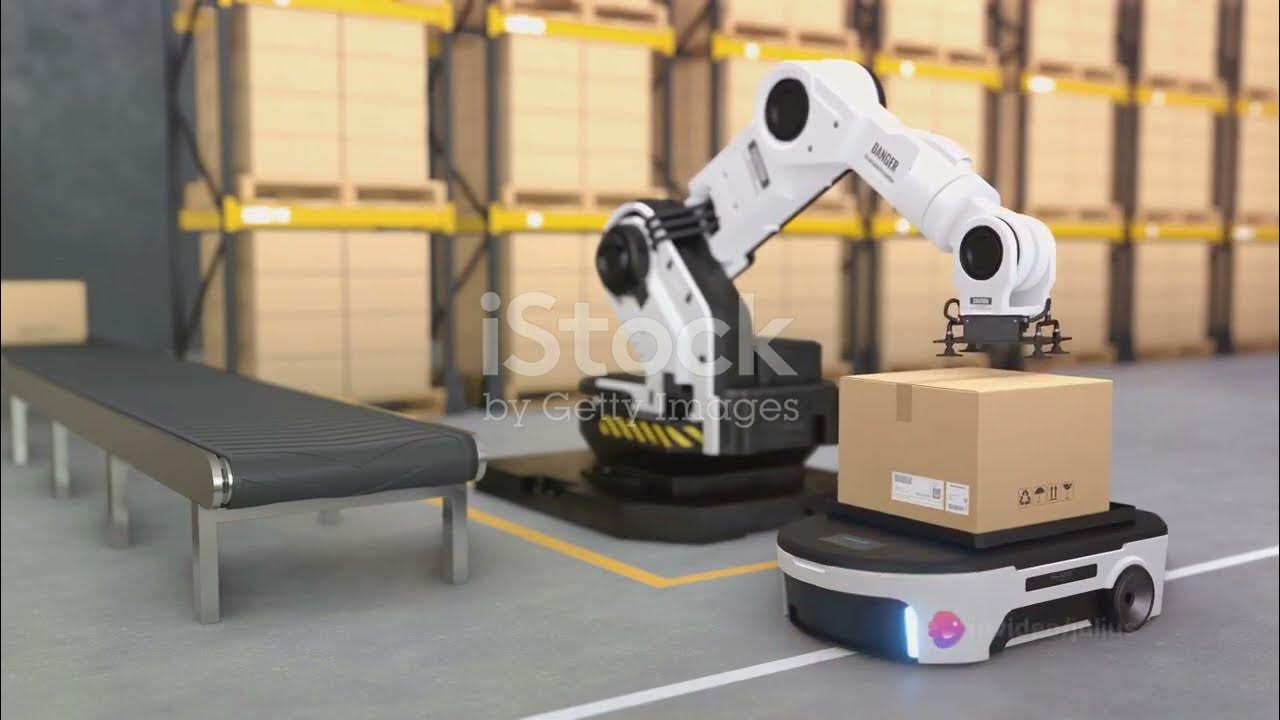
Robotics Sensors 1: The Eyes and Ears of Robots
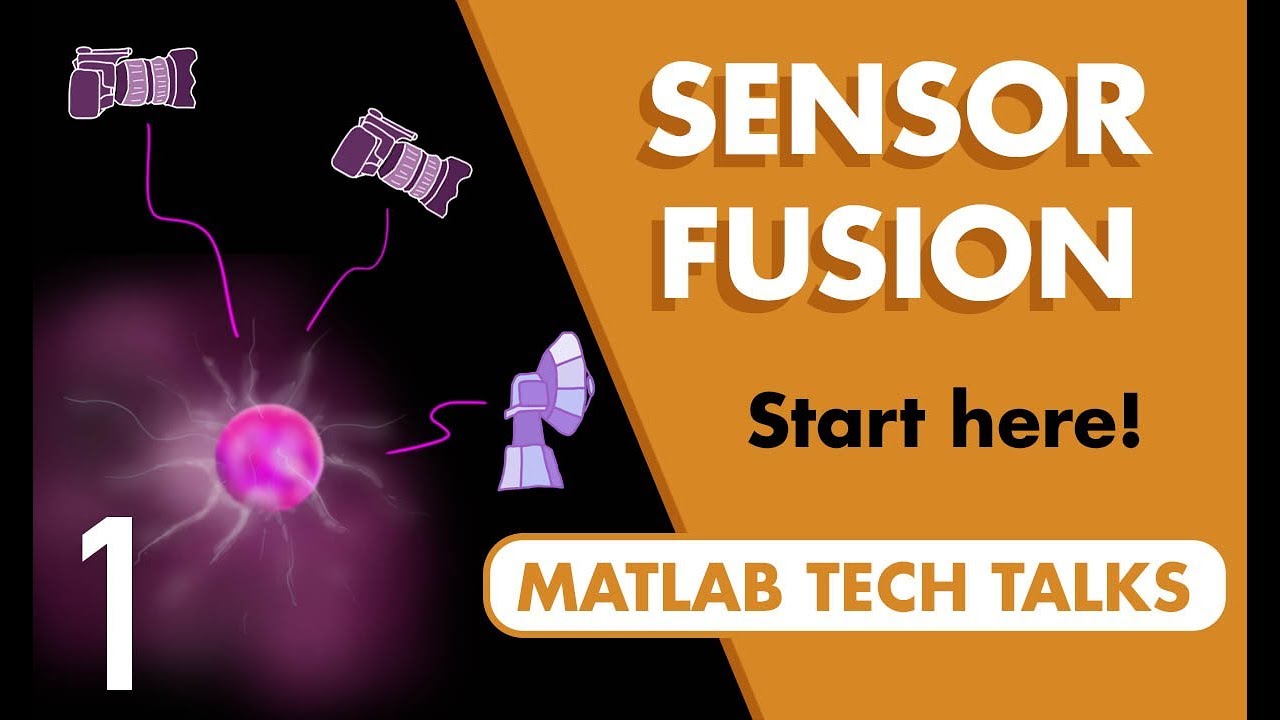
Understanding Sensor Fusion and Tracking, Part 1: What Is Sensor Fusion?
