AI Won’t Plateau — if We Give It Time To Think | Noam Brown | TED
Summary
TLDRThe speaker discusses the incredible progress of AI in the past five years, focusing on the impact of scaling data, computation, and model size. Despite rising costs, AI's evolution continues through a new paradigm—scaling up 'system 2 thinking' (deliberate, slow decision-making) instead of just training larger models. By drawing on experiences from developing AI for poker, chess, and Go, the speaker shows that longer thinking times enhance AI performance. This shift has vast implications, enabling AI to tackle complex real-world problems and accelerate progress in crucial areas like medicine and scientific research, ultimately proving that AI’s potential is far from plateauing.
Takeaways
- 😀 AI's incredible progress over the past five years is primarily driven by scaling data and computational power, rather than major changes in architecture.
- 😀 While AI models today are much larger and cost hundreds of millions of dollars to train, the cost to query these models is still minimal—fractions of a penny per query.
- 😀 AI might face concerns about plateauing as models become increasingly expensive, but scaling up 'system two thinking' offers a new direction for continued progress.
- 😀 A personal story from the speaker's PhD journey shows how allowing AI to take more time to think (system two thinking) can significantly boost performance, much more than simply scaling up the model.
- 😀 The poker AI developed by the speaker demonstrated that allowing it to take just 20 seconds to think per hand provided the same performance boost as scaling the model by 100,000x.
- 😀 The shift from system one (fast, intuitive thinking) to system two (slower, more methodical thinking) was key to the AI's success, both in poker and other domains.
- 😀 Other famous AI achievements, like Deep Blue in chess and AlphaGo in Go, also benefited from taking time to think, showing this pattern applies across various games.
- 😀 In 2021, research showed that scaling thinking time by 10x in games could have the same effect as scaling model size and training by 10x.
- 😀 A new paradigm in AI development focuses not just on scaling model training, but also on scaling system two thinking, which has the potential to revolutionize AI's real-world applications.
- 😀 While AI models that take longer to think might cost more per query, this added cost could be worth it for solving critical, high-stakes problems like cancer treatments and renewable energy solutions.
Q & A
What is the main factor driving AI progress over the past 5 years?
-The main factor driving AI progress is scale, particularly the scale of data and compute used to train models. While the underlying architecture, like the Transformer, has remained largely the same, improvements have come from using larger datasets and more computational resources.
How much did it cost to train GPT-2 in 2019?
-It cost about $5,000 to train GPT-2 in 2019.
What is the concern about the future of AI progress?
-There is a concern that AI may soon plateau or hit a wall due to the massive costs of scaling models. Some worry that the financial and computational demands of training AI will eventually break the scaling paradigm.
What experience does the speaker reference to highlight AI's potential?
-The speaker refers to his experience as a PhD student working on developing AI systems to play poker. This project, which began in 2012, involved teaching AI to play poker and led to significant breakthroughs in AI's performance.
How does the performance of the AI bot in poker compare to human experts?
-In a competition against top human experts in 2015, the AI bot initially struggled, losing by a wide margin. However, the bot's ability to think quickly (within milliseconds) was contrasted with the human experts, who took time to think through their decisions.
What is the difference between system one and system two thinking, as discussed in the script?
-System one thinking is fast, intuitive, and automatic, used for quick decisions like recognizing a friendly face. System two thinking is slower, more deliberate, and requires more thought, used for complex tasks like planning or problem-solving.
How did adding 20 seconds of 'system two thinking' affect the AI bot's performance in poker?
-Adding just 20 seconds of system two thinking in poker resulted in the same performance improvement as scaling up the model by 100,000 times, showing the immense power of thoughtful deliberation in AI.
How did the AI bot's redesigned strategy in 2017 change its performance in poker?
-In 2017, the AI bot was redesigned to scale up both system one (quick thinking) and system two (deliberate thinking). This new approach led to a decisive victory over top poker professionals in a 120,000-hand competition, beating them by a huge margin.
What is the significance of AI taking time to think in games like poker, chess, and Go?
-In games like poker, chess, and Go, AI benefits significantly from taking time to think, as demonstrated by the success of Deep Blue in chess and AlphaGo in Go. These AIs performed much better when allowed time for deliberation, leading to victories over world champions.