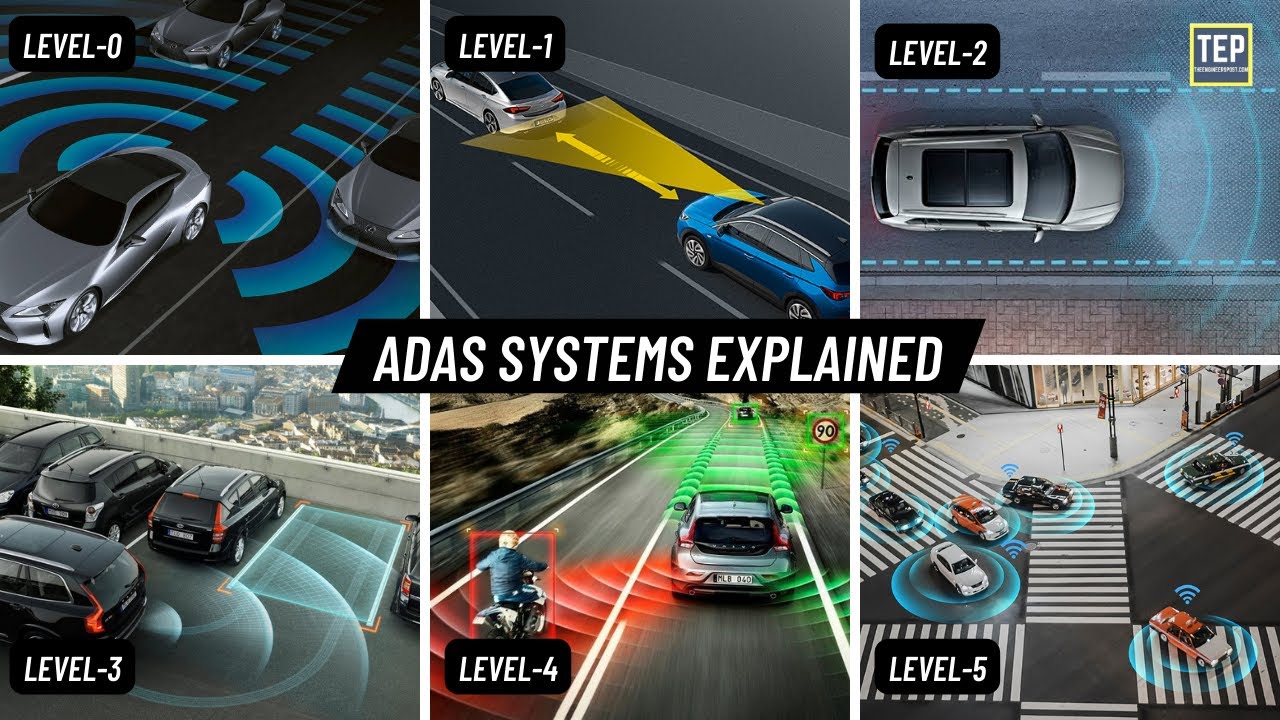
Advanced Driver Assistance System | Every ADAS Levels in Car Explained
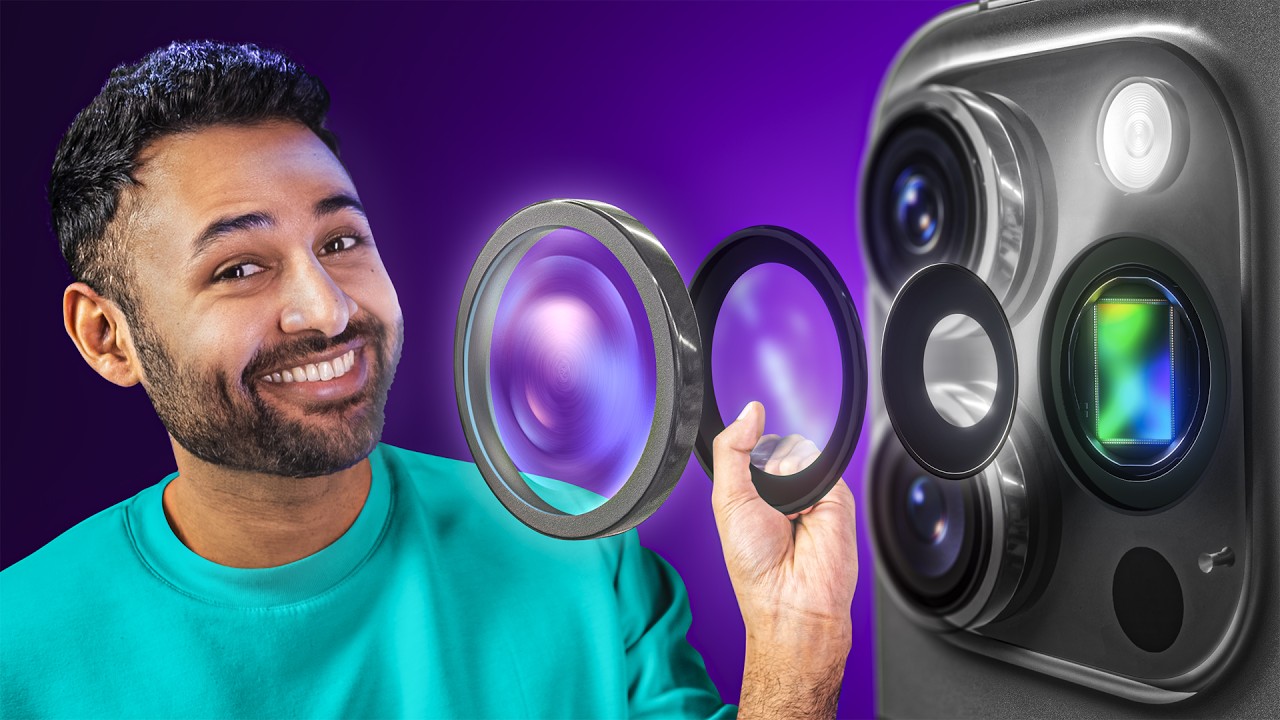
How smartphone cameras ACTUALLY work!
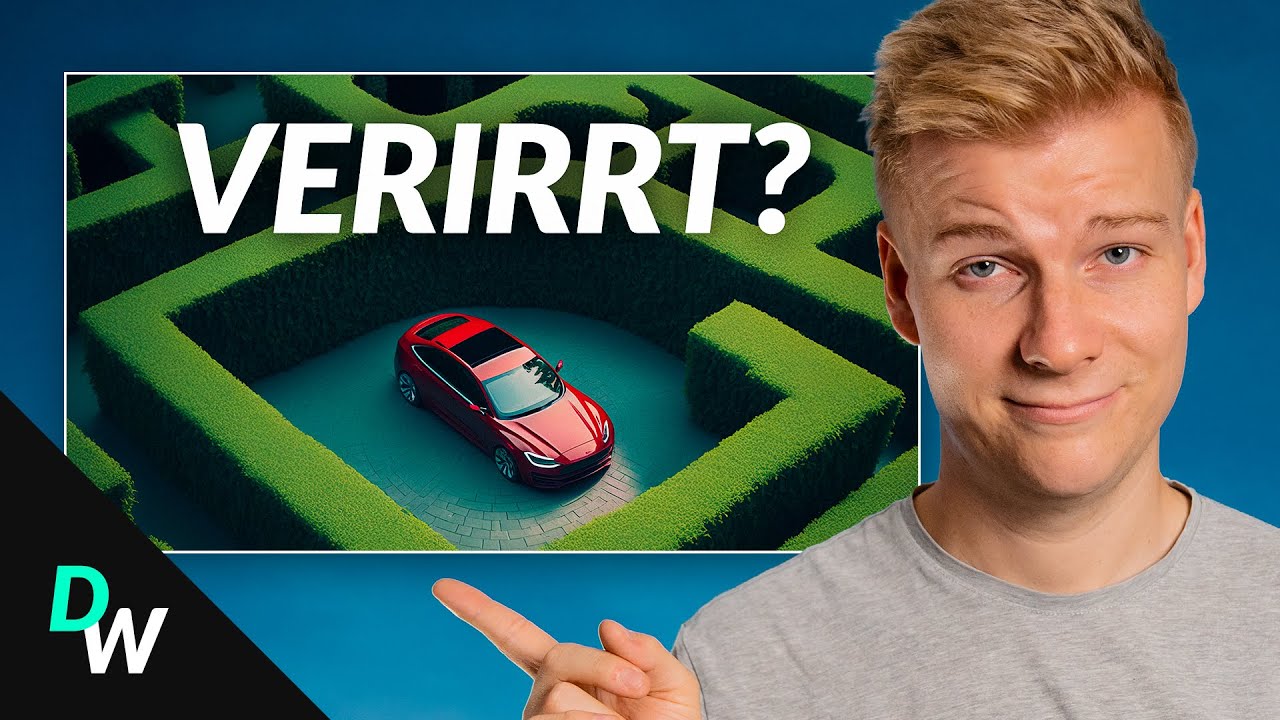
Warum es noch immer keine selbstfahrenden Autos gibt
5.0 / 5 (0 votes)