What new approach to scaling AI models does the speaker suggest?
-The speaker suggests a new approach to scaling AI by not only increasing the size of models (system one) but also by scaling up system two thinking, allowing AI to take more time to deliberate before providing an answer. This untapped direction could lead to even more powerful AI models.
Why does the speaker believe that scaling system two thinking could be worth the additional cost?
-The speaker argues that while scaling system two thinking increases the cost of querying an AI, the potential benefits in solving important and complex problems—like cancer treatment or efficient solar panels—are worth the extra cost, making it a valuable investment.
What recent AI model does the speaker mention that uses system two thinking?
-The speaker mentions the launch of the O1 series of language models by OpenAI, which incorporates system two thinking by taking time to think before responding, improving the quality of answers.
Outlines
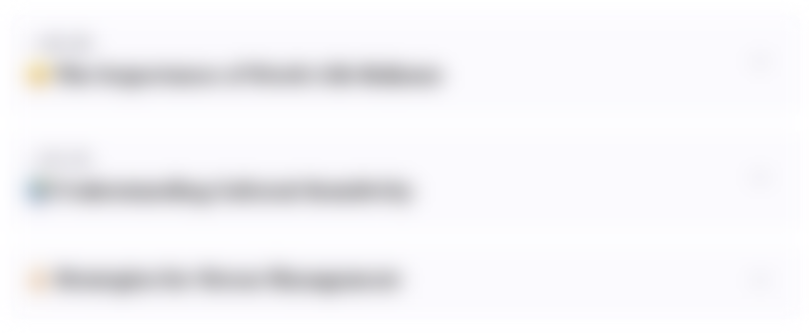
This section is available to paid users only. Please upgrade to access this part.
Upgrade NowMindmap
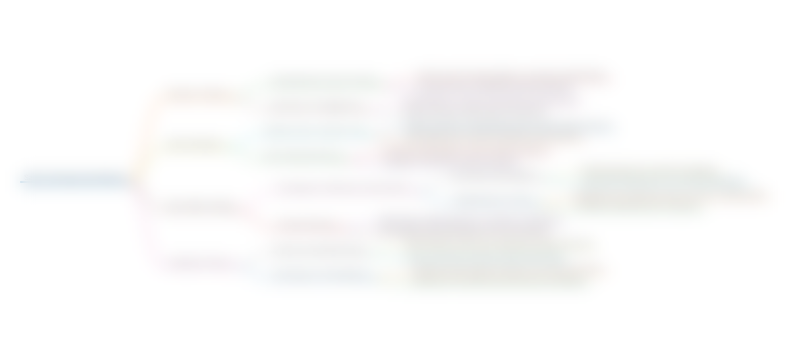
This section is available to paid users only. Please upgrade to access this part.
Upgrade NowKeywords
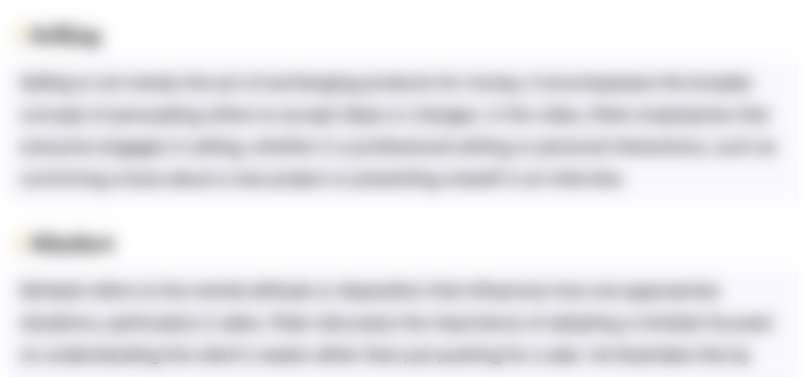
This section is available to paid users only. Please upgrade to access this part.
Upgrade NowHighlights
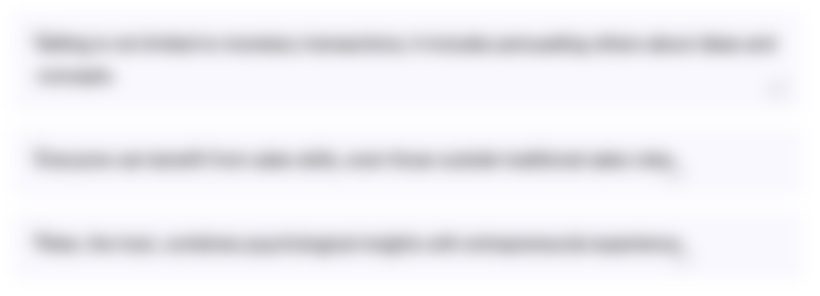
This section is available to paid users only. Please upgrade to access this part.
Upgrade NowTranscripts
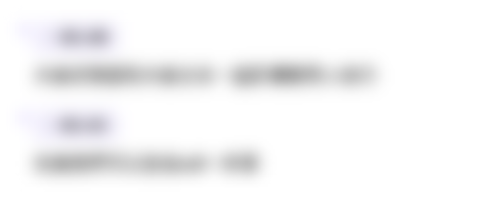
This section is available to paid users only. Please upgrade to access this part.
Upgrade NowBrowse More Related Video

AI can't cross this line and we don't know why.
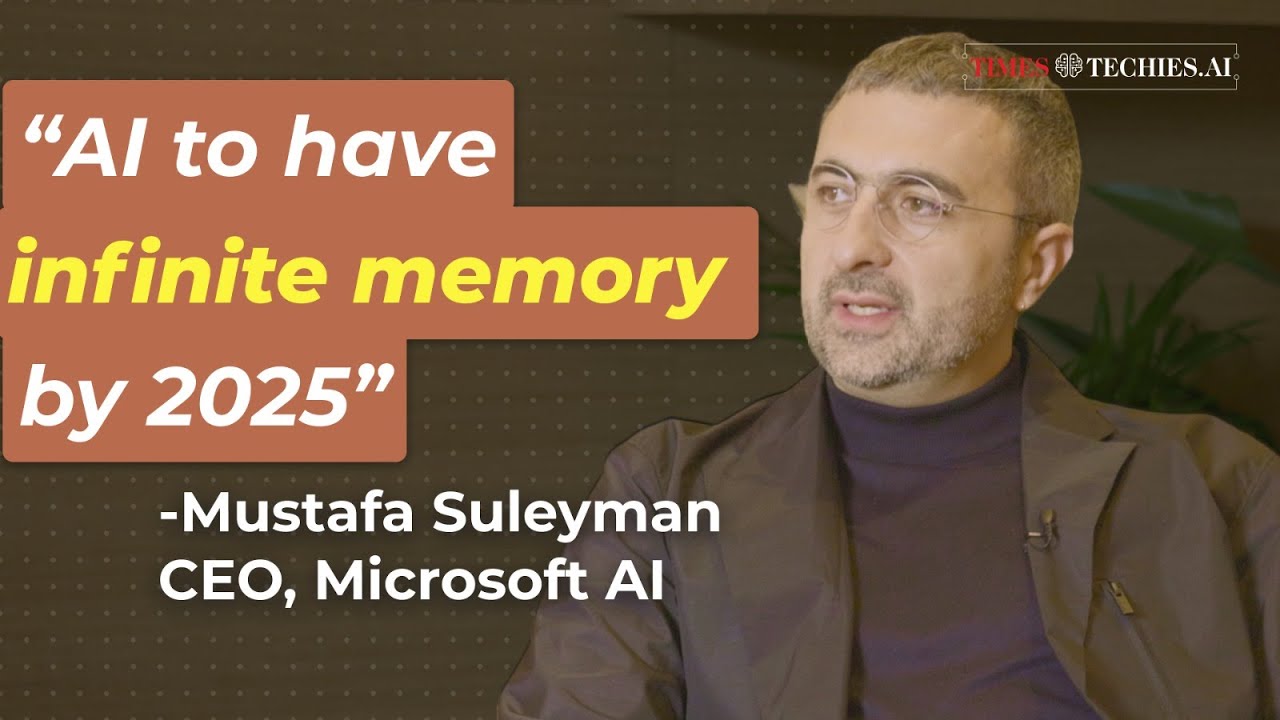
@Microsoft AI CEO Mustafa Suleyman x Times Techies | An exclusive interaction | #copilot
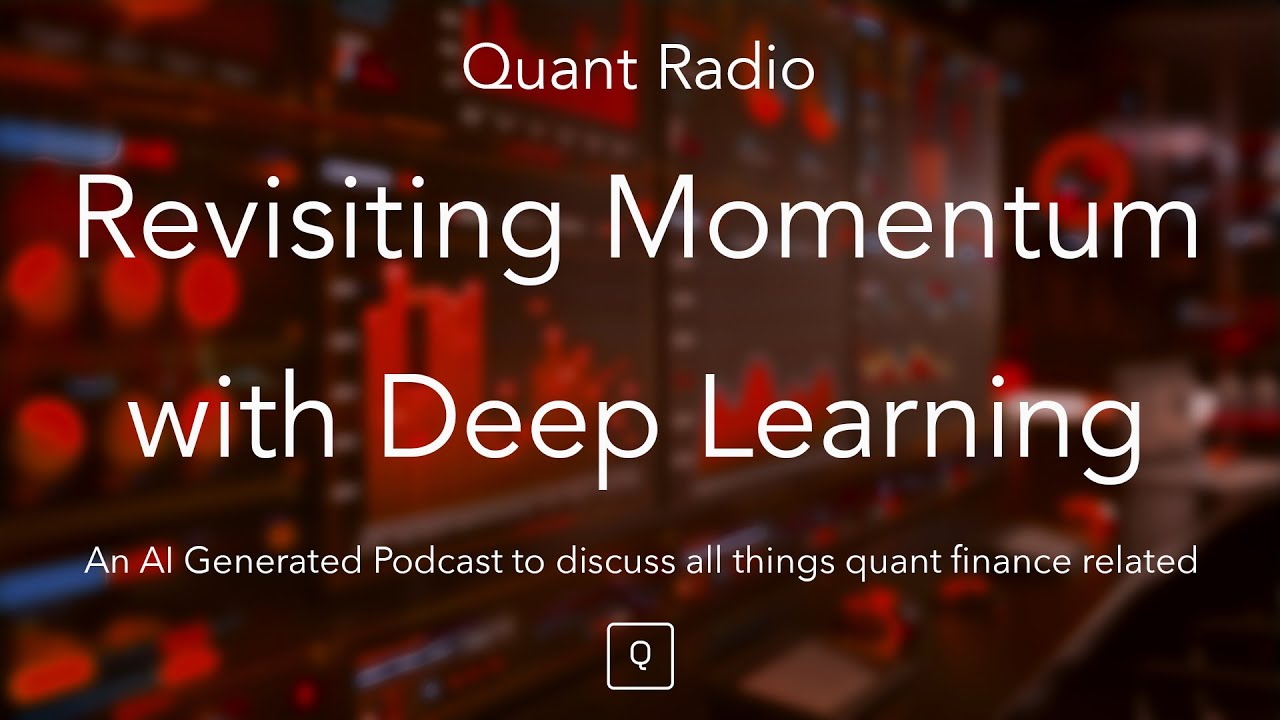
Quant Radio: Revisiting Momentum with Deep Learning
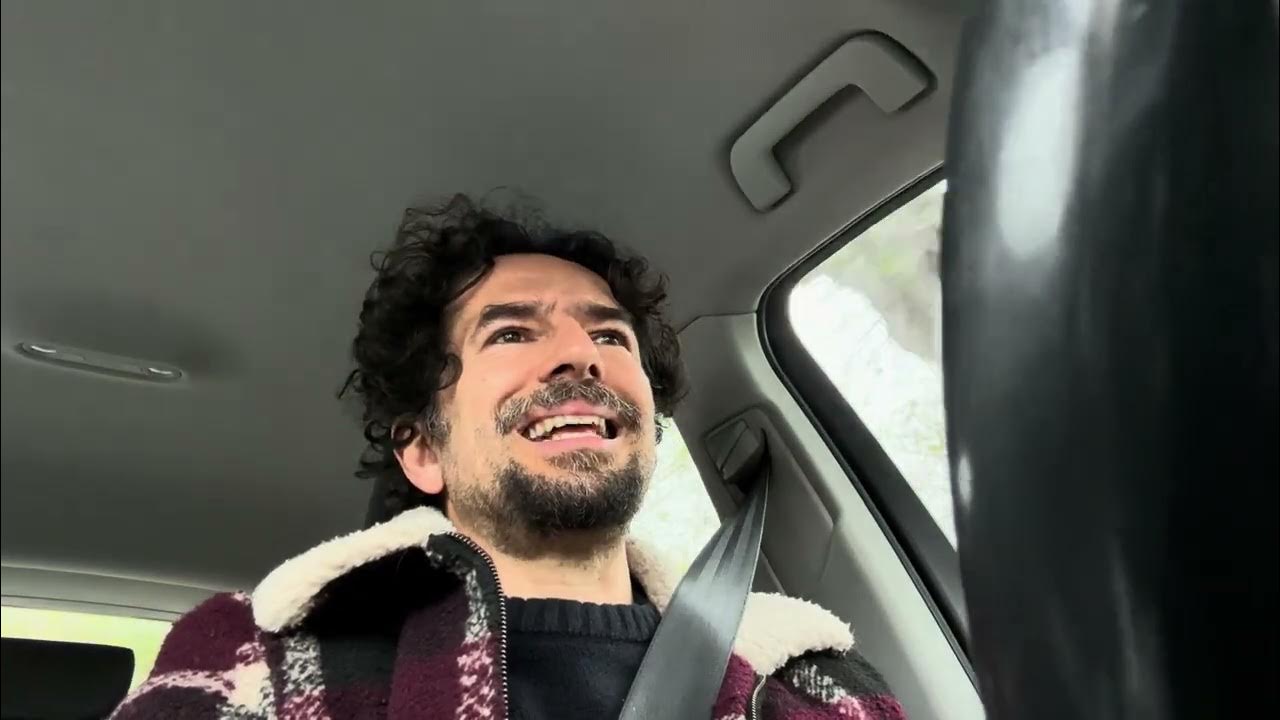
L'inesplicabile utilità di Claude Sonnet, a prescindere da ciò che dicono i benchmark
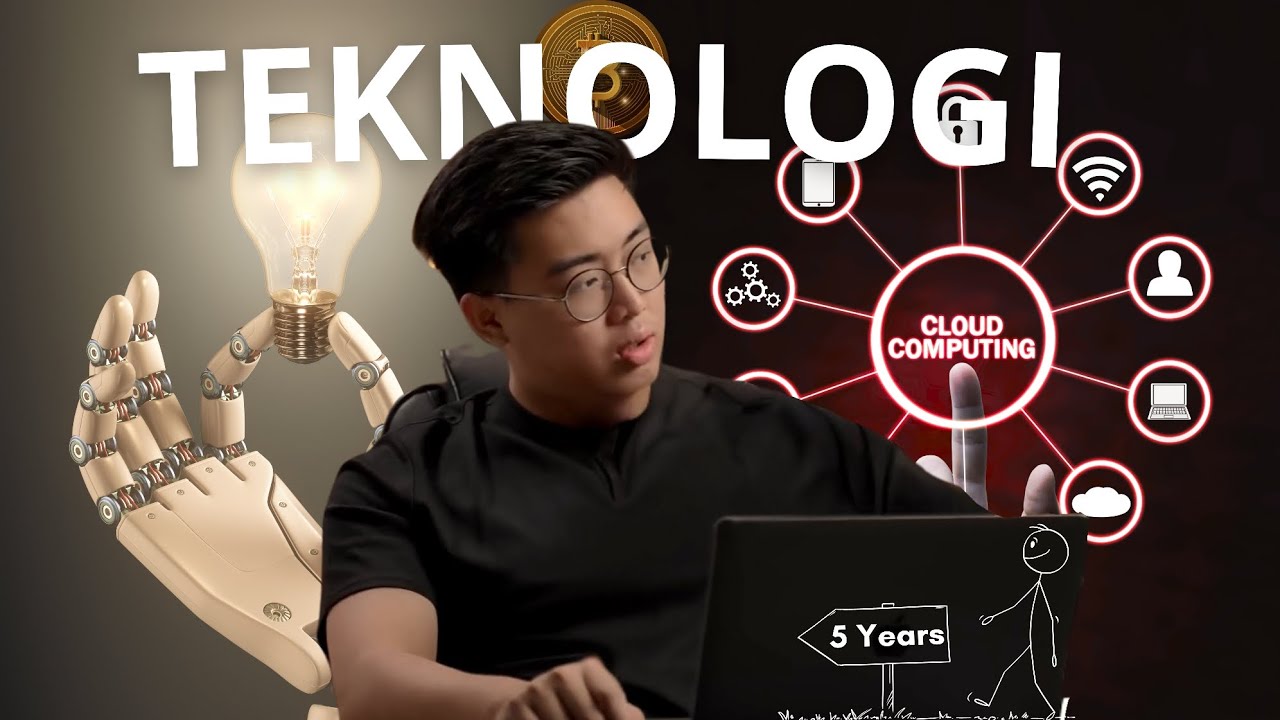
Mempersiapkan Diri 13 Menit - Tahun 2030
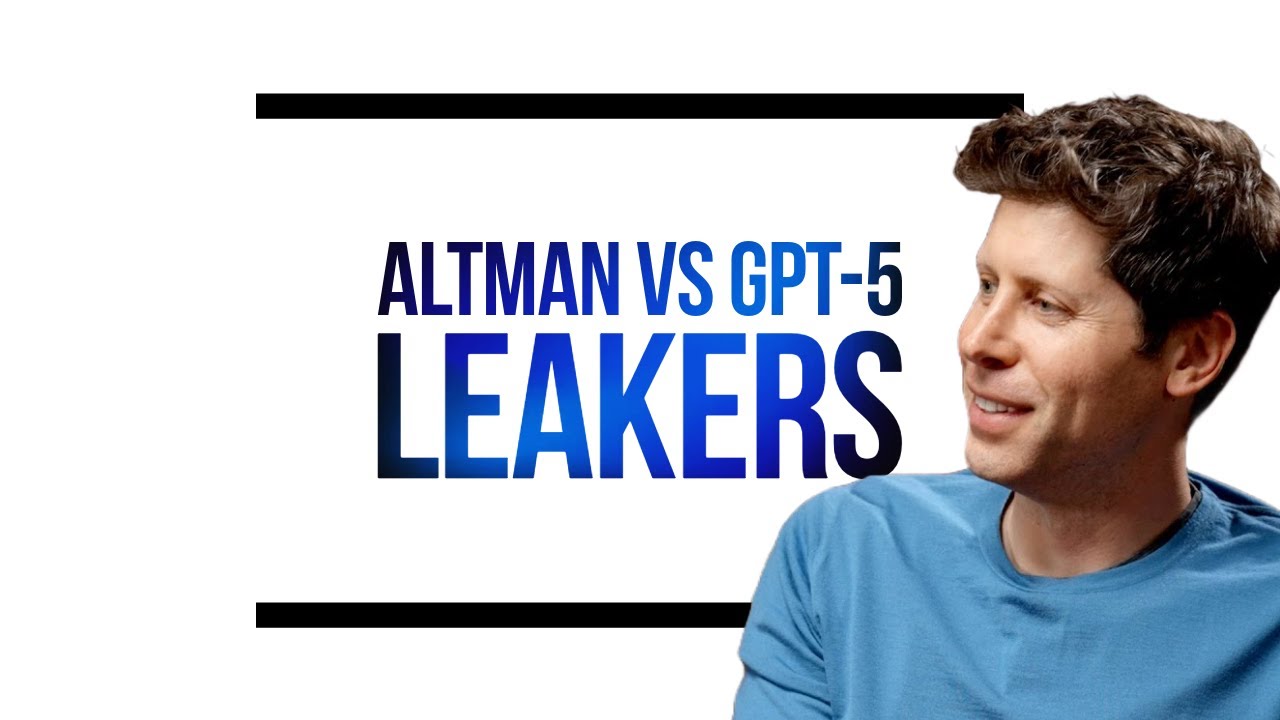
Leak: ‘GPT-5 exhibits diminishing returns’, Sam Altman: ‘lol’
5.0 / 5 (0 votes)